Understanding and Supporting Metacognitive Monitoring Judgments - A Commentary
ZEITSCHRIFT FUR ENTWICKLUNGSPSYCHOLOGIE UND PADAGOGISCHE PSYCHOLOGIE(2023)
摘要
Free AccessUnderstanding and Supporting Metacognitive Monitoring Judgments – A CommentaryMartine BaarsMartine BaarsProf. Dr. Martine Baars, Erasmus University Rotterdam, Postbus 1738, 3000 DR Rotterdam, The Netherlands, [email protected]Department of Psychology, Education and Child Studies, Erasmus University Rotterdam, The NetherlandsSearch for more papers by this authorPublished Online:September 08, 2023https://doi.org/10.1026/0049-8637/a000282PDF ToolsAdd to favoritesDownload CitationsTrack Citations ShareShare onFacebookTwitterLinkedInReddit SectionsMoreTo successfully study text materials, solve problems, and complete assignments, students must self-monitor their performance. Do they understand what they are reading? Are they taking the right steps to solve the problem? Do they understand the assignment and how to work on it? Self-monitoring is a central part of self-regulated learning (SRL, e. g., Winne & Hadwin, 1998; Zimmerman, 2002, 2008). It can be defined as the degree to which people are “metacognitively and behaviorally active participants in their own learning process” (Zimmerman, 1989, p. 4). In SRL, students typically prepare their own learning processes by planning, choosing strategies, and setting goals in relation to their motivations to learn. Next, students self-monitor their own learning process to check how the process is going and whether they would need to adjust it according to their goals or other standards. Finally, in SRL students end a particular learning process by evaluating how they did and whether they were satisfied about it in relation to their goals. They then use this information for yet another cycle of SRL (e. g., Zimmerman, 2013). However, we know that people are poor at judging their own performance (e. g., Bjork et al., 2013; Dunlosky & Lipko, 2007; Dunning et al., 2003). That is, people usually overestimate themselves (e. g., Bjork et al., 2013; Dunning et al., 2003), causing them to prematurely stop studying materials (e. g., Dunlosky & Rawson, 2012). Therefore, it is crucial to gain more insight into the process of metacognitive monitoring during learning to support students’ SRL processes and subsequently their academic performance. Fortunately, understanding and fostering metacognitive judgment accuracy is central to the current special issue. Indeed, the eight contributions in this special issue investigate what metacognitive monitoring looks like and how we can best support it.According to the model by Nelson and Narens (1994), self-monitoring helps us to obtain information about our performance from the object-level to a meta-level. That is, whatever is happening at the object-level, for example, while reading the text, can be analyzed at the meta-level. This is useful because, at the meta-level, actions to control or regulate the learning process can be initiated. For example, if the text you are reading does not make sense to you, this information could be brought up to the meta level, where it is analyzed. This leads you to act and reread the bit of the text you did not get. Thus, accurate monitoring processes can lead to accurate regulation processes, which in turn can improve performance (e. g., Broadbent & Poon, 2015; Dent & Koenka, 2016; Dignath et al., 2008; Dignath & Büttner, 2008; Thiede et al., 2003).To investigate self-monitoring processes, we can use different types of monitoring or calibration judgments at different moments in the learning process (see Schraw, 2009, for a taxonomy of calibration judgments). For example, a Judgment of Learning (JOL) is a prospective judgment made before testing about how much information is subsequently recollected. Other judgments made before testing are Ease of Learning (EOL) judgment about the relative ease of learning information and a Feeling of Knowing (FOK) judgment about the likelihood of recognizing information that could not be recalled. There are also concurrent self-monitoring judgments that can be made during testing, such as event-related confidence judgments about confidence in one’s performance as well as ease of solution judgments and online performance accuracy judgments about the accuracy of performance. The third category of self-monitoring judgments according to the taxonomy by Schraw (2009) is retrospective judgments, such as retrospective judgment of performance or ease of learning or solution judgments.However, research has shown that monitoring accuracy is generally low (e. g., Dunlosky & Lipko, 2007; Thiede et al., 2009). Interestingly, studies have found several ways to improve monitoring using word pairs (e. g., Nelson & Dunlosky, 1992), learning from text (e. g., Thiede et al., 2009) and learning to solve problems (e. g., Baars et al., 2013, 2014, 2017). For example, the Delayed JOL effect (e. g., Nelson & Dunlosky, 1992; Rhodes & Tauber, 2011) showed that delaying JOLs about being able to recall the second item in a word pair on a subsequent test improved when made after studying the complete list of word pairs compared to right after each word pair. For learning from text, a range of generative strategies were found to improve self-monitoring accuracy when applied at a delay after the reading of a set of texts (e. g., Anderson & Thiede, 2008; Thiede et al., 2005) as well as immediately after the reading of a single text (for a meta-analysis, see