Leveraging Graph Machine Learning for Moonlighting Protein Prediction: A Local PPI Network and Physiochemical Feature Approach
bioRxiv (Cold Spring Harbor Laboratory)(2024)
摘要
Moonlighting proteins (MPs), characterized by their ability to perform multiple independent functions, play key roles in various metabolic pathways and disease mechanisms. Accurately predicting these proteins remains a challenge in the field of bioinformatics. Traditionally, state-of-the-art methods use the physiochemical features of proteins as predictors, employing machine learning approaches like Support Vector Machines(SVM), K-Nearest Neighbors (KNN), and Random Forests (RF). Recently, Graph Neural Networks (GNNs) surged with their capabilities in handling the graph-based data and found success in various bioinformatics applications. This study focuses on evaluating the efficacy of GNNs in predicting MPs. We introduce a specialized GNN-based framework, designed specifically for MP prediction. This framework employs Protein-Protein Interaction (PPI) networks, comprising query proteins and their interacting partners, as foundational graphs, incorporating physiochemical properties as node features. Through testing with two representative models-Graph Convolutional Networks (GCN) and Graph Attention Networks (GAT)-our approach not only excels in accuracy but also matches the performance of existing state-of-the-art methods in other metrics. The adaptability of the GNN architecture offers substantial potential for developing more advanced prediction techniques, likely increasing the accuracy of these models.
### Competing Interest Statement
The authors have declared no competing interest.
更多查看译文
AI 理解论文
溯源树
样例
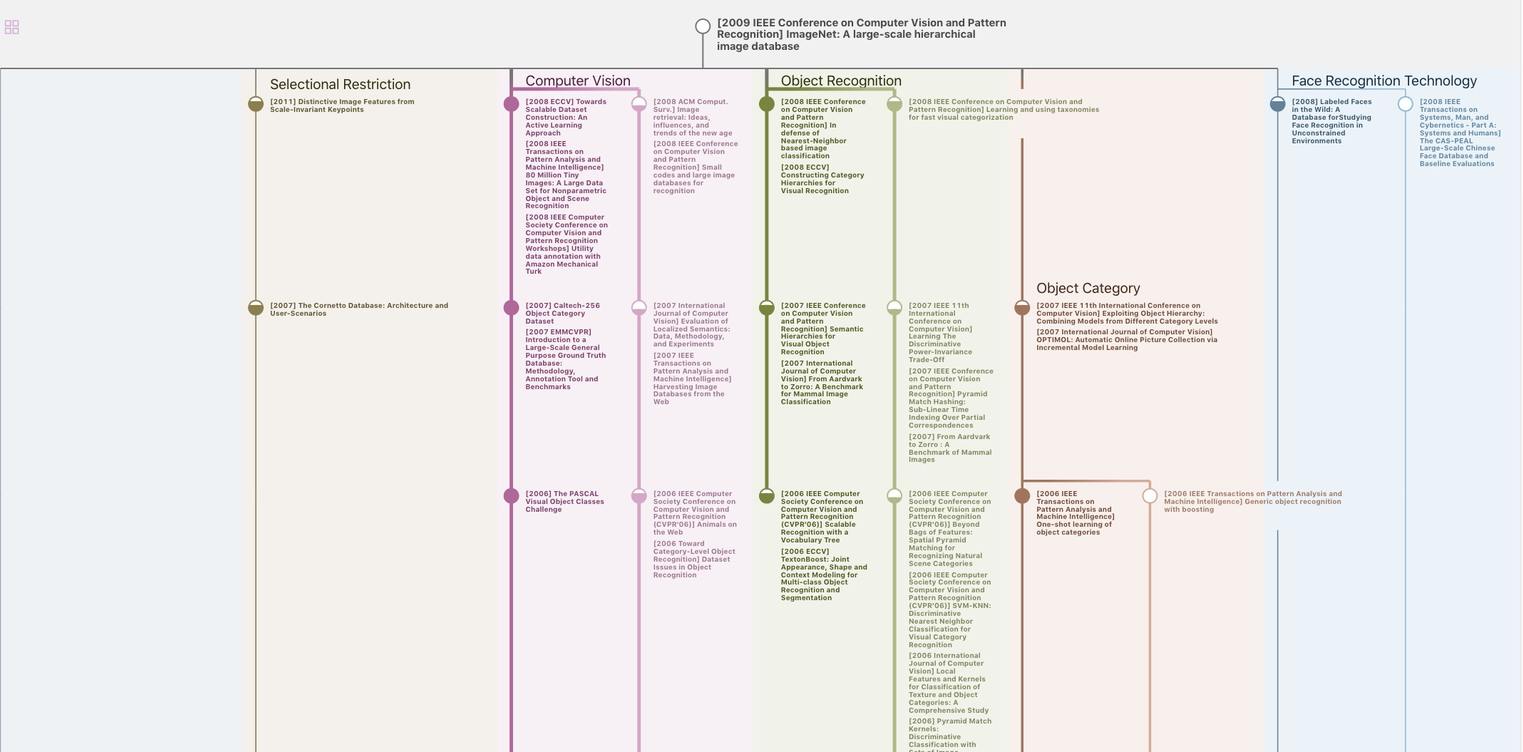
生成溯源树,研究论文发展脉络
Chat Paper
正在生成论文摘要