Hovering Control of Full-Scaled Helicopter Based on Deep Reinforcement Learning
2023 IEEE International Conference on Unmanned Systems (ICUS)(2023)
摘要
The control of helicopters, especially full-scaled helicopters, is widely regarded as a challenging problem because of its highly nonlinear dynamics. This paper describes a trial to hover the full-scaled helicopter via the deep reinforcement learning (DRL) method. Unlike previous related works, we train the controller in a model-free way. First, a nonlinear six degrees-of-freedom (DOF) dynamics model of the full-scaled UH-60 Black Hawk helicopter is established with mechanism modeling and flight experimental data. With the dynamics model, we build a numeric simulation environment for training. Then, the interactions between the controller and the environment, including the observation, the reward function, and the action space are designed. The Twin Delayed Deep Deterministic Policy Gradient (TD3) algorithm is applied to train the hovering controller, which takes around 6000 episodes to converge. Finally, the trained controller is tested by hovering experiments and further, a trajectory tracking task is carried out to validate the position control performance.
更多查看译文
关键词
helicopter control,deep reinforcement learning,model-free
AI 理解论文
溯源树
样例
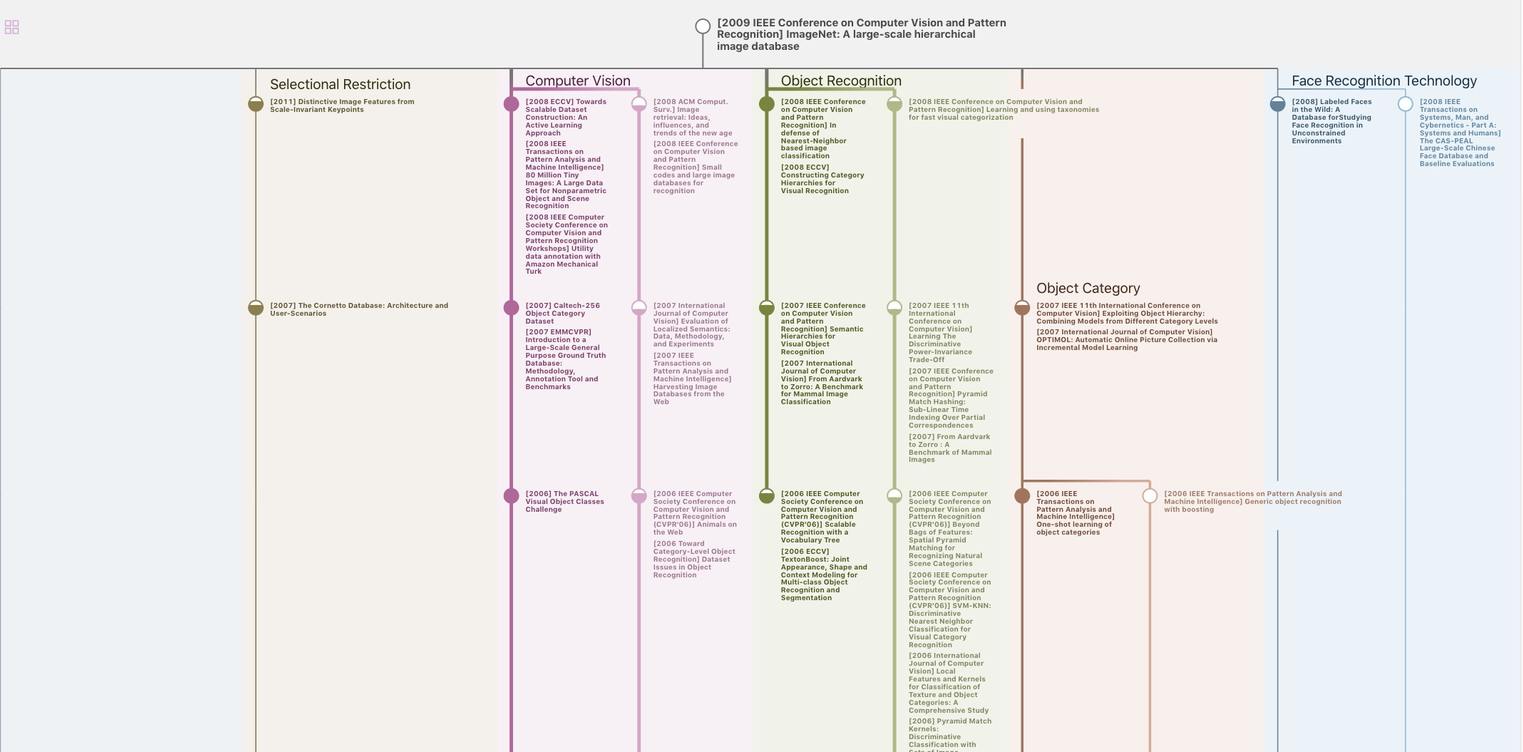
生成溯源树,研究论文发展脉络
Chat Paper
正在生成论文摘要