Computer Communications(2022)
摘要
In this paper, we design, analyze the convergence properties, address the implementation aspects, and numerically test the performance of AFAFed . This is a novel A synchronous F air A daptive Fed erated learning framework for stream-oriented IoT application environments, which are featured by time-varying operating conditions, heterogeneous resource-limited devices (i.e., coworkers), non-i.i.d. local training data and unreliable communication links. The key new of AFAFed is the synergic co-design of: (i) two sets of adaptively tuned tolerance thresholds and fairness coefficients at the coworkers and central server, respectively; and, (ii) a distributed adaptive mechanism, which allows each coworker to adaptively tune own communication rate. The convergence properties of AFAFed under (possibly) non-convex loss functions is guaranteed by a set of new analytical bounds, which formally unveil the impact on the resulting AFAFed convergence rate of a number of Federated Learning (FL) parameters, like, first and second moments of the per-coworker number of consecutive model updates, data skewness, communication packet-loss probability, and maximum/minimum values of the (adaptively tuned) mixing coefficient used for model aggregation. Extensive numerical tests show that AFAFed is capable to improve test accuracy by up to 20% and reduce training time by up to 50%, compared to state-of-the-art FL schemes, even under challenging learning scenarios featured by deep Machine Learning (ML) models, data skewness, coworker heterogeneity and unreliable communication.
更多查看译文
关键词
Adaptive asynchronous FL,Heterogeneous fog computing ecosystems,Distributed ML,Non-i.i.d. data,Personalization-vs.-generalization trade-off
AI 理解论文
溯源树
样例
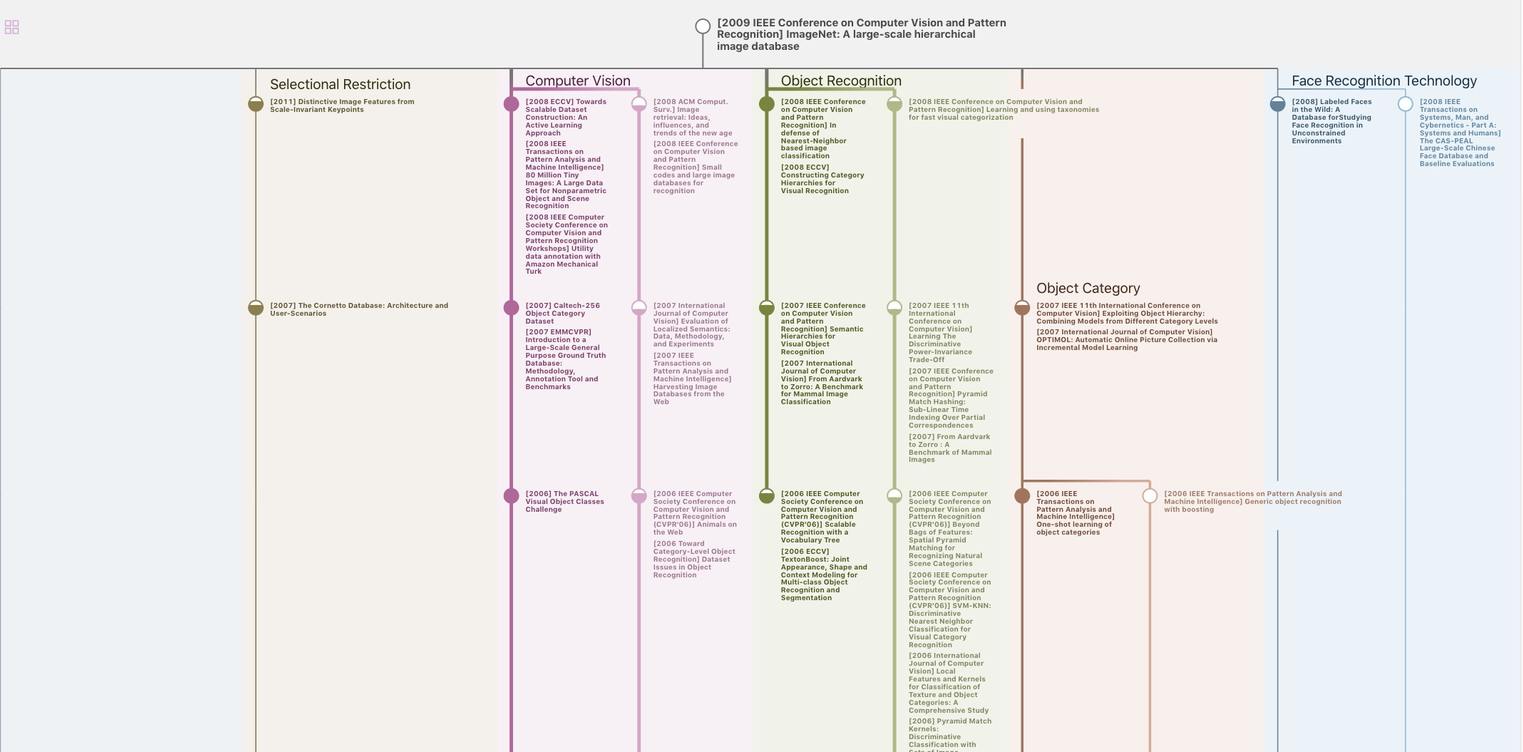
生成溯源树,研究论文发展脉络
Chat Paper
正在生成论文摘要