A novel deep transfer learning framework with adversarial domain adaptation: application to financial time-series forecasting
NEURAL COMPUTING & APPLICATIONS(2023)
摘要
Financial market prediction is generally regarded as one of the most challenging tasks in data mining. Recent deep learning models have achieved success in improving the accuracy of financial time-series forecasting (TSF), but as implicit complex information, and there have few available labeled data, the generalization capability of current benchmarks is poor in this field. To alleviate the restriction of overfitting caused by insufficient clean data, a novel deep transfer learning framework incorporating adversarial domain adaptation is proposed for financial TSF task, dubbed as ADA-FTSF for short, in improving reliable, accurate and competitive deep forecasting models. Concretely, we implement a typical adversarial domain adaptation architecture to transfer feature knowledge and reduce the distribution discrepancy between financial datasets. To reduce the shape difference during the pre-train process, a smoothed formulation of dynamic time warping (DTW) is also introduced tactfully in adversarial training phase to measure the shape loss. Notably, the confident selection of source domain from potential source datasets will make significant impact on forecasting performance. In our study, appropriate source dataset is selected using temporal causal discovery method via transfer entropy derived from copula entropy. The feasibility and effectiveness of the proposed framework are validated by the empirical experiments, ablation study, Diebold–Mariano test and parameter sensitivity analysis conducting on different financial datasets of three domains (financial indexes, energy futures and agricultural futures).
更多查看译文
关键词
Financial time-series forecasting,Deep learning,Transfer learning,Adversarial domain adaptation,Temporal causal discovery
AI 理解论文
溯源树
样例
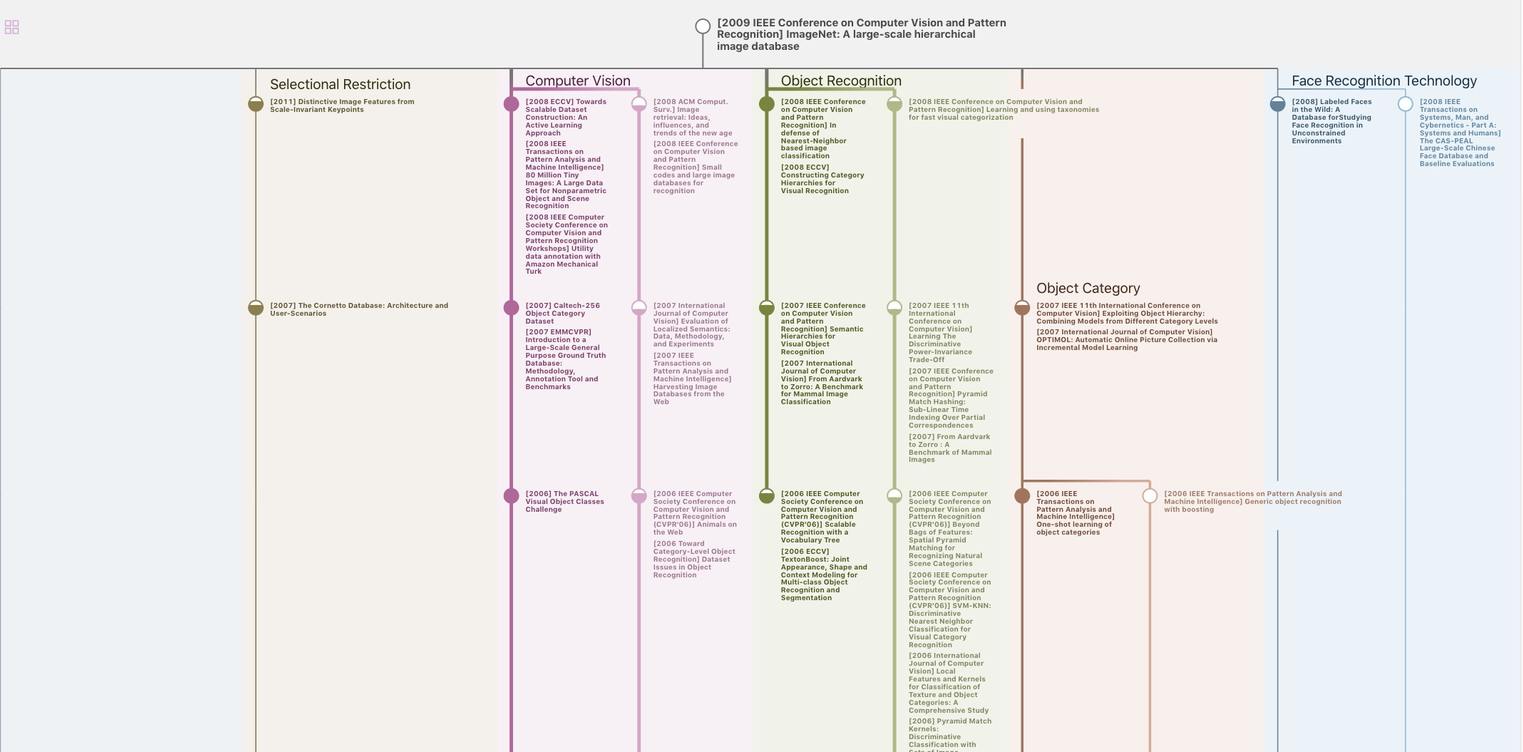
生成溯源树,研究论文发展脉络
Chat Paper
正在生成论文摘要