Prinz et al., 2020). For example, delayed keywords (De Bruin et al., 2011; Thiede et al., 2005) and delayed summaries (Anderson & Thiede, 2008) improved JOLs about text comprehension. Immediate generative strategies such as making concept maps (Redford et al., 2012) and self-explaining (Griffin et al., 2008) also improved JOLs about text comprehension. When learning to solve problems, it is also helpful to generate (part of) the problem-solving procedure to improve self-monitoring accuracy (Baars et al., 2013). For example, using a practice test after a learning phase with worked examples improved the accuracy of self-assessments of current performance on the problem-solving task (Baars et al., 2014, 2017).Because these findings on how to improve self-monitoring opened new avenues to investigate how people monitor their own learning process, there are still many unanswered questions. In relation to the development of SRL skills such as self-monitoring, it is often unclear from what age self-monitoring supports are helpful and whether this would also affect subsequent regulation and performance. Moreover, using strategies to improve self-monitoring presumably helps students to improve their self-monitoring judgments because they access cues on which to base their judgments (i. e., cue-utilization, Koriat 1997). Yet, it is largely unclear what type of cues are diagnostic in relation to subsequent performance on different types of tasks at different ages (e. g., Baars et al., 2020; David et al., 2023). The contributions to this special issue provide valuable insights into strategies to support the self-monitoring and regulation of learning; factors to consider when investigating the self-monitoring process; and how we can measure self-monitoring. We can distinguish at least four lines of work in the contributions to this special issue which I used to structure this commentary. The first line relates to generative strategies such as creating keywords, writing summaries, or using retrieval tasks. The second line is providing feedback on the cognitive and on the metacognitive level. The third line covers the role of emotions during self-monitoring learning. And finally, a fourth line of work concerns approaches to measuring self-monitoring and self-regulation of learning. I briefly summarize the contributions and subsequently discuss some overarching questions and insights that arise from these contributions.Generative StrategiesWaldeyer and Roelle (2023) investigate whether providing expert keywords to compare to self-made keywords would improve self-monitoring accuracy and regulation in high school students (Mage = 14.43 years, SDage = 0.55). Based on earlier findings in higher education, it was expected that being able to compare expert keywords to one’s own keywords would improve self-monitoring accuracy and subsequent regulation of learning. High-school students read three expository texts about varying topics and provided keywords either without comparing them to expert keywords or comparing them to expert keywords. All participants provided Judgments of Performance (JOP) by rating how many questions about the text they would be able to answer correctly before and after generating keywords. In contrast to earlier findings with university students (Waldeyer & Roelle, 2021), we found no differences in self-monitoring accuracy or regulation. The study by Schuster et al. (2023) investigated whether Twitter-like summaries of historical texts provided at a delay or immediately after a set of texts improved self-monitoring. Adult learners (Mage = 23.07 years, SDage = 5.05) read a set of three texts with different views on a historical event. After reading the set of three texts, participants either created immediate Twitter-like summaries, delayed Twitter-like summaries after a distracter task, or no summaries. Participants in the experimental conditions wrote Twitter-like summaries from the standpoints of each of the three historians they had read about. Interestingly, the authors considered not only the classic measure of self-monitoring accuracy of the content of the text was but also self-monitoring in relation to knowledge of source-content links, knowledge of intertextual conflicts, and the quality of written essays. All participants provided JOPs globally across the three texts in relation to each dependent measure (i. e., content knowledge, intertextual conflict knowledge, source-content links knowledge, and essay task). It was expected that generating delayed summaries would improve self-monitoring compared to immediate summaries or no summaries. The findings revealed that delayed Twitter-like summaries indeed improved self-monitoring accuracy about the content knowledge but not for the other dependent measures concerning intertextual conflict knowledge, source-content links knowledge, and essay task.Hoch et al. (2023) investigated whether monitoring prompts can reduce confidence bias when learning from multimedia learning materials and using generative retrieval practice activities. It was expected that monitoring accuracy would improve by asking participants to attend more to experience-based cues, such as their confidence in understanding the materials, their invested mental effort, and the difficulty of the learning materials. Moreover, when participants focused on the experience-based cues, they were expected to also change their subsequent learning behavior. That is, participants who received monitoring