An intelligent milling chatter detection method based on VMD-synchro-squeeze wavelet and transfer learning via deep CNN with vibration signals
INTERNATIONAL JOURNAL OF ADVANCED MANUFACTURING TECHNOLOGY(2023)
摘要
Chatter is an undesirable vibration that reduces the surface quality and dimensional accuracy of the workpieces, as well as reduction tool life. In the rapid milling process, this phenomenon can cause a reduction in the surface quality of the workpiece that is difficult to detect and control. It means early identification of chatter is needed to improve the surface quality of the workpiece, but this is still an obstacle for manufacturers. Intelligent real-time chatter monitoring is becoming a major trend in the present manufacturing industry, which is increasingly adopting digital technology and artificial intelligence to improve the quality, speed, efficiency, and safety of production processes. Therefore, this study aimed to develop an intelligent chatter detection model for real-time monitoring based on an optimized variational mode decomposition (VMD) by using a Bayesian optimization algorithm (VMD-BOA), wavelet-based synchro-squeeze transform (WSST), and transfer learning (TL) applications of modified pre-trained deep convolution neural networks (DCNNs). Through the VMD-BOA process, a measured vibration signal is automatically decomposed into appropriate intrinsic mode components (IMFs). To select IMFs with chatter-rich information, the maximum energy ratio is calculated, and the IMFs with chatter-rich information are reconstructed for the next signal-to-image conversion stage. The reconstructed one-dimensional IMF signal is segmented, and then, a wavelet-based synchro-squeeze transform (WSST) is performed to generate vibration signal feature indicators. TL through a modified Squeeze-Net pre-trained network for generating automatic feature extraction and state recognition of machining condition processes is carried out. The results show that a high-performance model was obtained with a maximum classification accuracy of 98.21% and a good recognition time of ±4.2 s for detecting the chatter occurrence. Therefore, this new method could be a solution to the challenge of realizing real-time chatter monitoring for the manufacturing industry, especially in machining.
更多查看译文
关键词
Chatter detection,Modified pre-trained deep convolution neural networks,Wavelet synchro-squeezing transform,Transfer learning,Variational mode decomposition-Bayesian optimization algorithm
AI 理解论文
溯源树
样例
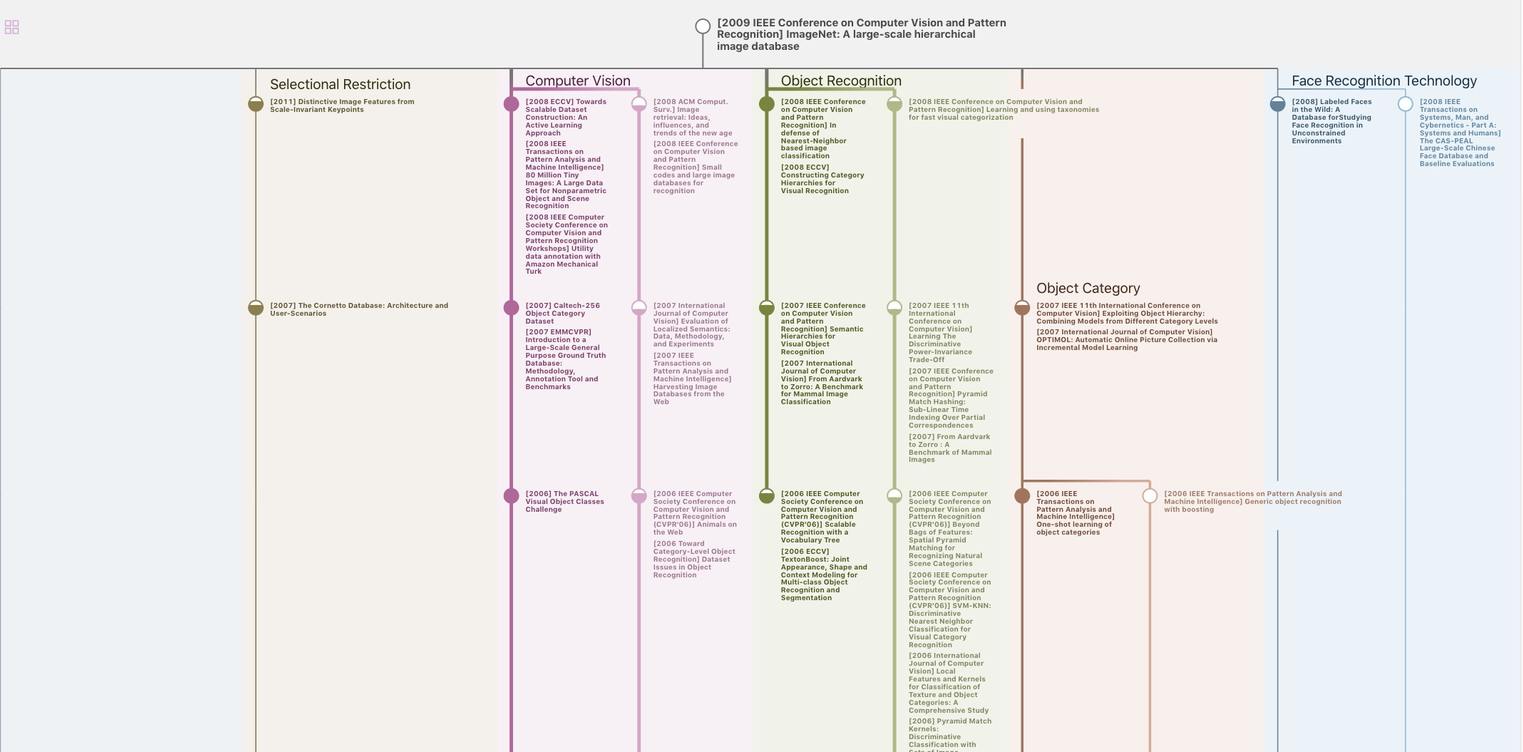
生成溯源树,研究论文发展脉络
Chat Paper
正在生成论文摘要