A multi-scale self-supervised hypergraph contrastive learning framework for video question answering
NEURAL NETWORKS(2023)
摘要
Video question answering (VideoQA) is a challenging video understanding task that requires a comprehensive understanding of multimodal information and accurate answers to related questions. Most existing VideoQA models use Graph Neural Networks (GNN) to capture temporal–spatial interactions between objects. Despite achieving certain success, we argue that current schemes have two limitations: (i) existing graph-based methods require stacking multi-layers of GNN to capture high-order relations between objects, which inevitably introduces irrelevant noise; (ii) neglecting the unique self-supervised signals in the high-order relational structures among multiple objects that can facilitate more accurate QA. To this end, we propose a novel Multi-scale Self-supervised Hypergraph Contrastive Learning (MSHCL) framework for VideoQA. Specifically, we first segment the video from multiple temporal dimensions to obtain multiple frame groups. For different frame groups, we design appearance and motion hyperedges based on node semantics to connect object nodes. In this way, we construct a multi-scale temporal–spatial hypergraph to directly capture high-order relations among multiple objects. Furthermore, the node features after hypergraph convolution are injected into a Transformer to capture the global information of the input sequence. Second, we design a self-supervised hypergraph contrastive learning task based on the node- and hyperedge-dropping data augmentation and an improved question-guided multimodal interaction module to enhance the accuracy and robustness of the VideoQA model. Finally, extensive experiments on three benchmark datasets demonstrate the superiority of our proposed MSHCL compared with stat-of-the-art methods.
更多查看译文
关键词
Video question answering,Hypergraph contrastive learning,Data augmentation,High-order relations,Multi-scale
AI 理解论文
溯源树
样例
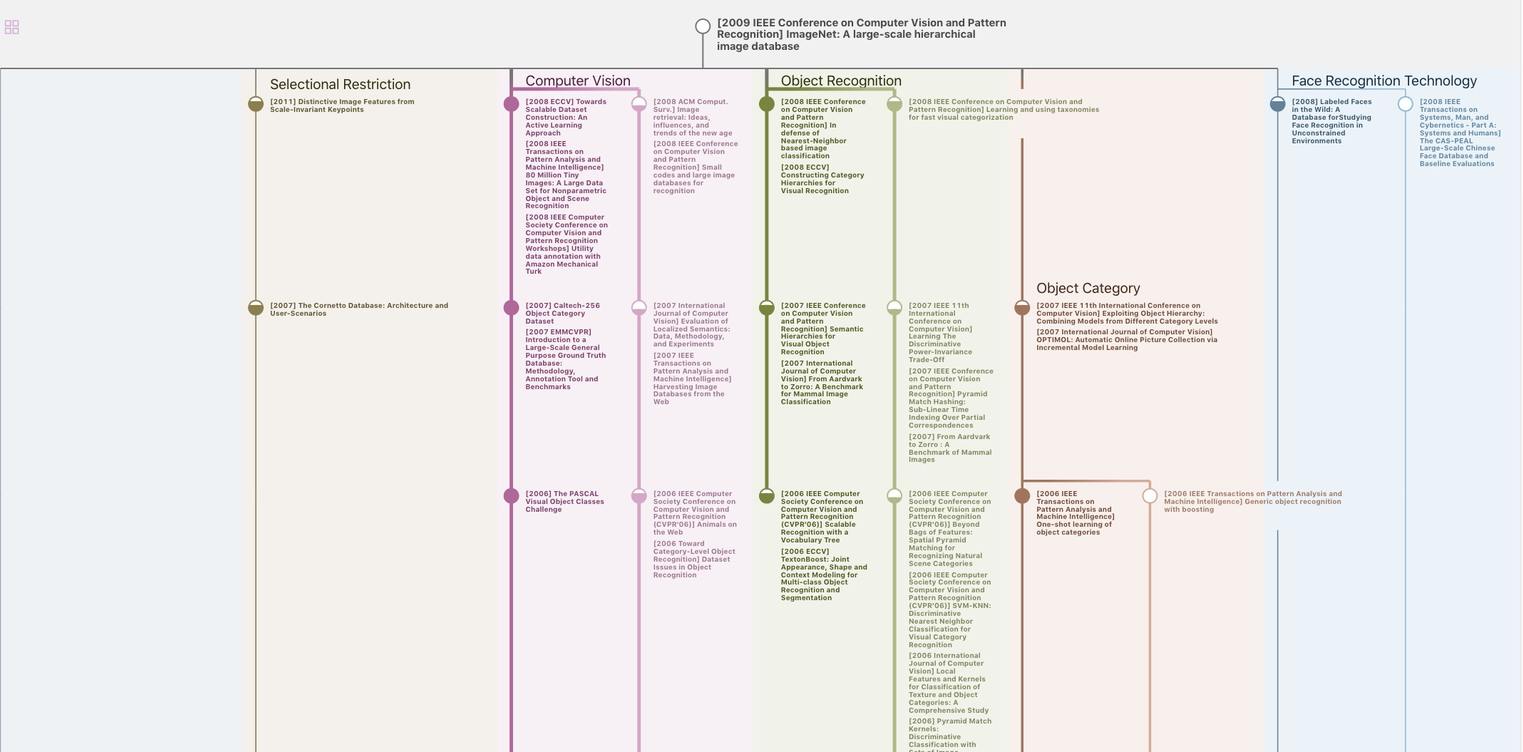
生成溯源树,研究论文发展脉络
Chat Paper
正在生成论文摘要