prompts were expected to spend more time on studying and retrieval practice activities and perform better on retrieval practice and the test. In this study, all participants (Mage = 23.8, SDage = 4.2) engaged in studying the concept of mitosis in a multimedia learning environment using generative retrieval practice activities. Half were prompted to monitor experience-based cues several times throughout the learning process. All participants provided confidence judgments about their performance at a later test. Contrary to the authors‘ expectations, there were no differences in monitoring accuracy between participants prompted to monitor experience-based cues during learning and those not prompted to do this. However, the results seem to suggest that learning behaviors (e. g., answer length in retrieval practice) can potentially mediate the relation between monitoring and learning performance.The study by Endres et al. (2023) investigated the effect of retrieval tasks on re-learning materials. The authors argue that retrieval opportunities can help learners to enhance their self-monitoring accuracy and regulation quality during SRL. That is, retrieval opportunities can help monitoring knowledge and identify knowledge gaps. Moreover, identifying knowledge gaps can motivate students to spend more time and effort on the learning process. Therefore, it was hypothesized that having retrieval opportunities would lower monitoring judgments (i. e., JOLs), making them more accurate, increasing relearning time, motivation (i. e., situational interest), self-efficacy, mental effort, and immediate learning outcomes. Participants in this study read expository texts about coffee and sugar and were invited to either restudy the text or engage in a retrieval task across two sessions. That way, all participants engaged in restudy and retrieval across the two sessions. The granularity of the task was varied between conditions. Participants in the coarse-grained condition were asked to retrieve everything they had learned from the text, whereas the participants in the fine-grained conditions were asked to answer 9 more specific questions about the text. Findings in this study showed that participants performed better if they engaged in the retrieval task in session 1 but not if they did this in session 2. Indeed, participants who engaged in retrieval showed lower JOLs and longer relearning times. However, longer relearning times did not explain the effect of retrieval activities on immediate learning outcomes. Lower JOLs and self-efficacy did mediate the effect of the retrieval activities on immediate learning outcomes, showing that both the magnitude of the JOLs and self-efficacy are associated with the benefits of the retrieval activities. It was found that students focused their relearning efforts on the subsections of the text that were the least well retrieved. This indicates that the granularity of the retrieval task is related to monitoring and regulation processes.To sum up, the contributions in this special issue that concern generative strategies to help learners monitoring their performance using a more cognitive (e. g., keywords, Waldeyer & Roelle, 2023; summaries, Schuster et al., 2023; or retrieval tasks, Endres et al., 2023) or metacognitive approach (e. g., experience-based cues, Hoch et al., 2023) showed mixed results across the studies. Although, it can be helpful to engage in generative activities (e. g., summaries, Schuster et al., 2023; retrieval tasks, Endres et al., 2023), it did not enhance monitoring and performance on all measures (e. g., Schuster et al., 2023) or replicated in younger samples (e. g., Waldeyer & Roelle, 2023). Hence, we need a more thorough understanding of what students are doing while engaging in generative strategies, what other factors are involved (e. g., effort and motivation), and how this could help them to use valid cues to monitor and regulate their learning processes. For example, cues based on one’s understanding of the learning material might not be as valid for other types of outcome measures (e. g., source-content links, Schuster et al., 2023). Also, the „quality“ of the generative activity might be a more powerful predictor of monitoring and regulation accuracy compared to the „quantity“ of a generative activity (e. g., time spent on relearning, Endres et al., 2023). Also, because there might be an interaction between cues made available by using generative strategies and monitoring prompts (e. g., Hoch et al., 2023), it would be promising for future research to also zoom in on this interaction between generative strategies and monitoring prompts.FeedbackKolloff et al. (2023) investigated the effect of item-specific monitoring and/or performance feedback on the relative accuracy of monitoring during a paired-associates task in a sample of 1st-grade children (Mage = 7 years, 4 months, SDage = 4.8 months). The study investigated whether overconfidence in monitoring could be reduced using item-specific feedback. The authors hypothesized that monitoring feedback about whether a monitoring judgment matches actual performance would help children to more accurately distinguish between accurate and inaccurate items compared to the performance feedback condition. Children were randomly assigned to either the performance feedback or the monitoring feedback condition. After studying a picture pair of an animal and its food, they were asked whether they recognized the right food for the