SpICE: An interpretable method for spatial data
arXiv (Cornell University)(2023)
摘要
Statistical learning methods are widely utilized in tackling complex problems
due to their flexibility, good predictive performance and its ability to
capture complex relationships among variables. Additionally, recently developed
automatic workflows have provided a standardized approach to implementing
statistical learning methods across various applications. However these tools
highlight a main drawbacks of statistical learning: its lack of interpretation
in their results. In the past few years an important amount of research has
been focused on methods for interpreting black box models. Having interpretable
statistical learning methods is relevant to have a deeper understanding of the
model. In problems were spatial information is relevant, combined interpretable
methods with spatial data can help to get better understanding of the problem
and interpretation of the results.
This paper is focused in the individual conditional expectation (ICE-plot), a
model agnostic methods for interpreting statistical learning models and
combined them with spatial information. ICE-plot extension is proposed where
spatial information is used as restriction to define Spatial ICE curves
(SpICE). Spatial ICE curves are estimated using real data in the context of an
economic problem concerning property valuation in Montevideo, Uruguay.
Understanding the key factors that influence property valuation is essential
for decision-making, and spatial data plays a relevant role in this regard.
更多查看译文
AI 理解论文
溯源树
样例
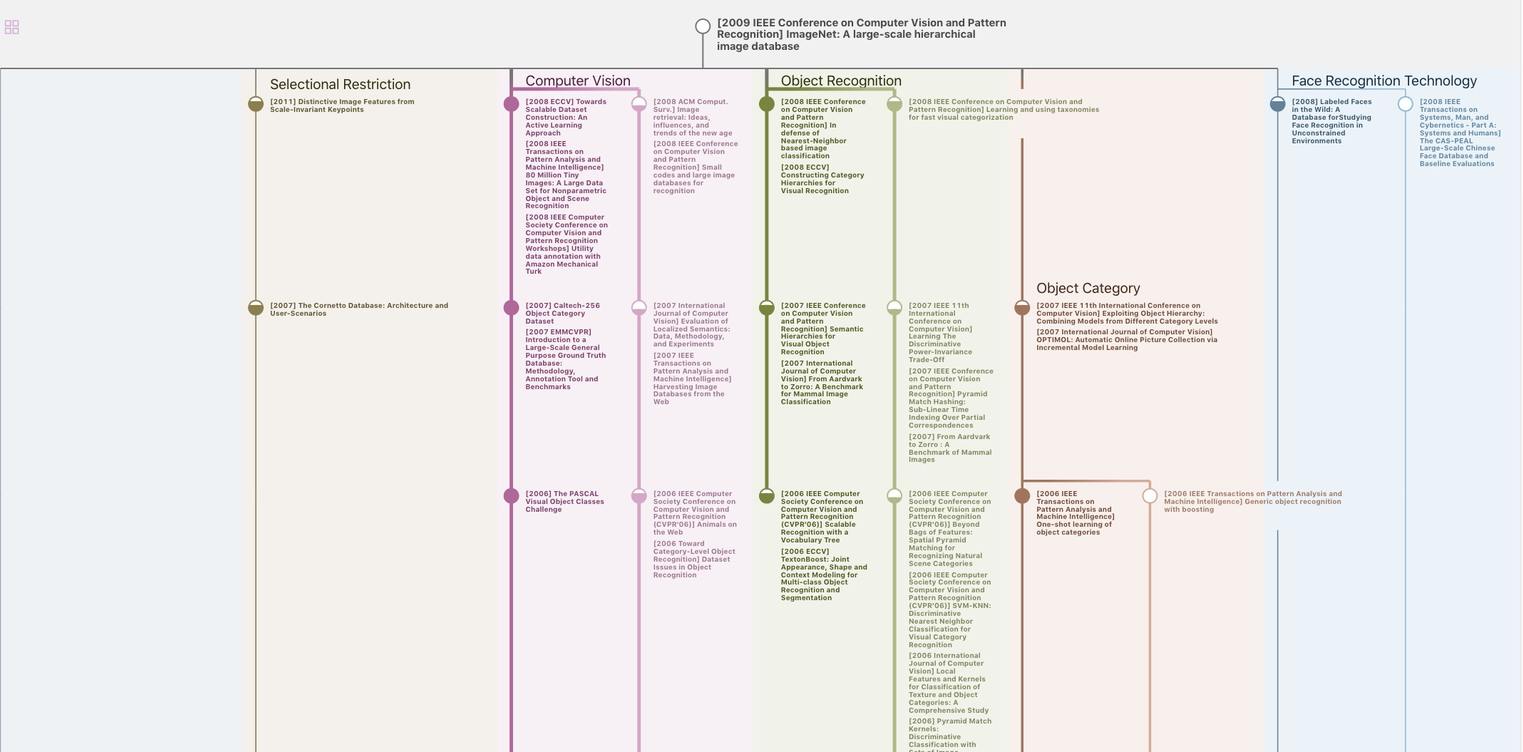
生成溯源树,研究论文发展脉络
Chat Paper
正在生成论文摘要