Improving the Generation Quality of Watermarked Large Language Models via Word Importance Scoring.
CoRR(2023)
摘要
The strong general capabilities of Large Language Models (LLMs) bring
potential ethical risks if they are unrestrictedly accessible to malicious
users. Token-level watermarking inserts watermarks in the generated texts by
altering the token probability distributions with a private random number
generator seeded by its prefix tokens. However, this watermarking algorithm
alters the logits during generation, which can lead to a downgraded text
quality if it chooses to promote tokens that are less relevant given the input.
In this work, we propose to improve the quality of texts generated by a
watermarked language model by Watermarking with Importance Scoring (WIS). At
each generation step, we estimate the importance of the token to generate, and
prevent it from being impacted by watermarking if it is important for the
semantic correctness of the output. We further propose three methods to predict
importance scoring, including a perturbation-based method and two model-based
methods. Empirical experiments show that our method can generate texts with
better quality with comparable level of detection rate.
更多查看译文
关键词
Topic Modeling,Natural Language Processing,Word Representation
AI 理解论文
溯源树
样例
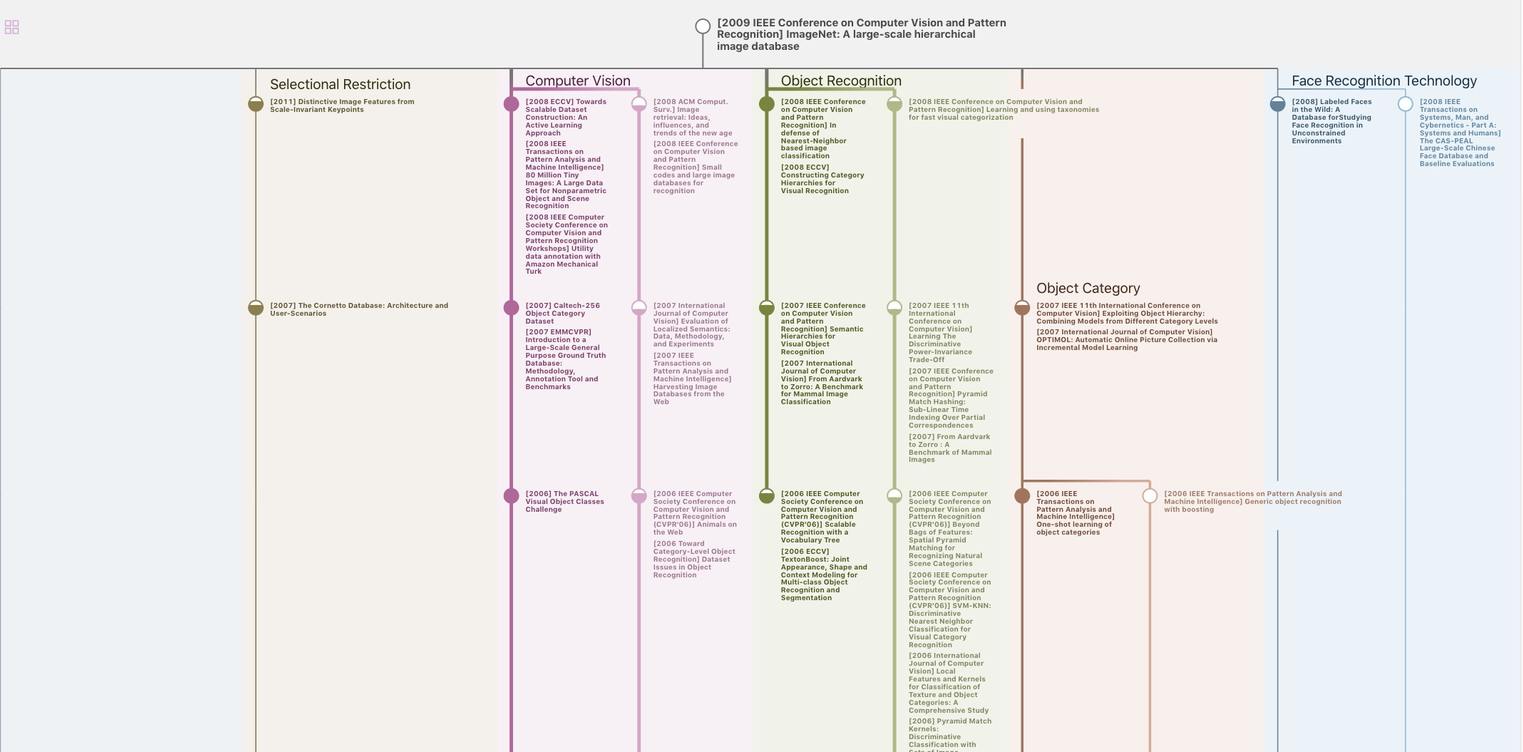
生成溯源树,研究论文发展脉络
Chat Paper
正在生成论文摘要