DIISCO: A Bayesian framework for inferring dynamic intercellular interactions from time-series single-cell data.
bioRxiv (Cold Spring Harbor Laboratory)(2023)
摘要
Characterizing cell-cell communication and tracking its variability over time is essential for understanding the coordination of biological processes mediating normal development, progression of disease, or responses to perturbations such as therapies. Existing tools lack the ability to capture time-dependent intercellular interactions, such as those influenced by therapy, and primarily rely on existing databases compiled from limited contexts. We present DIISCO, a Bayesian framework for characterizing the temporal dynamics of cellular interactions using single-cell RNA-sequencing data from multiple time points. Our method uses structured Gaussian process regression to unveil time-resolved interactions among diverse cell types according to their co-evolution and incorporates prior knowledge of receptor-ligand complexes. We show the interpretability of DIISCO in simulated data and new data collected from CAR-T cells co-cultured with lymphoma cells, demonstrating its potential to uncover dynamic cell-cell crosstalk.
更多查看译文
AI 理解论文
溯源树
样例
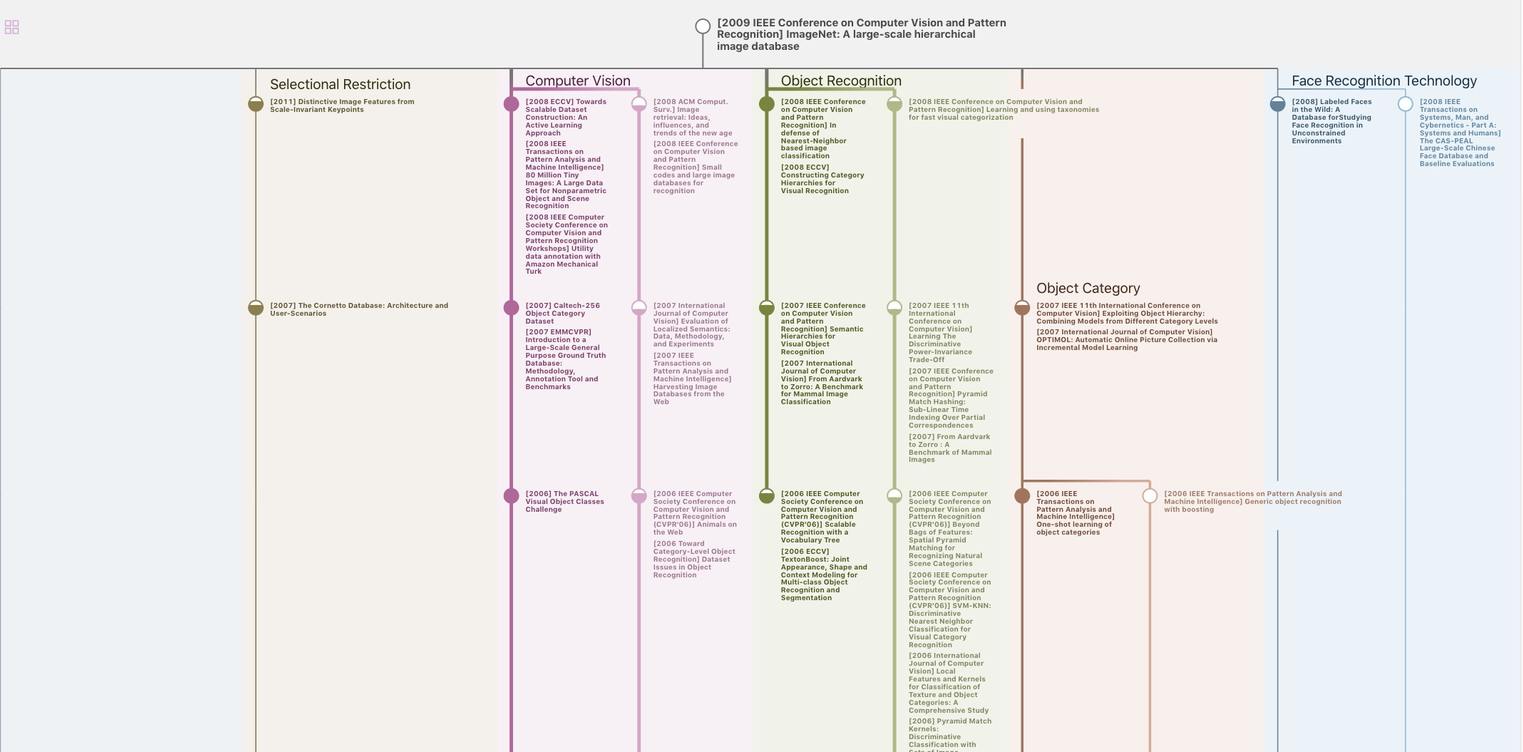
生成溯源树,研究论文发展脉络
Chat Paper
正在生成论文摘要