Greedy PIG: Adaptive Integrated Gradients.
CoRR(2023)
摘要
Deep learning has become the standard approach for most machine learning tasks. While its impact is undeniable, interpreting the predictions of deep learning models from a human perspective remains a challenge. In contrast to model training, model interpretability is harder to quantify and pose as an explicit optimization problem. Inspired by the AUC softmax information curve (AUC SIC) metric for evaluating feature attribution methods, we propose a unified discrete optimization framework for feature attribution and feature selection based on subset selection. This leads to a natural adaptive generalization of the path integrated gradients (PIG) method for feature attribution, which we call Greedy PIG. We demonstrate the success of Greedy PIG on a wide variety of tasks, including image feature attribution, graph compression/explanation, and post-hoc feature selection on tabular data. Our results show that introducing adaptivity is a powerful and versatile method for making attribution methods more powerful.
更多查看译文
关键词
pig,integrated
AI 理解论文
溯源树
样例
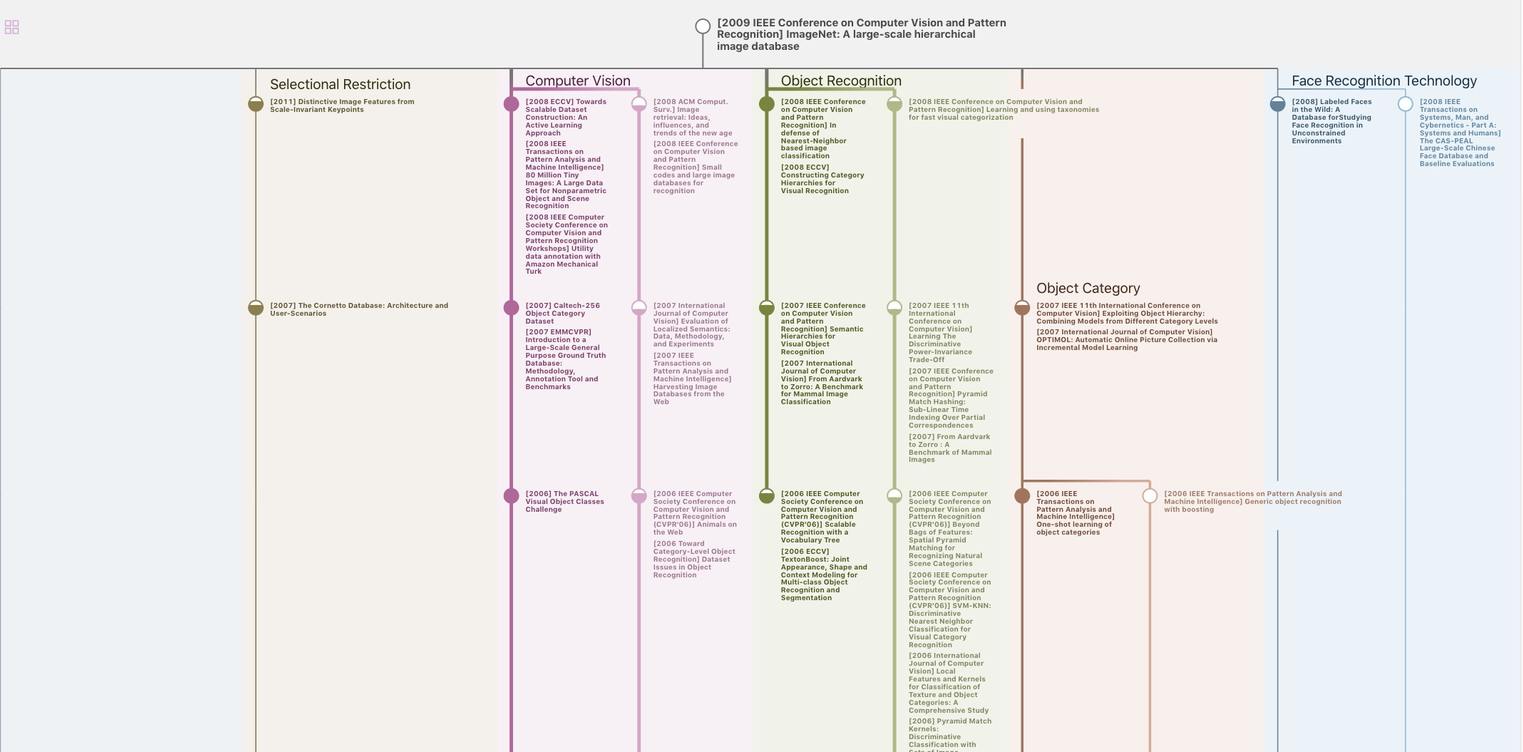
生成溯源树,研究论文发展脉络
Chat Paper
正在生成论文摘要