SCORE: A framework for Self-Contradictory Reasoning Evaluation.
CoRR(2023)
摘要
Large language models (LLMs) have demonstrated impressive reasoning ability
in various language-based tasks. Despite many proposed reasoning methods aimed
at enhancing performance in downstream tasks, two fundamental questions
persist: Does reasoning genuinely support predictions, and how reliable is the
quality of reasoning? In this paper, we propose a framework \textsc{SCORE} to
analyze how well LLMs can reason. Specifically, we focus on self-contradictory
reasoning, where reasoning does not support the prediction. We find that LLMs
often contradict themselves when performing reasoning tasks that involve
contextual information and commonsense. The model may miss evidence or use
shortcuts, thereby exhibiting self-contradictory behaviors. We also employ the
Point-of-View (POV) method, which probes models to generate reasoning from
multiple perspectives, as a diagnostic tool for further analysis. We find that
though LLMs may appear to perform well in one-perspective settings, they fail
to stabilize such behavior in multi-perspectives settings. Even for correct
predictions, the reasoning may be messy and incomplete, and LLMs can easily be
led astray from good reasoning. \textsc{SCORE}'s results underscore the lack of
robustness required for trustworthy reasoning and the urgency for further
research to establish best practices for a comprehensive evaluation of
reasoning beyond accuracy-based metrics.
更多查看译文
AI 理解论文
溯源树
样例
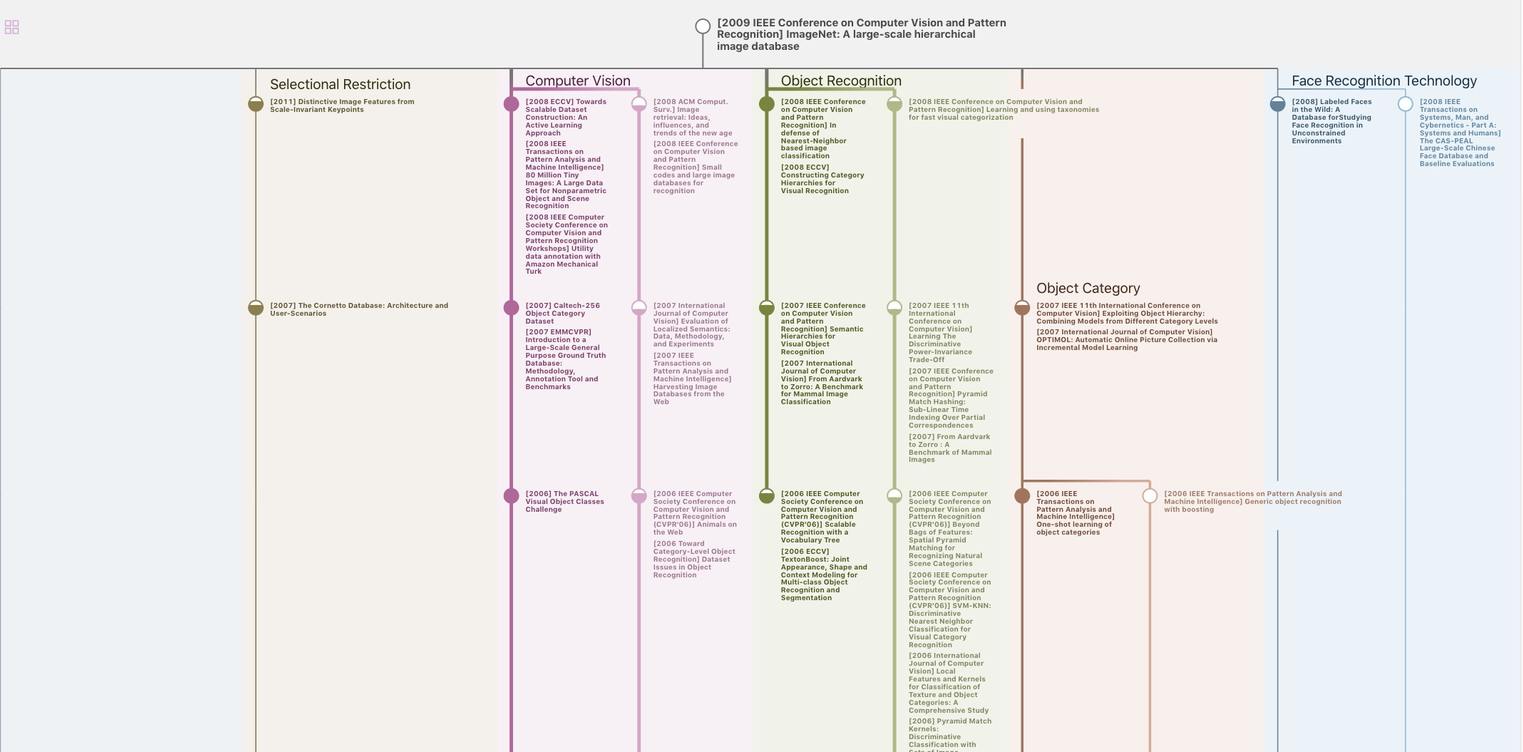
生成溯源树,研究论文发展脉络
Chat Paper
正在生成论文摘要