Differential treatment for time and frequency dimensions in mel-spectrograms: An efficient 3D Spectrogram network for underwater acoustic target classification
OCEAN ENGINEERING(2023)
摘要
Underwater acoustic target classification (UATC) has traditionally relied on time–frequency (TF) analysis, with the mel-spectrogram being widely used due to its 2D image-like format and size efficiency. However, many previous UATC approaches have not adequately acknowledged the distinction between natural images and mel-spectrograms. In this paper, we propose a novel approach to transform mel-spectrograms into 3D data and introduce an efficient 3D Spectrogram Network (3DSNet) that treats the time and frequency dimensions separately. Our 3DSNet consists of three key components: the Time–Frequency Separate Convolution (TFSConv) module, Asymmetric Pooling (AsyPool) module, and Channel-Time Attention (CTA) module. We introduce the TFSConv module, which utilizes two convolution operators for the time and frequency dimensions to extract 3D T-F features. This module serves as a lightweight approximation of 3D convolution, effectively reducing approximately 85 percent of parameters. To maintain the inherent distinction between the frequency and time dimensions, we propose an AsyPool module that employs two downsampling strategies. Additionally, we introduce a CTA module to capture more informative and meaningful T-F features. We evaluate our 3DSNet on two publicly available underwater acoustic datasets, and the results demonstrate that our method achieves the optimal balance between performance and model parameters compared to other mainstream methods.
更多查看译文
关键词
Underwater acoustic target classification,Mel-spectrograms,Light-weight network,Deep learning
AI 理解论文
溯源树
样例
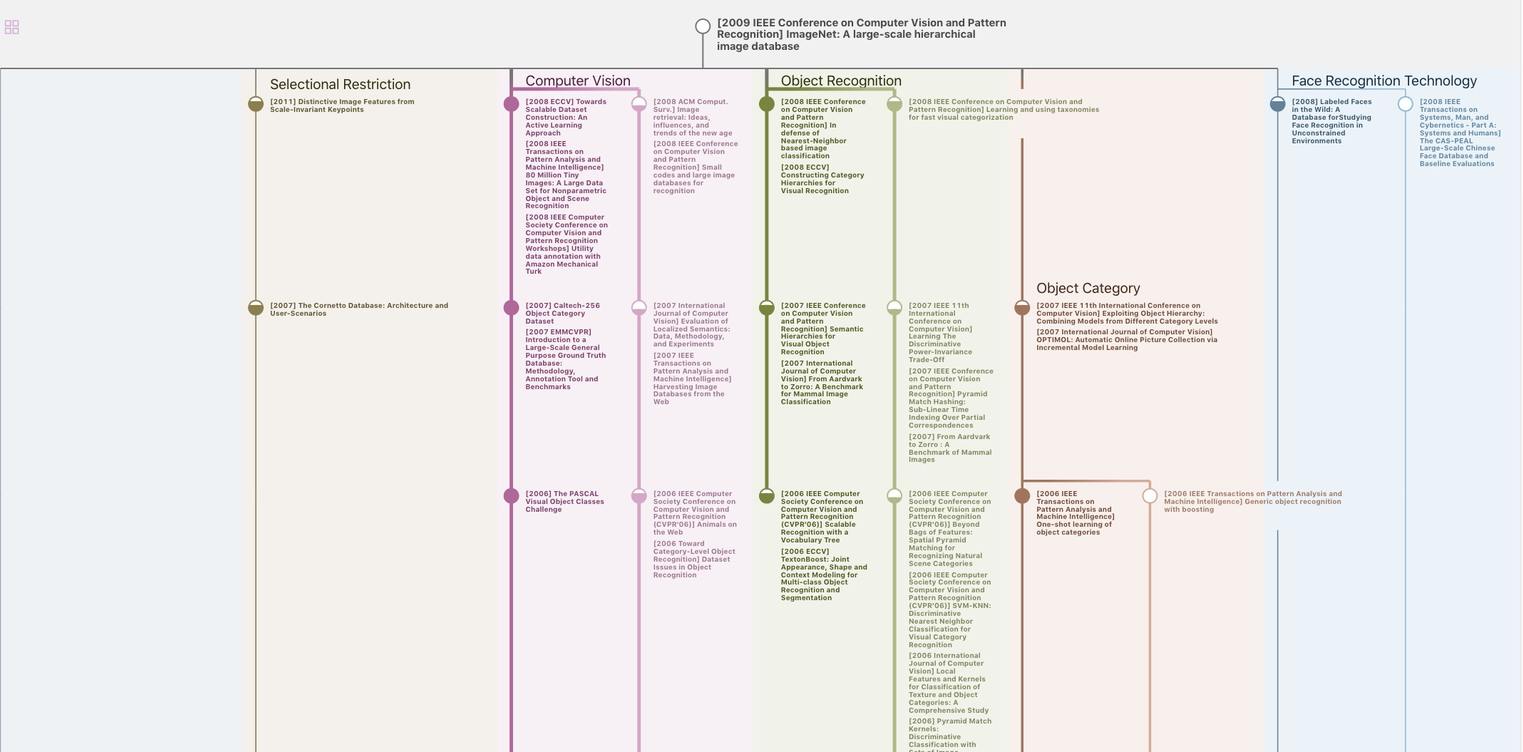
生成溯源树,研究论文发展脉络
Chat Paper
正在生成论文摘要