Step by Step to Fairness: Attributing Societal Bias in Task-oriented Dialogue Systems.
CoRR(2023)
Abstract
Recent works have shown considerable improvements in task-oriented dialogue (TOD) systems by utilizing pretrained large language models (LLMs) in an end-to-end manner. However, the biased behavior of each component in a TOD system and the error propagation issue in the end-to-end framework can lead to seriously biased TOD responses. Existing works of fairness only focus on the total bias of a system. In this paper, we propose a diagnosis method to attribute bias to each component of a TOD system. With the proposed attribution method, we can gain a deeper understanding of the sources of bias. Additionally, researchers can mitigate biased model behavior at a more granular level. We conduct experiments to attribute the TOD system's bias toward three demographic axes: gender, age, and race. Experimental results show that the bias of a TOD system usually comes from the response generation model.
MoreTranslated text
Key words
Spoken Dialogue Systems,Topic Modeling,Argumentation Frameworks,Dialectical Argumentation,Dialog Management
AI Read Science
Must-Reading Tree
Example
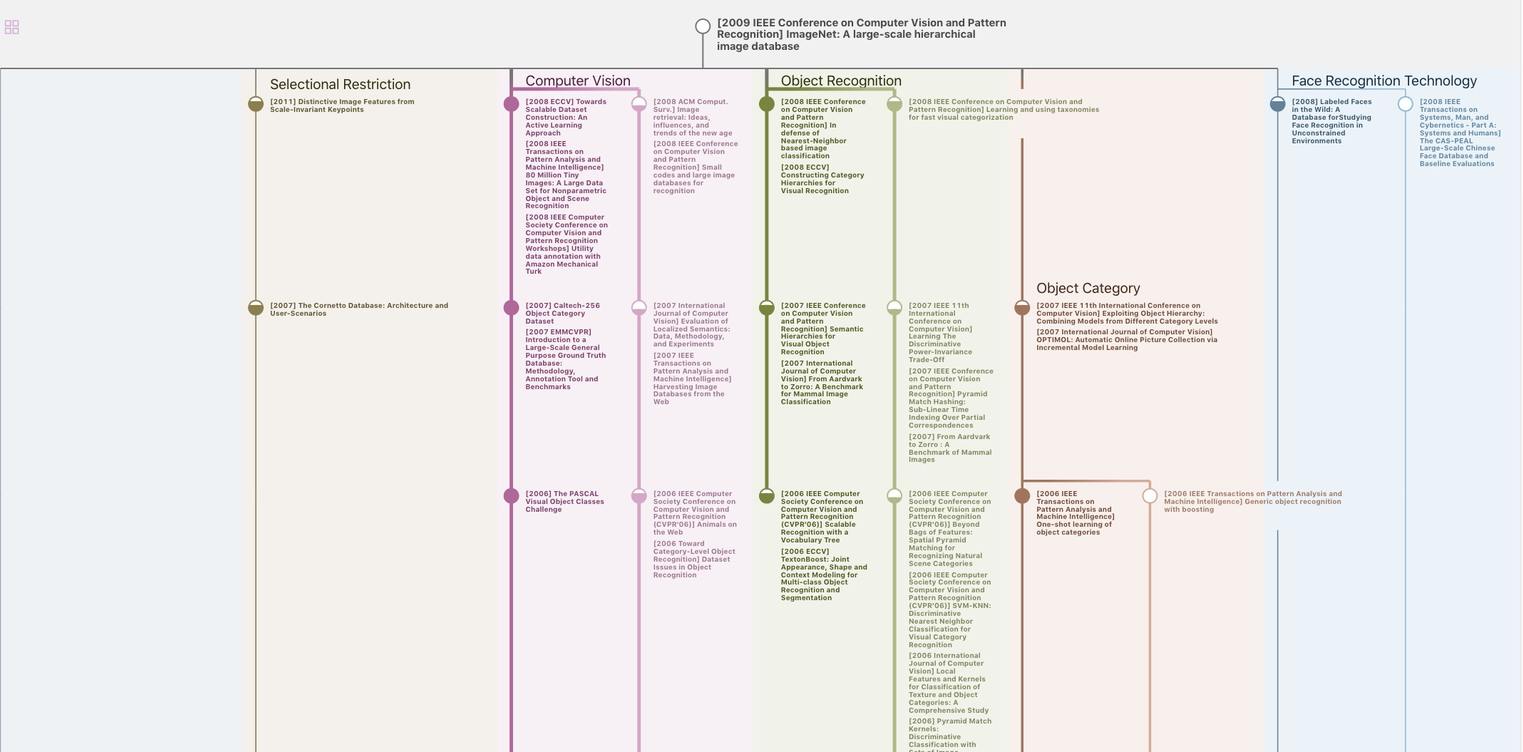
Generate MRT to find the research sequence of this paper
Chat Paper
Summary is being generated by the instructions you defined