An accurate denoising lithium-ion battery remaining useful life prediction model based on CNN and LSTM with self-attention
IONICS(2023)
摘要
In order to ensure the safe and reliable operation of lithium-ion battery (LIB), it is urgent to accurately predict the remaining useful life (RUL) of LIB. The LIB RUL is related to many health characteristics, and the prediction accuracy of the data-driven method of extracting partial characteristics is insufficient. To solve this problem, a novel denoising LIB RUL prediction model based on convolution neural network (CNN) and long short-term memory (LSTM) with self-attention, namely, DCLA, is proposed in this article. In this model, a specially designed denoising autoencoder (DAE) is used to remove a variety of common noises in LIB data, CNN is used to mine the correlation of multiple features of LIB, and LSTM with self-attention is used to capture the time sequence information of long battery degradation sequence. A series of complementary experiments are designed to verify the effectiveness of the proposed method. The verification results show that compared with other typical data-driven methods, this method has higher prediction accuracy and robustness on datasets affected by various noises.
更多查看译文
关键词
lstm,useful life prediction model,cnn,lithium-ion,self-attention
AI 理解论文
溯源树
样例
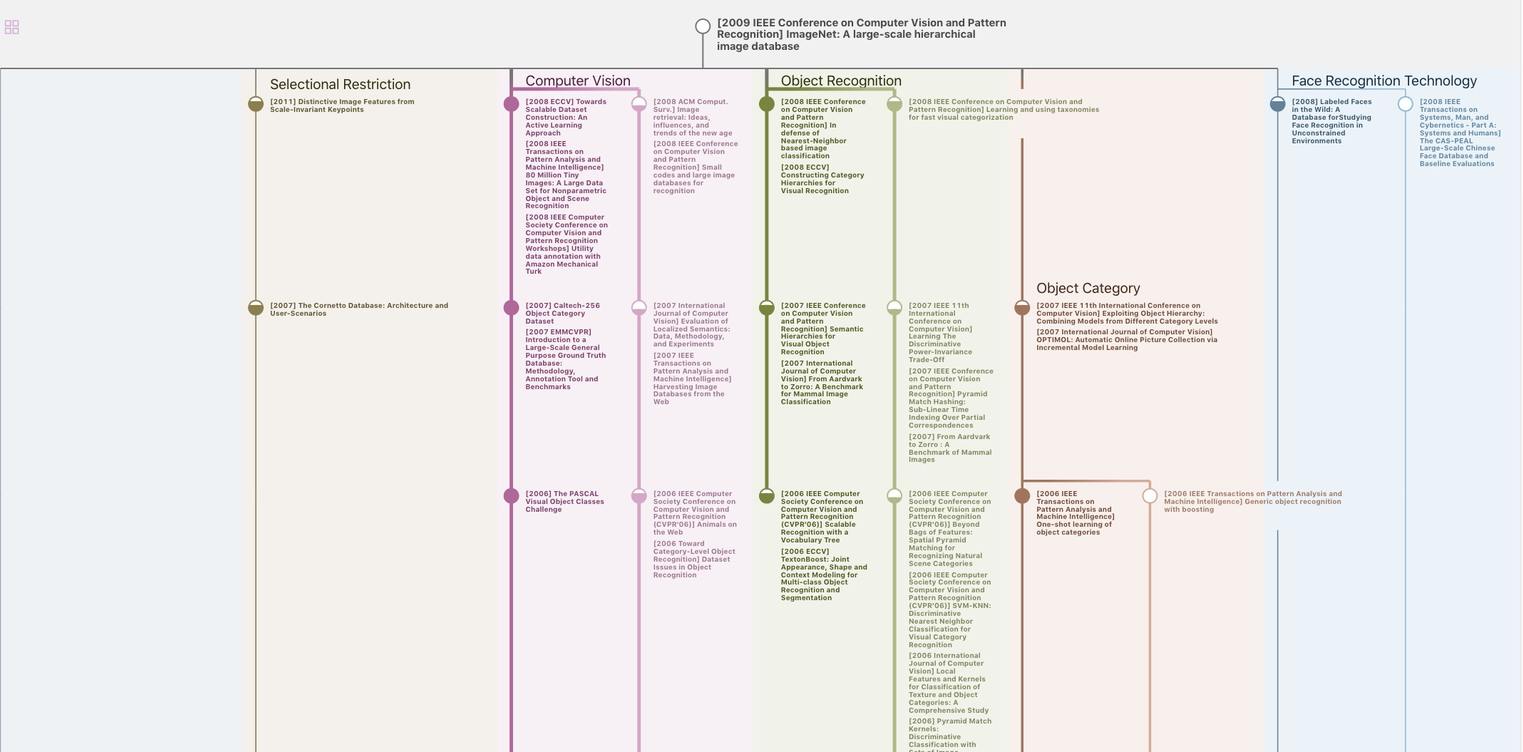
生成溯源树,研究论文发展脉络
Chat Paper
正在生成论文摘要