An Ambiguous Edge Detection Method for Computed Tomography Scans of Coronavirus Disease 2019 Cases
IEEE TRANSACTIONS ON SYSTEMS MAN CYBERNETICS-SYSTEMS(2024)
摘要
Recently, the coronavirus disease of 2019 (COVID-19), as named by the World Health Organization (WHO), has spread to over 200 countries. The WHO has declared this disease as a worldwide public health emergency. One of the most difficult tasks in combating this epidemic is to identify and segregate the afflicted people. The reverse transcription-polymerase chain reaction test (RT-PCR) is the most common pathology test used to diagnose this infection. Studies show that the RT-PCR test has a low-positive rate and sometimes becomes ineffective in diagnosing infection. In some cases, computed tomography (CT) scans reveal acute pneumonia and pulmonary anomalies. Therefore, CT scans are used together with RT-PCR tests to confirm infected people. Existing artificial intelligence and machine learning techniques require a large number of CT scans for training, which is a time-consuming process. Visual inspection shows that most CT scans of COVID-19 cases have broken, blurred, and ambiguous edges for infectious areas. Another major issue with these images is the heterogeneous intensity of the pixels, high noise, and low resolution. As a result of all these issues, the problem of effective edges/boundaries of various areas of CT scans of COVID-19 cases cannot be resolved by the current edge detection approach. Indeed, improper selection of edges can lead to an incorrect diagnosis of diseases through CT scans of COVID-19 cases. Therefore, there is an urgent need for a diagnostic method in addition to the RT-PCR test that can extract useful information from the minimum number of chest CT scans of suspected COVID-19 cases. This study introduces a new ambiguous edge detection method (AEDM) for identifying the edges/boundaries of different regions in CT scans of COVID-19 cases. The proposed AEDM is developed on the basis of ambiguous set (AS) theory, which is highly efficient in processing ambiguous pixel information. For simulation purposes, various CT scans of COVID-19 cases are classified into three different categories: 1) low infection (LI); 2) moderate infection (MI); and 3) severe infection (SI). Empirical analysis shows that the proposed AEDM can effectively highlight the edges in CT scans of three different categories in comparison with other well-known edge detection methods.
更多查看译文
关键词
Ambiguous set (AS),computed tomography (CT),COVID-19,edge detection
AI 理解论文
溯源树
样例
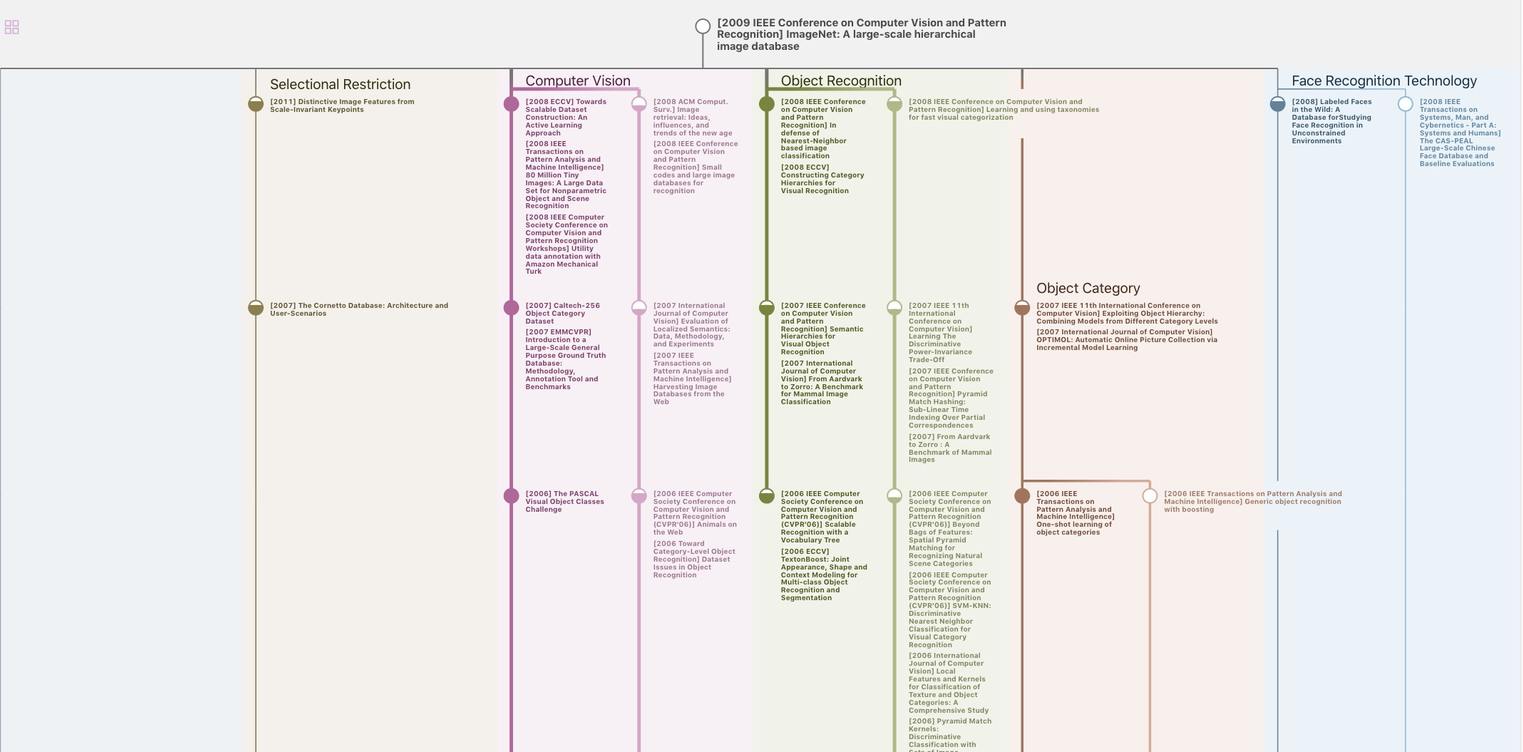
生成溯源树,研究论文发展脉络
Chat Paper
正在生成论文摘要