Data-driven Algorithms for Reducing the Carbon Footprint of Ride-sharing Ecosystems
e-Energy '23 Companion: Companion Proceedings of the 14th ACM International Conference on Future Energy Systems(2023)
摘要
Urban mobility contributes 40% of CO2 emissions from road transport, which is projected to double by 2050 [6]. Ride-sharing services like Uber and Lyft have transformed urban mobility by providing convenient and on-demand personal transportation through smartphone applications. However, their success has resulted in an increase in traffic and congestion on roads?a type of rebound effect. For example, in New York City, ride-sharing accounts for over 50% of road traffic. Recent studies estimate that a typical ride-sharing trip is less efficient than a personal car trip, mainly due to "deadhead" miles traveled by a ride-share vehicle between consecutive hired rides, resulting in 36-45% higher distance travelled and upto 47% higher CO2 emissions compared to a private car ride [3]. As a result, there is a need to develop emission-aware ride-assignment algorithms that reduce emissions from deadhead miles. Recent work has used theoretical as well as data-driven and machine learning (ML) approaches to improve the performance of ride-sharing platforms. For example, Abkarian et al. [1] present a model that aims to balance the tradeoff between waiting times and deadhead mileage driven by the vehicles in the fleet. Ke et al. [4] propose a novel spatio-temporal deep learning approach that uses a convolutional neural network (CNN) to model the spatial distribution of demand and a long short-term memory (LSTM) network to model the temporal patterns in ride demand. While these studies focus on improving the performance of ride-sharing services, they do not explicitly target reducing deadhead miles. The most relevant work to ours targets reducing deadhead miles for individual trips [5]. Authors combine demand predictions with a heuristic approach to driver assignment to demonstrate up to 82% reduction in trip-level deadhead miles. However, their approach may not effectively reduce system-wide deadhead miles and emissions, which depend on factors like fuel efficiency and traffic conditions. Furthermore, they neither consider EVs nor do they take equity into account. Our work takes a holistic approach toward designing multi-objective ride assignment optimizations, aiming to reduce emissions from deadhead miles, incorporate equity considerations, and account for EVs in ride-sharing fleets. In this paper, we present a preliminary study illustrating the benefits of emission-aware ride assignment and propose combining data-driven algorithms and machine learning to enhance online decision-making processes.
更多查看译文
AI 理解论文
溯源树
样例
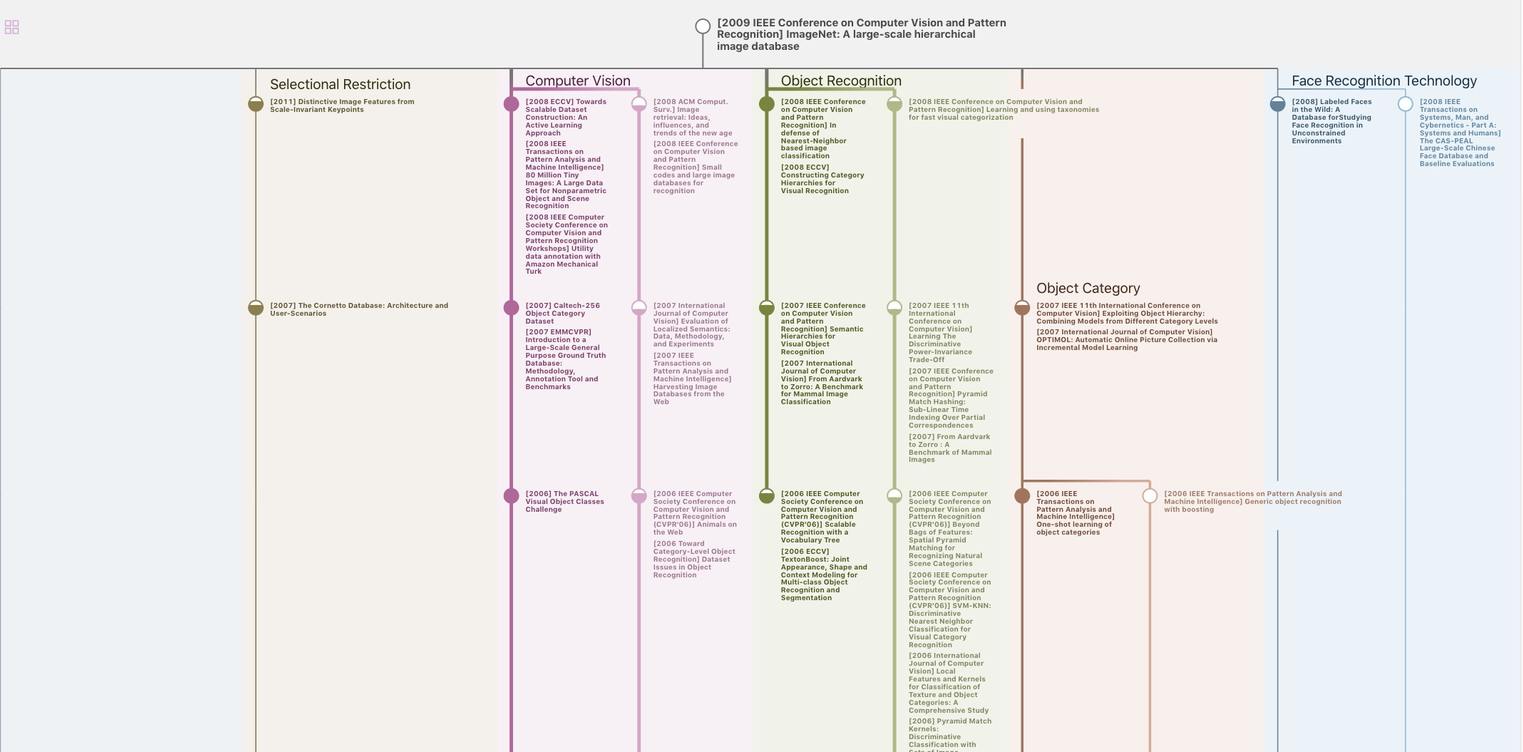
生成溯源树,研究论文发展脉络
Chat Paper
正在生成论文摘要