Variational auto encoder fused with Gaussian process for unsupervised anomaly detection
The Journal of Supercomputing(2024)
摘要
The unsupervised anomaly detection in high-dimensional and complex settings poses a formidable challenge. To tackle the challenges associated with the recognition of high-dimensional data, this paper proposes a feedback channel between the Variational Auto Encoder and the Gaussian process to enhance its data feature extraction capabilities. In order to alleviate the impact of common mislabeling issues within unsupervised contexts, the proposed model introduces a method for the selection of representative data. Subsequently, these representative data points are employed as anchors for the construction of a full-data Gaussian process model covering the entire dataset. To further disentangle the intertwined normal and abnormal data, the proposed model employs a Bayesian framework to guide the displacement of each data point within the latent space toward its position with the highest confidence. This strategic approach effectively discriminates between normal and abnormal data. This innovative method significantly enhances data consolidation within the latent space, thus preserving robustness, particularly when processing high-complexity data. Also, the numerical experiments not only validate the method’s effectiveness but also showcase the model’s robustness. These experiments highlight that even a small number of incorrect labels do not significantly impact the accuracy of anomaly detection.
更多查看译文
关键词
Machine learning,Unsupervised anomaly detection,Gaussian process,Variational auto encoder,Feature conversion
AI 理解论文
溯源树
样例
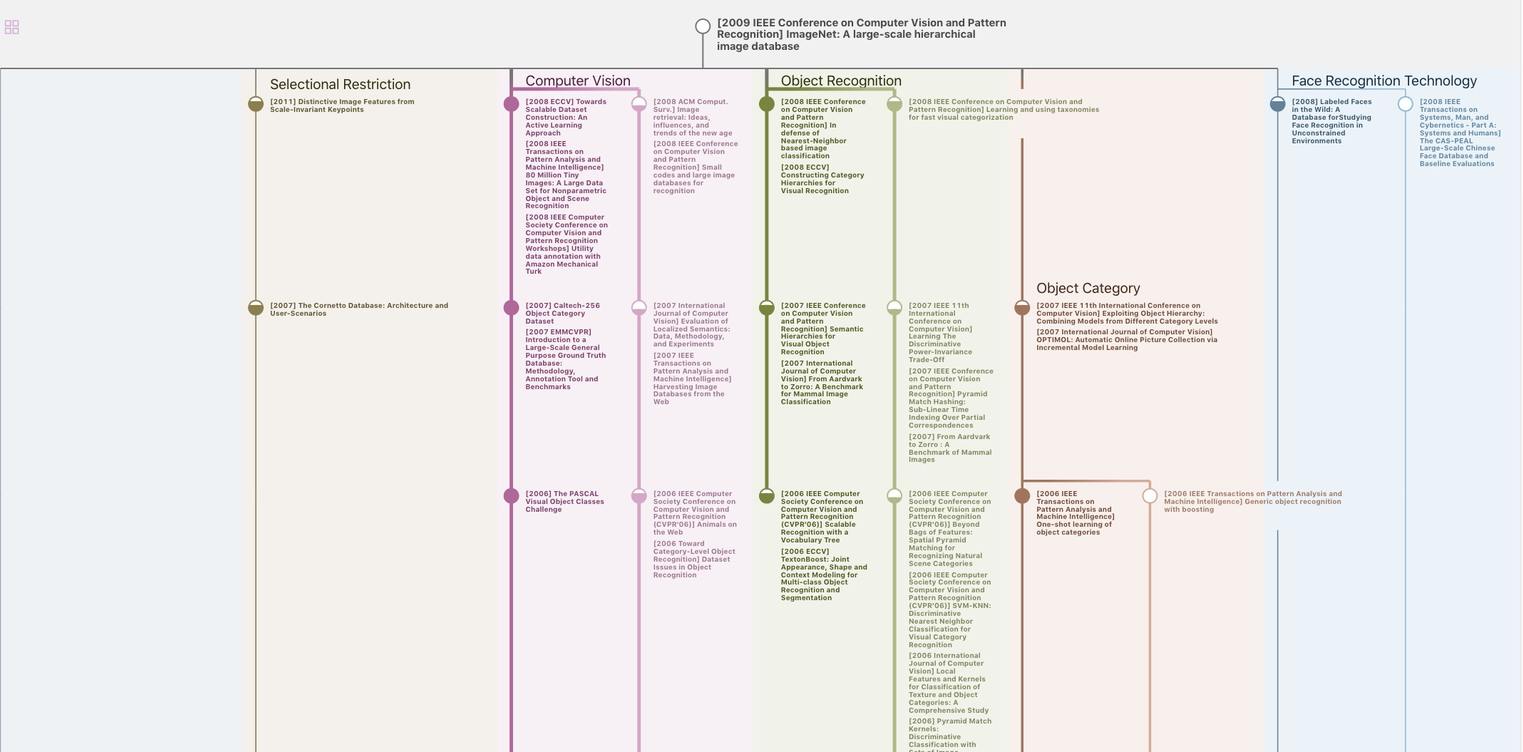
生成溯源树,研究论文发展脉络
Chat Paper
正在生成论文摘要