animal shown using multiple choice recognition. Then the children provided a confidence judgment about how certain they were about their answer on a 4-point smiley scale. Next, children received feedback either on their performance or on their performance and their monitoring (i. e., confidence judgment). The results concerning the relative accuracy of monitoring showed that children were able to discriminate between accurate and inaccurate responses. Yet, children were found to be overconfident, as witnessed in the absolute accuracy of their monitoring judgments. The type of feedback children received throughout the study did not improve their monitoring accuracy.Thus, although children are already able to distinguish between what they know and what they do not know, providing feedback to diminish overconfidence in their judgments did not yield the expected effect (Kolloff et al., 2023). Interestingly, the authors also refer to other factors that might come into play when incorporating feedback, such as self-protection mechanisms. This seems an interesting avenue for future studies to explore. Specifically, it would be interesting to investigate the long-term effects of supporting self-monitoring using feedback, in relation to affect, emotions, and motivation students experience during learning.The Role of EmotionsIn an experiment with university students, Prinz-Weiß et al. (2023) investigated to what extent hope or hopelessness play a role during monitoring learning from text and whether a reattribution technique could remediate negative effects. Activating-positive emotions such as hope support and deactivating-negative emotions such as hopelessness can undermine learning processes as well as influence people’s monitoring judgments. The authors expected that higher hopelessness would be associated with lower performance and more underconfidence in monitoring judgments compared to lower hopelessness. Yet, a recue instruction (i. e., reattribution technique) was expected to diminish the effect on monitoring in the experimental condition. The participants (Mage = 22.26, SDage = 5.09) received instructions to induce hopelessness, hope, or a neutral state and were randomly assigned to either the recue condition or the control condition. In the recue condition, the participants received instruction about how emotions can negatively affect monitoring and the advice to focus on their comprehension instead. The participants read an expository text about a heat pump. Both pre- and postdictions about the number of correct comprehension questions were measured as well as comprehension performance. Findings in this experiment showed that participants indeed used their emotions to make monitoring judgments, even though this cue could be of limited validity. Yet the recue instruction did not significantly moderate the association between the induced emotions, comprehension, or metacomprehension (i. e., monitoring accuracy). Interestingly, postdictions in the recue condition were more accurate compared to the control condition. This indicates that participants did use the recue instructions to make more accurate postdictions about their performance.Hence, this study by Prinz-Weiß et al. (2023) seems to confirm that feelings of hope or hopelessness were used to make monitoring judgments. It is valuable to gain insight into this process of using different types of emotions in SRL processes. As it is still unclear what different types of emotions could be considered a valid or diagnostic cue for self-monitoring learning, it poses an interesting challenge for researchers in the field.How and When to Measure SRLThe study by Dörrenbächer-Ulrich and Perels (2023) analyzed the relationship between monitoring judgments and the metacognitive component of SRL (SRL-MC). The SRL-MC was measured by an 18-item SRL questionnaire, a strategy knowledge test, and a microanalytic assessment of metacognitive components during learning. Making an accurate monitoring judgment is important to selecting the right strategies to perform well, but it is still largely unclear how more event-related measures such as monitoring judgments relate to more stable trait-like measures such as SRL abilities. Also, there are individual differences in how people perform across different types of monitoring judgments. Using a person-oriented approach (i. e., Latent Profile Analysis, LPA), the authors explored profiles of monitoring judgment performance. Participants read expository texts about an Asian ball sport and provided three types of monitoring judgments: 1) Global Prospective Bias (GPB), in which participants predicted number of correct items on the test; 2) Global Judgment on Learning Process Quality (JOL-PQ), in which participants judged the quality of their learning process; and 3) Local Retrospective Bias (LRB), in which participants indicated how certain they felt about their answer. The results showed four profiles of monitoring judgment performance: 1) self-assured and accurate, 2) self-doubting but overconfident, 3) self-doubting and strongly underconfident, and 4) self-assured and strongly overconfident. In terms of performance, the profiles seem to fall into two groups:The self-assured and accurate and the self-doubting and strongly underconfident groups scored highest, and the self-doubting but overconfident and the self-assured and strongly overconfident groups scored lowest. Next, it was expected that participants who would show more accurate monitoring