Human-Centric Autonomous Systems With LLMs for User Command Reasoning
arXiv (Cornell University)(2023)
摘要
The evolution of autonomous driving has made remarkable advancements in
recent years, evolving into a tangible reality. However, a human-centric
large-scale adoption hinges on meeting a variety of multifaceted requirements.
To ensure that the autonomous system meets the user's intent, it is essential
to accurately discern and interpret user commands, especially in complex or
emergency situations. To this end, we propose to leverage the reasoning
capabilities of Large Language Models (LLMs) to infer system requirements from
in-cabin users' commands. Through a series of experiments that include
different LLM models and prompt designs, we explore the few-shot multivariate
binary classification accuracy of system requirements from natural language
textual commands. We confirm the general ability of LLMs to understand and
reason about prompts but underline that their effectiveness is conditioned on
the quality of both the LLM model and the design of appropriate sequential
prompts. Code and models are public with the link
\url{https://github.com/KTH-RPL/DriveCmd_LLM}.
更多查看译文
关键词
Autonomic System,User Commands,Large Language Models,Series Of Experiments,Natural Language,Autonomous Vehicles,Language Model,Usage Intention,Advances In Recent Years,Detailed Explanation,Multimedia,Submodule,Sentiment Analysis,Human Intention,Few-shot Learning,Verbal Commands,Traffic Laws
AI 理解论文
溯源树
样例
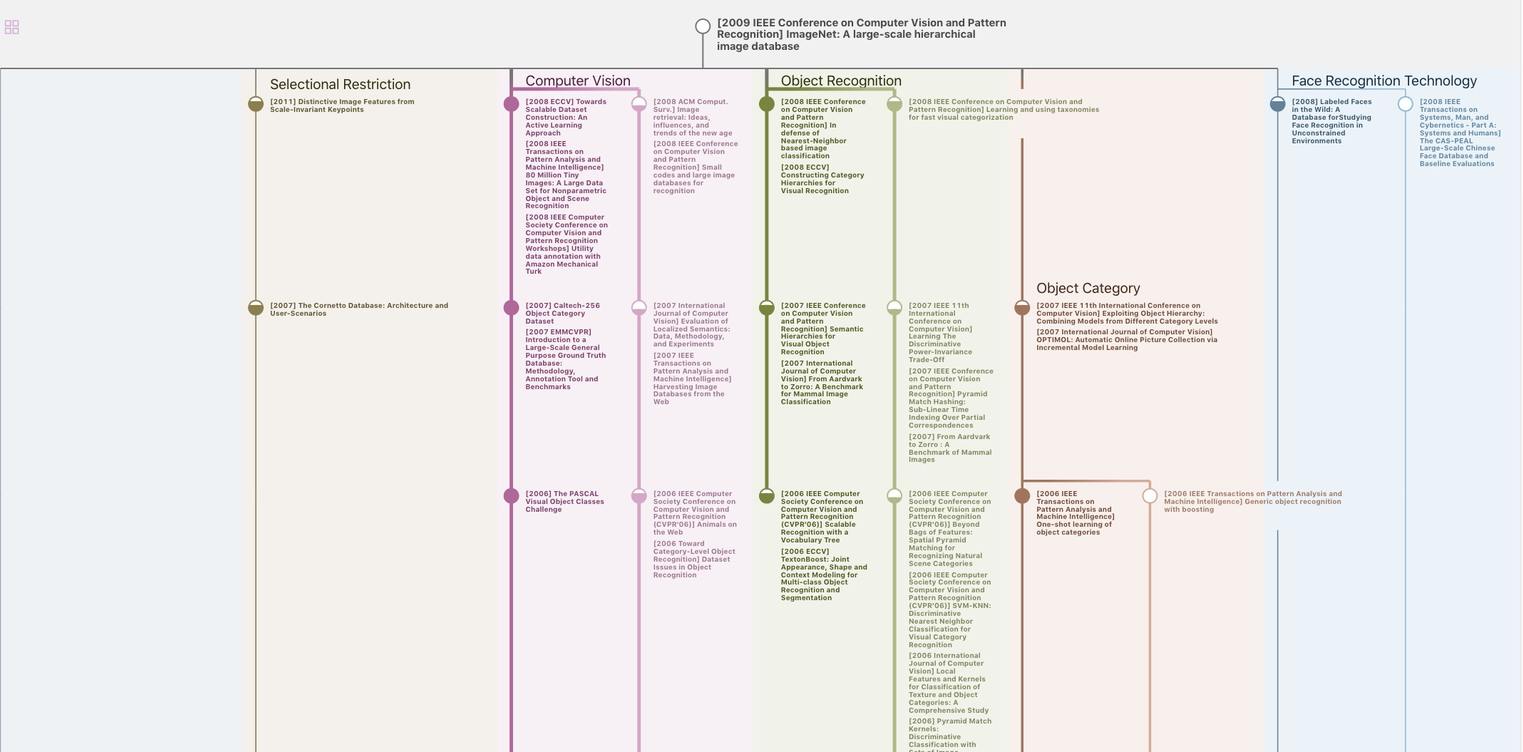
生成溯源树,研究论文发展脉络
Chat Paper
正在生成论文摘要