Machine-Learning-Based Interatomic Potentials for Group IIB to VIA Semiconductors: Towards a Universal Model
arxiv(2023)
摘要
Rapid advancements in machine-learning methods have led to the emergence of
machine-learning-based interatomic potentials as a new cutting-edge tool for
simulating large systems with ab initio accuracy. Still, the community awaits
universal inter-atomic models that can be applied to a wide range of materials
without tuning neural network parameters. We develop a unified deep-learning
inter-atomic potential (the DPA-Semi model) for 19 semiconductors ranging from
group IIB to VIA, including Si, Ge, SiC, BAs, BN, AlN, AlP, AlAs, InP, InAs,
InSb, GaN, GaP, GaAs, CdTe, InTe, CdSe, ZnS, and CdS. In addition, independent
deep potential models for each semiconductor are prepared for detailed
comparison. The training data are obtained by performing density functional
theory calculations with numerical atomic orbitals basis sets to reduce the
computational costs. We systematically compare various properties of the solid
and liquid phases of semiconductors between different machine-learning models.
We conclude that the DPA-Semi model achieves GGA exchange-correlation
functional quality accuracy and can be regarded as a pre-trained model towards
a universal model to study group IIB to VIA semiconductors.
更多查看译文
AI 理解论文
溯源树
样例
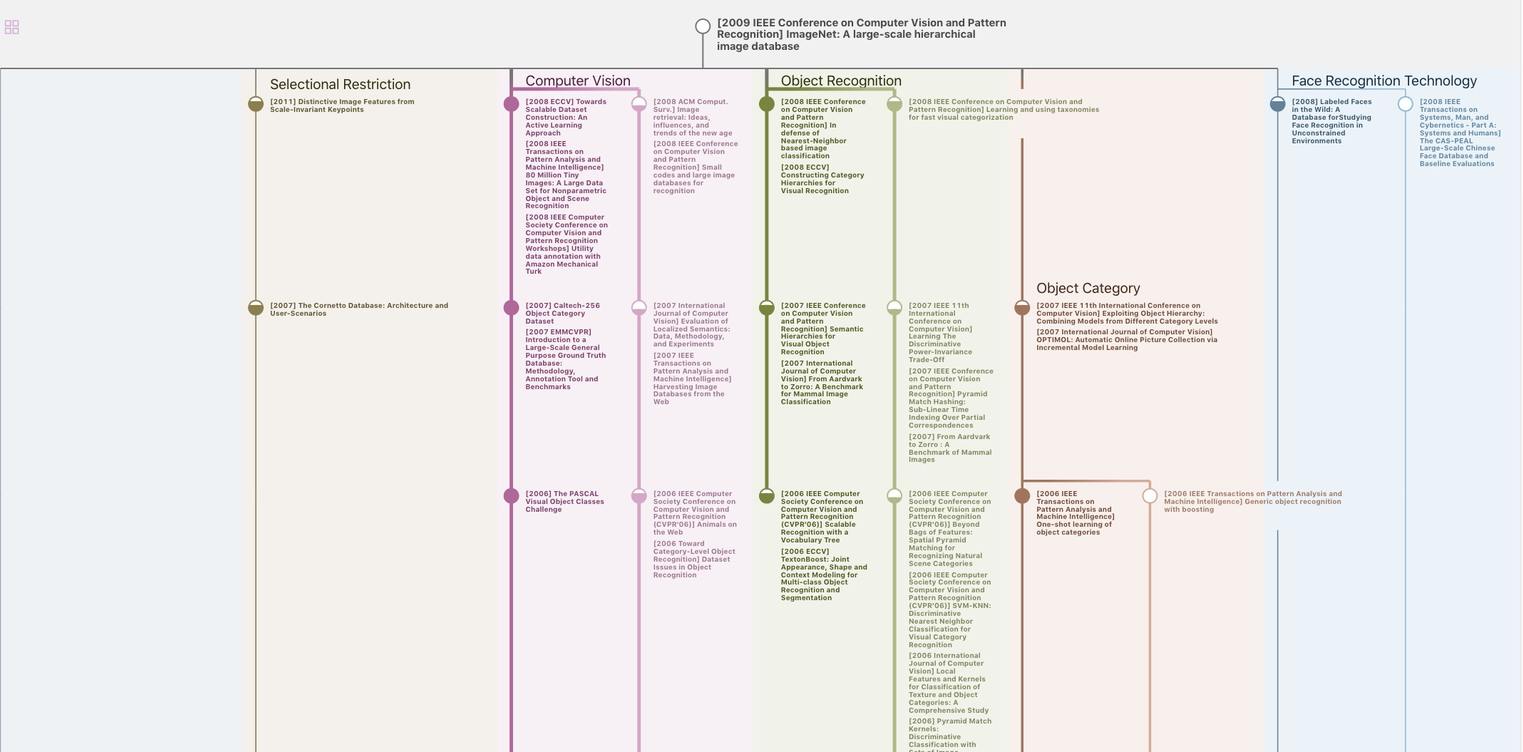
生成溯源树,研究论文发展脉络
Chat Paper
正在生成论文摘要