CurriculumLoc: Enhancing Cross-Domain Geolocalization through Multi-Stage Refinement
IEEE Transactions on Geoscience and Remote Sensing(2023)
摘要
Visual geolocalization is a cost-effective and scalable task that involves
matching one or more query images, taken at some unknown location, to a set of
geo-tagged reference images. Existing methods, devoted to semantic features
representation, evolving towards robustness to a wide variety between query and
reference, including illumination and viewpoint changes, as well as scale and
seasonal variations. However, practical visual geolocalization approaches need
to be robust in appearance changing and extreme viewpoint variation conditions,
while providing accurate global location estimates. Therefore, inspired by
curriculum design, human learn general knowledge first and then delve into
professional expertise. We first recognize semantic scene and then measure
geometric structure. Our approach, termed CurriculumLoc, involves a delicate
design of multi-stage refinement pipeline and a novel keypoint detection and
description with global semantic awareness and local geometric verification. We
rerank candidates and solve a particular cross-domain perspective-n-point (PnP)
problem based on these keypoints and corresponding descriptors, position
refinement occurs incrementally. The extensive experimental results on our
collected dataset, TerraTrack and a benchmark dataset, ALTO, demonstrate that
our approach results in the aforementioned desirable characteristics of a
practical visual geolocalization solution. Additionally, we achieve new high
recall@1 scores of 62.6% and 94.5% on ALTO, with two different distances
metrics, respectively. Dataset, code and trained models are publicly available
on https://github.com/npupilab/CurriculumLoc.
更多查看译文
关键词
Cross-domain geolocalization,visual localization,semantic attention,geometric verification,multi-stage geolocation refinement
AI 理解论文
溯源树
样例
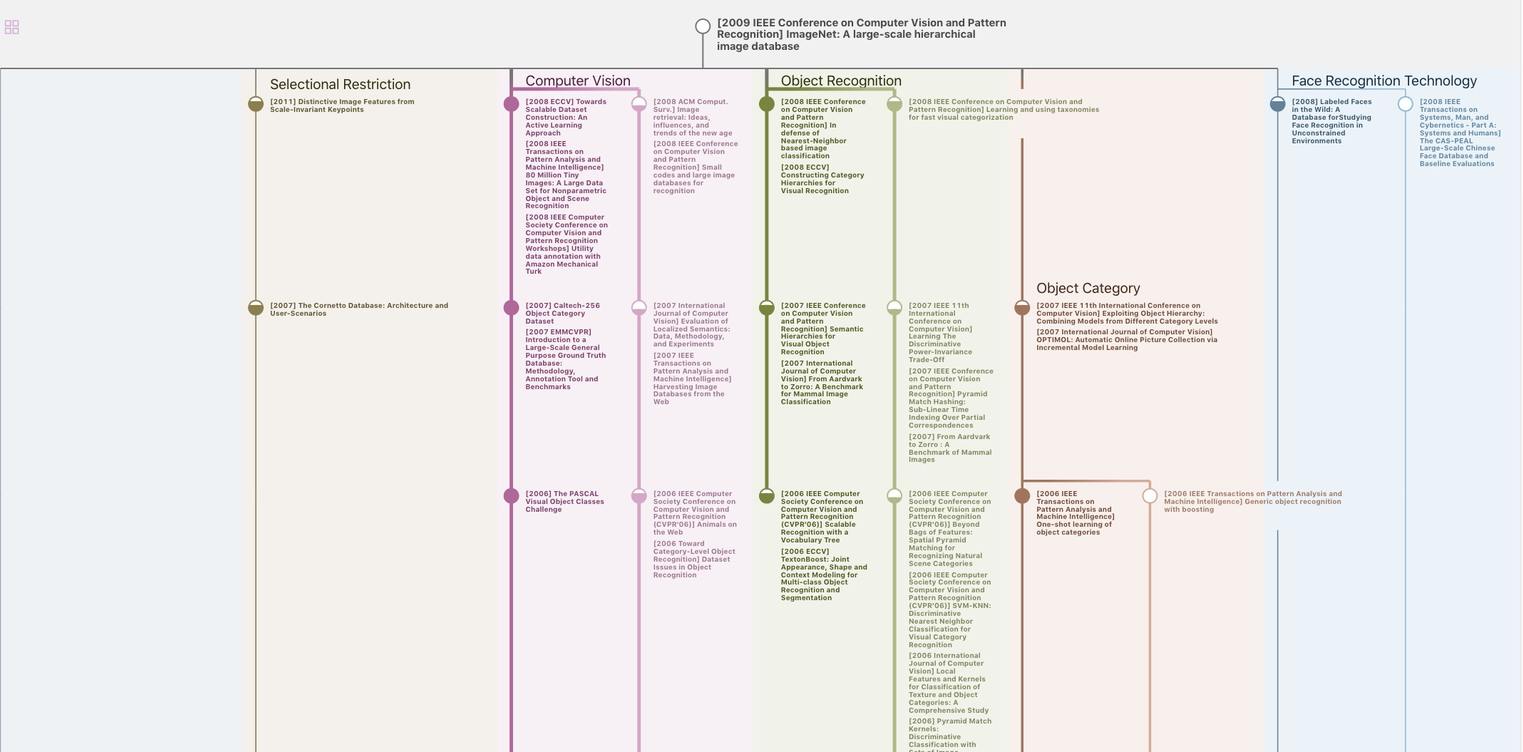
生成溯源树,研究论文发展脉络
Chat Paper
正在生成论文摘要