A Low-Cost Microcontroller-Based Normal and Abnormal Conditions Classification Model for Induction Motors Using Self-Organizing Feature Maps (SOFM)
Energies(2023)
摘要
Digital twins have provided valuable information for making effective decisions to ensure high efficiency in the manufacturing process using virtual models. Consequently, AC electric motors play a pivotal role in this framework, commonly employed as the primary electric actuators within Industry 4.0. In addition, classification systems could be implemented to identify normal and abnormal operating conditions in electric machines. Moreover, the execution of such classification systems in low-cost digital embedded systems is crucial, enabling continuous monitoring of AC electric machines. Self-Organized Maps (SOMs) offer a promising solution for implementing classification systems in low-cost embedded systems due to their ability to reduce system dimensionality and visually represent the model's features, so local digital systems can be used as classification systems. Therefore, this paper aims to investigate the utilization of SOMs for classifying operating conditions in AC electric machines. Furthermore, when integrated into an embedded system, SOMs detect abnormal conditions in AC electric machines. A trained SOM is deployed on a C2000 microcontroller to exemplify the proposed approach. It should be noted that the proposed structure can be adapted for implementation with different systems in the context of Industry 4.0.
更多查看译文
关键词
classification systems, Self-Organized Maps, virtual model, electric machines, microcontroller
AI 理解论文
溯源树
样例
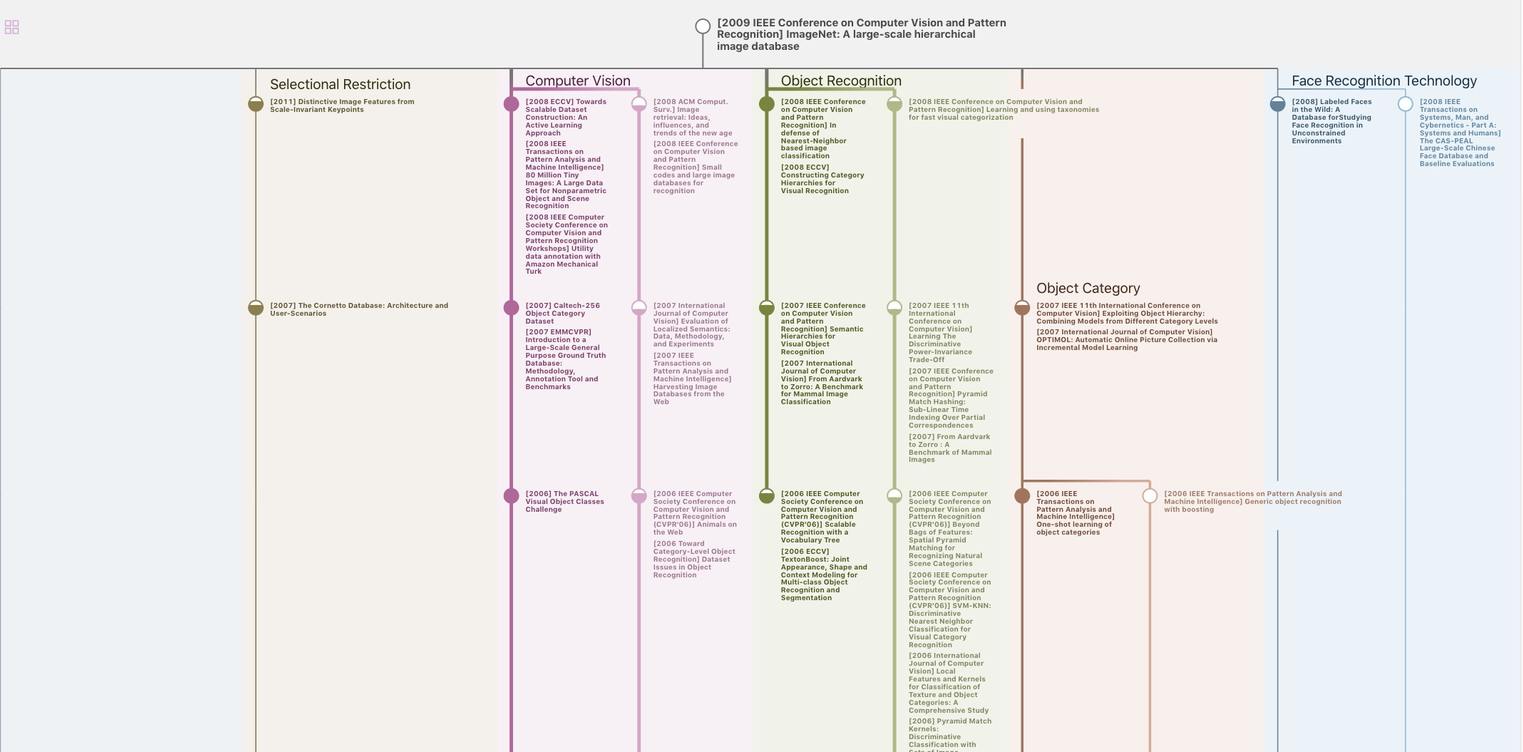
生成溯源树,研究论文发展脉络
Chat Paper
正在生成论文摘要