Diversity-Promoting Deep Reinforcement Learning for Interactive Recommendation
ICCSE '21: 5th International Conference on Crowd Science and Engineering(2022)
摘要
The interactive recommendation systems have recently attracted lots of research attentions, because they can model the interactions between the user and the recommender system. Most previous interactive recommendation methods only focus on optimizing recommendation accuracy. However, they usually overlook other important aspects of recommendation quality, e.g., the diversity of recommendation results. In this work, we propose a novel recommendation framework, called Diversity-promoting Deep Reinforcement Learning (D2RL), which aims to promote the diversity of interactive recommendation results. In D2RL, the Determinantal Point Process (DPP) is used to generate diverse, while relevant item recommendations. For each user, a personalized DPP kernel matrix is maintained, which is constructed from two parts: a fixed similarity matrix capturing item-item similarity, and the relevance of items dynamically learnt through an actor-critic reinforcement learning framework. To demonstrate the effectiveness of the proposed D2RL model, we have performed extensive offline experiments as well as simulated online experiments with real-world datasets.
更多查看译文
AI 理解论文
溯源树
样例
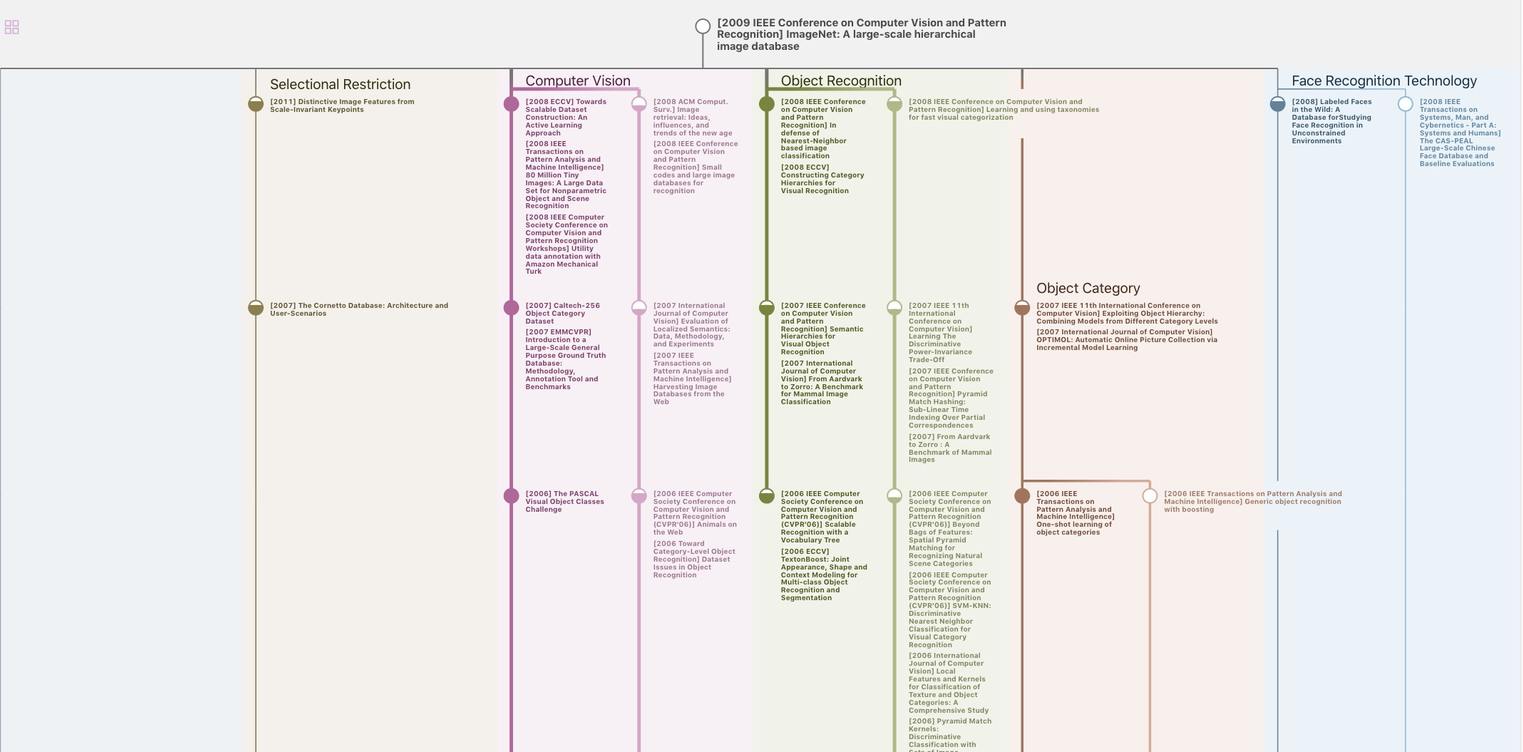
生成溯源树,研究论文发展脉络
Chat Paper
正在生成论文摘要