Deep reinforcement learning-based dual-mode congestion control for cellular V2X environments
ELECTRONICS LETTERS(2023)
摘要
The Society of Automotive Engineers (SAE) J2945/1 standard for Dedicated Short Range Communication (DSRC) environmentutilizes transmit (Tx) power control and rate control elements for the periodic BSM transmissions, which are intended to work in a complementary manner. An equivalent standard for the cellular vehicle-to-everything (C-V2X) communication environment is J3161/1, but it eliminates Tx power control and uses only rate control. However, the consequence is the degraded update delay of neighbouring vehicles' kinematics, potentially undermineing driving safety. In this Letter, the authors propose to retain the dual-mode control in the C-V2X environment and find a policy through reinforcement learning (RL) to adjust the rate control function to maintain synergy. Moreover, the authors can extract the RL-created policy from the neural network so that it can be explicitly specified in the standard, and downloaded and used more conveniently by vehicles. Finally, the RL-generated policy achieves a better packet delivery frequency than J2945/1 or J3161/1. Current V2X standards typically specify human-designed heuristic control algorithms. Their drawbacks are frequently discovered later by more thorough simulation experiments that were not done during standardization. This Letter shows that AI-generated control can be automatically obtained while running simulation, and better performing than heuristic algorithms, with the case of congestion control. Moreover, the policy can be extracted from the neural network and explicitly specified in the standard and used by vehicles instead of the neural network.image
更多查看译文
关键词
artificial intelligence, cellular radio, telecommunication congestion control, vehicular ad hoc networks
AI 理解论文
溯源树
样例
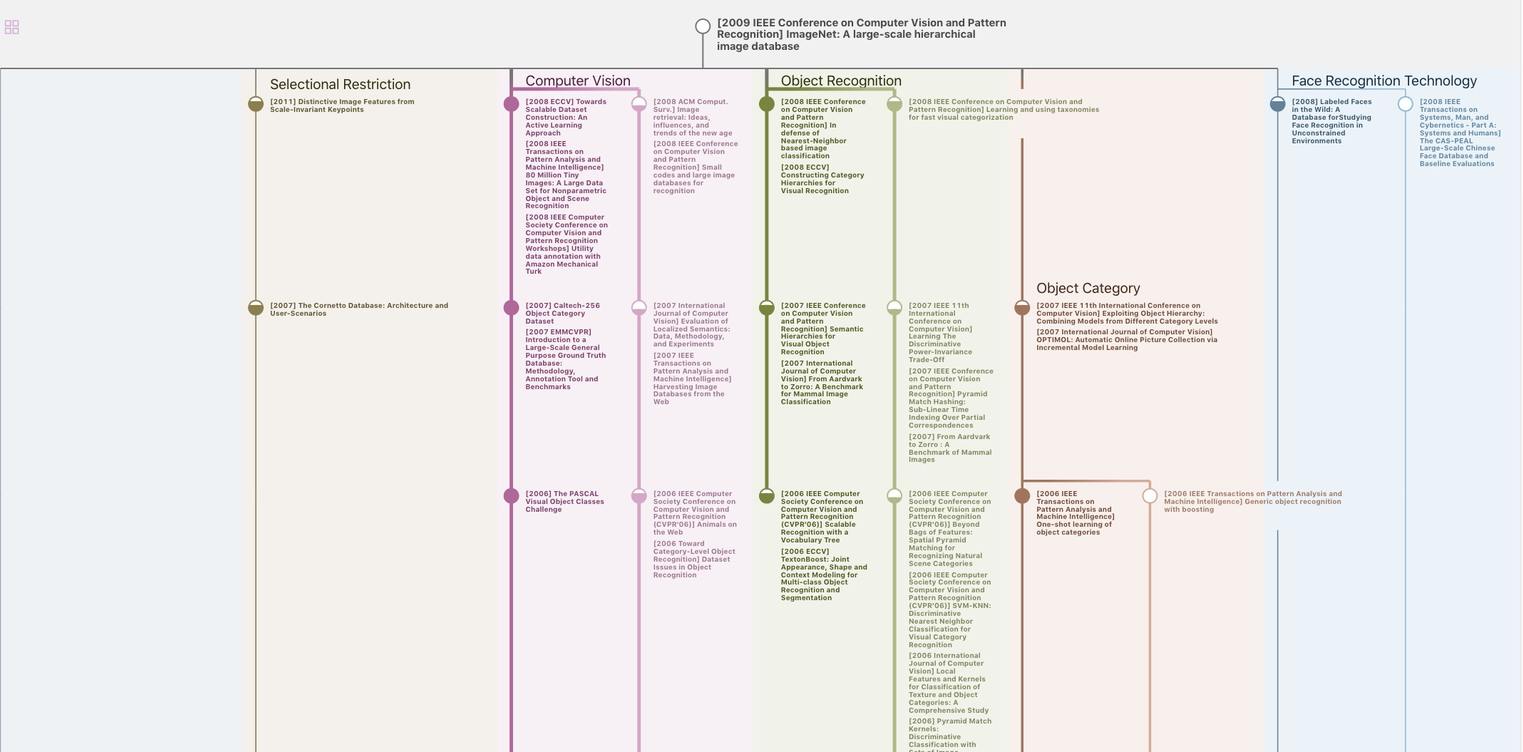
生成溯源树,研究论文发展脉络
Chat Paper
正在生成论文摘要