Improving ResNet Model Accuracy with Curriculum Learning Using Blurred Images.
HP3C '23: Proceedings of the 2023 7th International Conference on High Performance Compilation, Computing and Communications(2023)
摘要
Deep learning has achieved remarkable success in a variety of computer vision tasks, including image classification, object detection, and semantic segmentation. One of the major directions in deep learning development is to design effective training strategies that can improve model performance and generalization. In this paper, we creatively apply curriculum learning using blur images to train the famous ResNet models. We hypothesize that presenting blurry images using curriculum learning can help the model to learn general representation rather than detailed features and to descend the loss closer to the global minimum without overfitting. We verify our hypothesis by training on real-world datasets using control group, and thoroughly examine our discoveries. Our work represents an important step in curriculum learning in the latest ResNet studies, and has the potential to universally improve the performance of image classification models.
更多查看译文
AI 理解论文
溯源树
样例
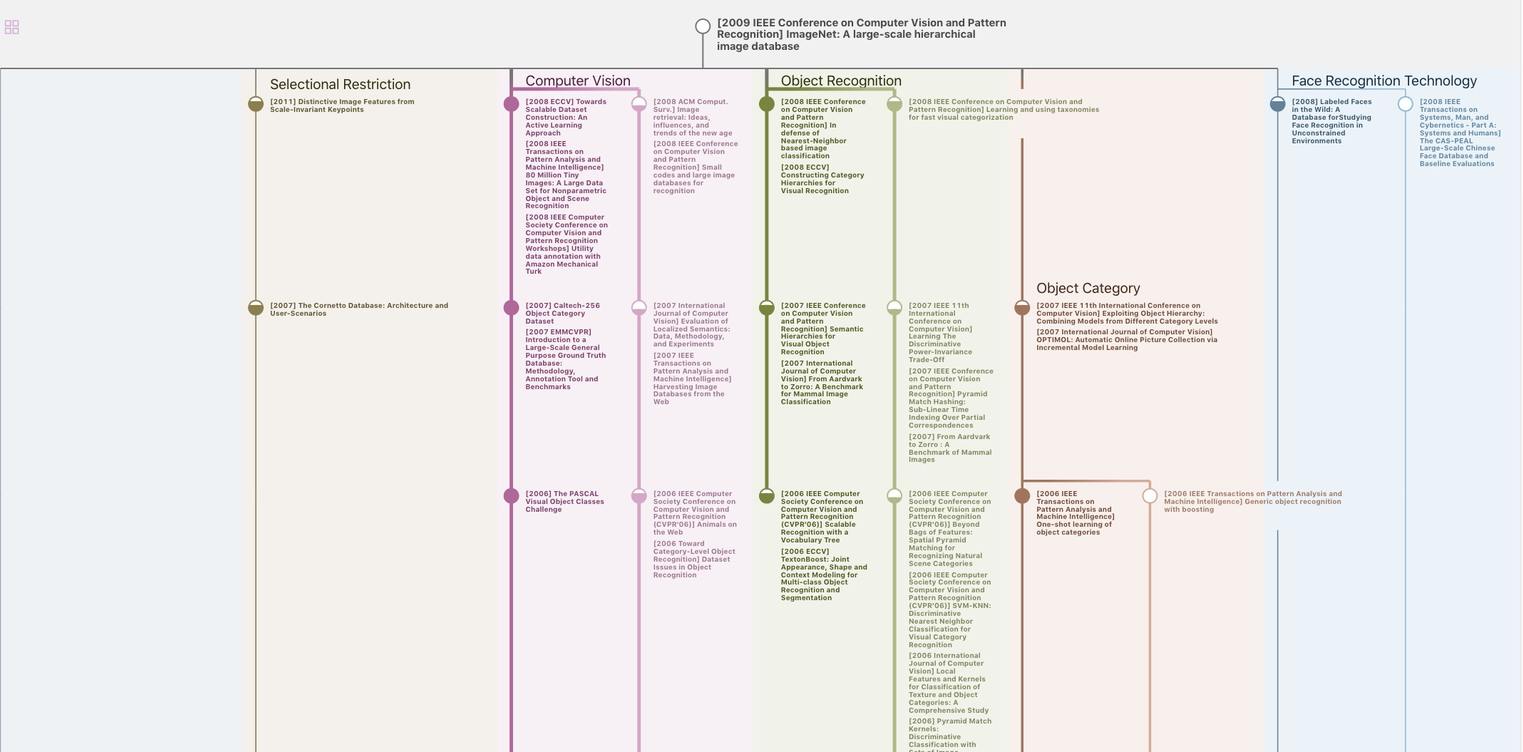
生成溯源树,研究论文发展脉络
Chat Paper
正在生成论文摘要