Deep learning and heterogeneous signal fusion approach to precursor feature recognition and early warning of coal and gas outburst
PROCESS SAFETY AND ENVIRONMENTAL PROTECTION(2023)
摘要
Coal and gas outburst is one of the main disasters during the production process of coal mines. Accurate recognition and advanced early warning are crucial to effectively preventing and controlling outburst. Acoustic Emission (AE) or Electromagnetic Resonance (EMR) continuous monitoring technologies have been widely used in outburst prediction due to their advantages of positive response and significant precursor characteristics of impending hazards. However, using a single signal and its one-dimensional time-domain characteristic for predicting outburst may lead to false and omissions alarms due to low credibility and lack of potential information, which may reduce the reliability and advance of early warning. To solve this problem, a new method for precursory feature recognition and early warning of outburst based on a two-dimensional CNN (Convolutional Neural Network) and heterogeneous signal fusion is proposed. Establish an outburst precursor feature recognition model based on Faster R-CNN and AE-EMR two-dimensional time-frequency signals, providing more reliable evidence sources for the fusion early warning of outburst; Then construct an outburst early warning model based on TBM (Transferable Belief Model), revealing the early warning criteria of heterogeneous signal fusion. The proposed method is applied to the Shiping Coal Mine. It is found that the outburst precursor recognition accuracy of AE and EMR achieve 98.00% and 98.57%; The heterogeneous signal fusion model usually warns to be advanced by 1–2 days, and the minimum warning time can be about 60 min in advance. The research results have practical significance in improving the reliability and advancement of outburst early warning and enhancing the ability to control safety risks in the coal mine production process.
更多查看译文
关键词
Coal and gas outburst,Faster R-CNN,Precursor feature recognition,Heterogeneous signal fusion,Early warning
AI 理解论文
溯源树
样例
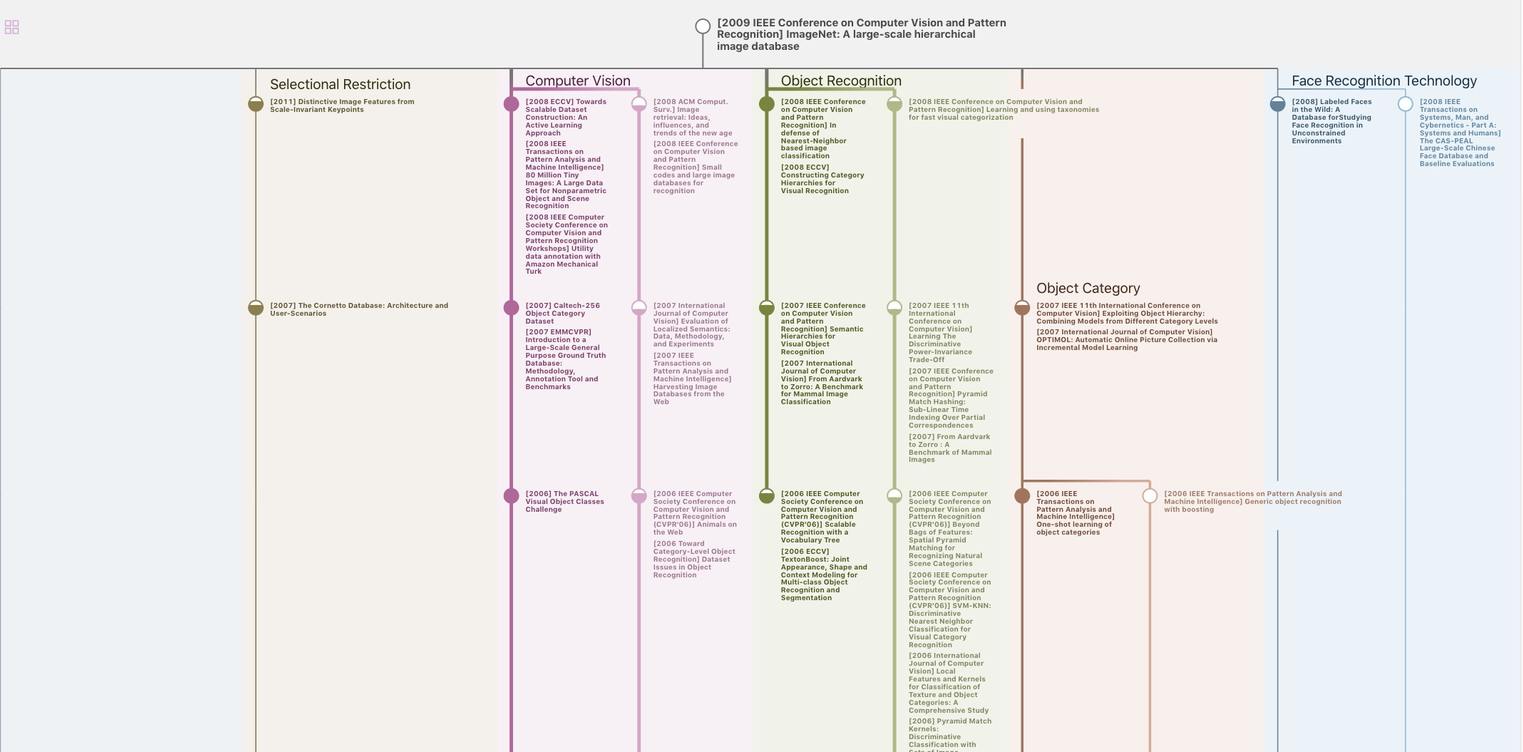
生成溯源树,研究论文发展脉络
Chat Paper
正在生成论文摘要