Backdoor Attack Against Split Neural Network-Based Vertical Federated Learning
IEEE TRANSACTIONS ON INFORMATION FORENSICS AND SECURITY(2024)
摘要
Vertical federated learning (VFL) is being used more and more widely in industry. One of its most common application scenarios is a two-party setting: a participant (i.e., the host), who exclusively owns the labels but possesses insufficient number of features, wants to improve its model performance by combining features from another participant (i.e., the client) of a different business group. The best deep ML architecture suits for this scenario is considered to be Split Neural Network (SplitNN), in which each participant runs a self-defined bottom model to learn the hidden representations (i.e., the local embeddings) of its local data and then forwards them to the host, who runs a top model to aggregate both the local embeddings to produce the final predicts. In this paper, we assume the client is malicious and demonstrate that she/he could inject a stealthy backdoor into the top model during the training to misclassify any sample to a pre-selected target class with a high probability by just replacing its local embedding with a special trigger vector regardless of the host-side embedding. This task is non-trivial because existing data poison attacks for backdoor injection in traditional models usually require to modify the labels of a set of trigger-tagged samples of non-target classes, which is impossible here as the client has no rights to access or modify the labels exclusively owned by the host. Targeting this challenge, we propose a SplitNN-dedicated data poison attack which does not require to modify any labels but just replaces the local embeddings of a very small number of target-class samples with a carefully constructed trigger vector during training. The experiments on four datasets show that our attack can achieve an attack rate as high as 94%, while bringing negligible side-effects to the model accuracy. Moreover, it is stealthy enough to resist various anomaly detection methods.
更多查看译文
关键词
Training,Data models,Toxicology,Predictive models,Neural networks,Computational modeling,Companies,Backdoor attack,split neural network,vertical federated learning
AI 理解论文
溯源树
样例
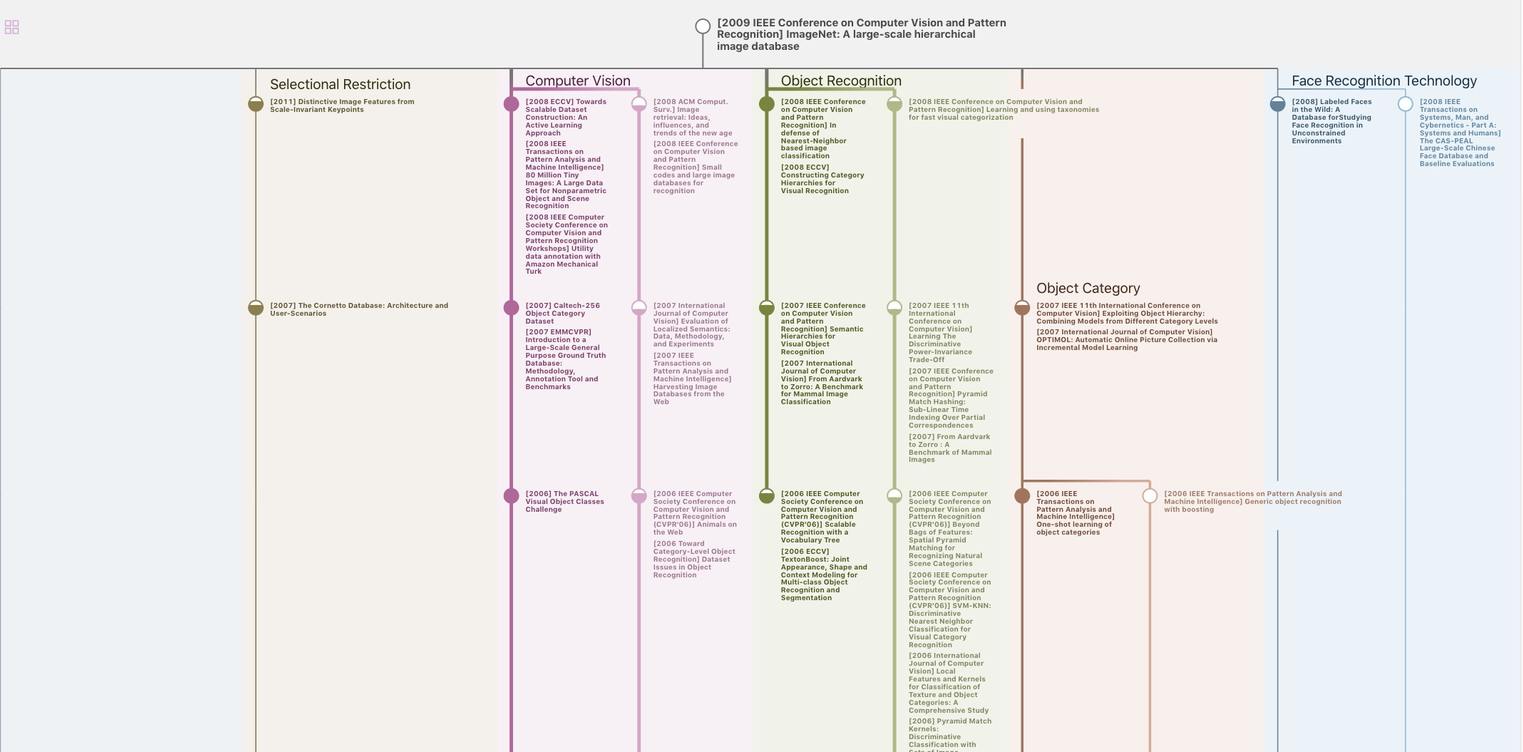
生成溯源树,研究论文发展脉络
Chat Paper
正在生成论文摘要