A nonsmooth optimization method.
CoRR(2023)
摘要
We present NCCSG, a nonsmooth optimization method. In each iteration, NCCSG finds the best length-constrained descent direction by considering the worst bound over all local subgradients. NCCSG can take advantage of local smoothness or local strong convexity of the objective function. We prove a few global convergence rates of NCCSG. For well-behaved nonsmooth functions (characterized by the weak smooth property), NCCSG converges in $O(\frac{1}{\epsilon} \log \frac{1}{\epsilon})$ iterations, where $\epsilon$ is the desired optimality gap. For smooth functions and strongly-convex smooth functions, NCCSG achieves the lower bound of convergence rates of blackbox first-order methods, i.e., $O(\frac{1}{\epsilon})$ for smooth functions and $O(\log \frac{1}{\epsilon})$ for strongly-convex smooth functions. The efficiency of NCCSG depends on the efficiency of solving a minimax optimization problem involving the subdifferential of the objective function in each iteration.
更多查看译文
关键词
nonsmooth optimization method,optimization method
AI 理解论文
溯源树
样例
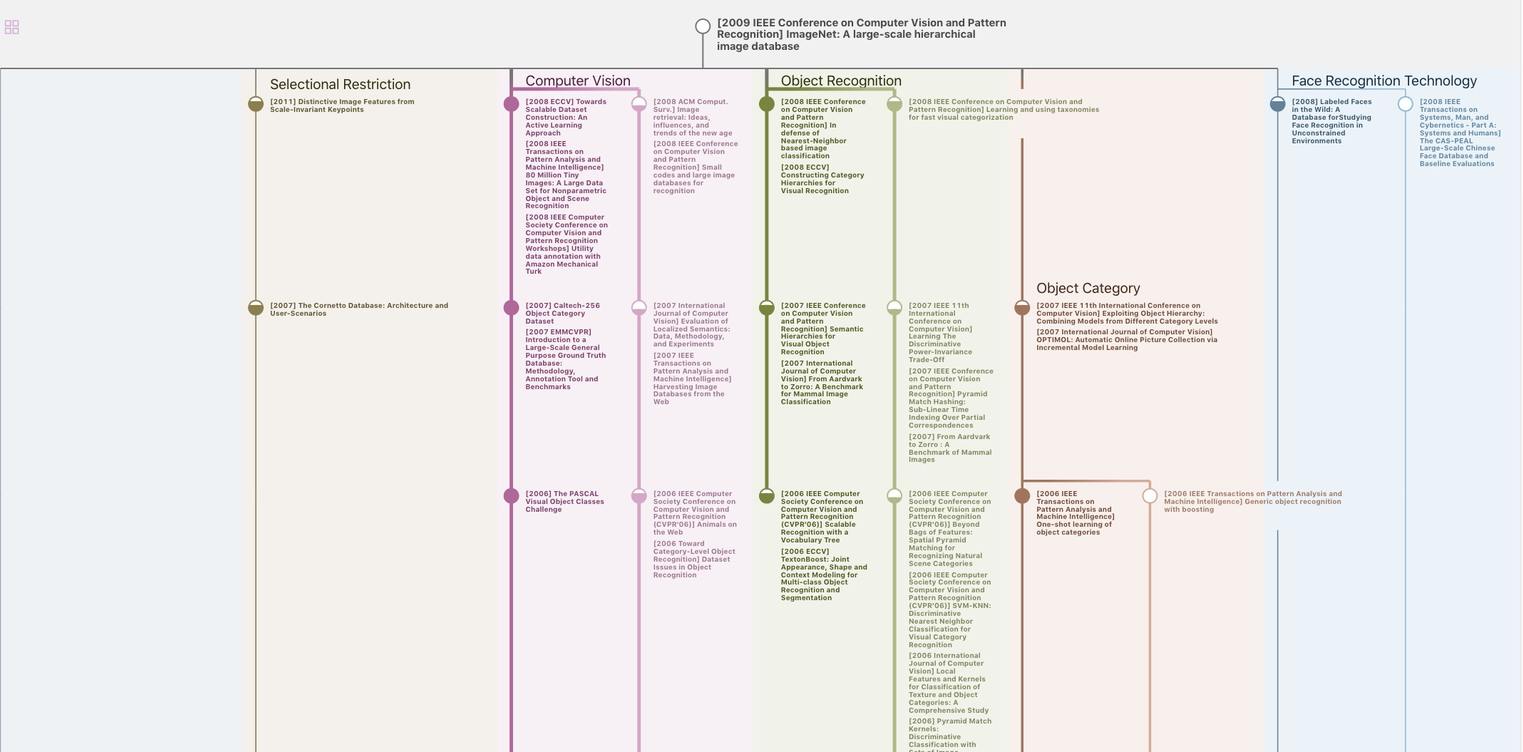
生成溯源树,研究论文发展脉络
Chat Paper
正在生成论文摘要