judgments should also score higher on more general SRL measures. However, the results seem to indicate that the profiles do not differ significantly on the SRL-MC measures.Händel et al. (2023) investigated monitoring in different phases in the learning process during a regular psychology course. That is, monitoring can be aimed at the preparation phase (e. g., monitoring comprehension) or the testing phase (e. g., monitoring correctness of given answers). In both phases, monitoring could be focused on processing information (e. g., monitoring learning effectiveness) or on retrieving information (e. g., correctness of given answers). It was hypothesized that this leads to 4 different objects of monitoring: 1) monitoring learning effectiveness, 2) monitoring comprehension, 3) monitoring test processing, and 4) monitoring correctness of given answers. Also, it was expected that the relationship between monitoring judgments within one phase (i. e., preparation or testing) is stronger than that across phases. Monitoring processes were measured across phases and objects in an ecologically valid setting in higher education using self-reports and monitoring judgments. Results confirmed both hypotheses, namely, different monitoring objects could be empirically distinguished and monitoring objects within a phase were related, whereas this was less the case across different phases.In sum, it matters how and when we measure self-monitoring and regulation of learning processes. The results found by Dörrenbächer-Ulrich and Perels (2023) make clear that there is still a divide between our offline, trait-like measures of SRL (e. g., questionnaires) compared to event-related measures (e. g., monitoring judgments). Even with the profiles found across different types of monitoring judgments in relation to performance, the association with offline SRL measures remained unclear. In addition, it might not surprise to learn from the study by Händel et al. (2023) that there are different objects to monitor across different phases. Future research could use these insights to have a more fine-grained approach to investigating the self-monitoring and regulation of learning. It would be valuable to define what objects are monitored in what phase of the learning process during a study to gain insight into when to intervene on monitoring what specific object to get the most optimal support for self-monitoring and regulation of learning. Conclusion and DiscussionThe contributions to this special issue focused on how to investigate and improve metacognitive monitoring and self-regulation of learning. In doing so, they gained many valuable insights and taised new research questions. An important insight would be that, in line with other research (see Prinz et al., 2020), generative activities can help learners to self-monitor their learning process more accurately (e. g., Endres et al., 2023; Schuster et al., 2023). Moreover, interestingly, some of the generative strategies that seem to work with older samples were not found to benefit the self-monitoring accuracy in younger samples (e. g., Waldeyer & Roelle, 2023). In addition, using generative strategies to improve self-monitoring accuracy may be limited to certain types of learning outcomes (e. g., comprehension performance) and not apply to others (e. g., source-context links, Schuster et al., 2023). Next to generative activities, feedback to support self-monitoring might also have different effects across different samples, learning tasks or settings. In contrast to earlier studies (e. g., Van Loon & Roebers, 2020), Kolloff et al. (2023) found no effects of performance and monitoring feedback on the relative self-monitoring accuracy in a primary school sample. Yet, in line with previous findings, children were able to distinguish between what they know or do not know. These findings invite more research on the relationship between using different types of support to improve self-monitoring accuracy (e. g., generative strategies or feedback) in relation to different learning outcomes and the development of learners’ metacognitive abilities.Another interesting insight in the contributions to this special issue relates to when and how we measure self-monitoring and regulation of learning. It matters whether we choose to measure offline, trait-like SRL abilities, use event-related SRL measures, or monitoring judgments. Different types of measures of SRL do not seem to capture the same SRL ability in learners (Dörrenbächer-Ulrich & Perels, 2023). Also, more fine-grained approaches in which it is defined what the object (i. e., comprehension or effectiveness) is learners would need to monitor during which phase (i. e., preparation or testing) in their learning process (e. g., Händel et al., 2023) could potentially provide us with more insights on how self-monitoring works and why it is often limited in its accuracy.Table 1 shows an overview of the different types of monitoring judgment used across the contributions to this special issue. We can infer that monitoring judgments that prompt the learner to judge performance in another phase of the learning process are often used in research. That is, all monitoring judgments in the first column of the table are prospective judgments in which learners predicted their performance at the test while still in the preparation or learning phase. However, findings in this special iss
更多查看译文
关键词
metacognitive monitoring judgments,understanding
AI 理解论文
溯源树
样例
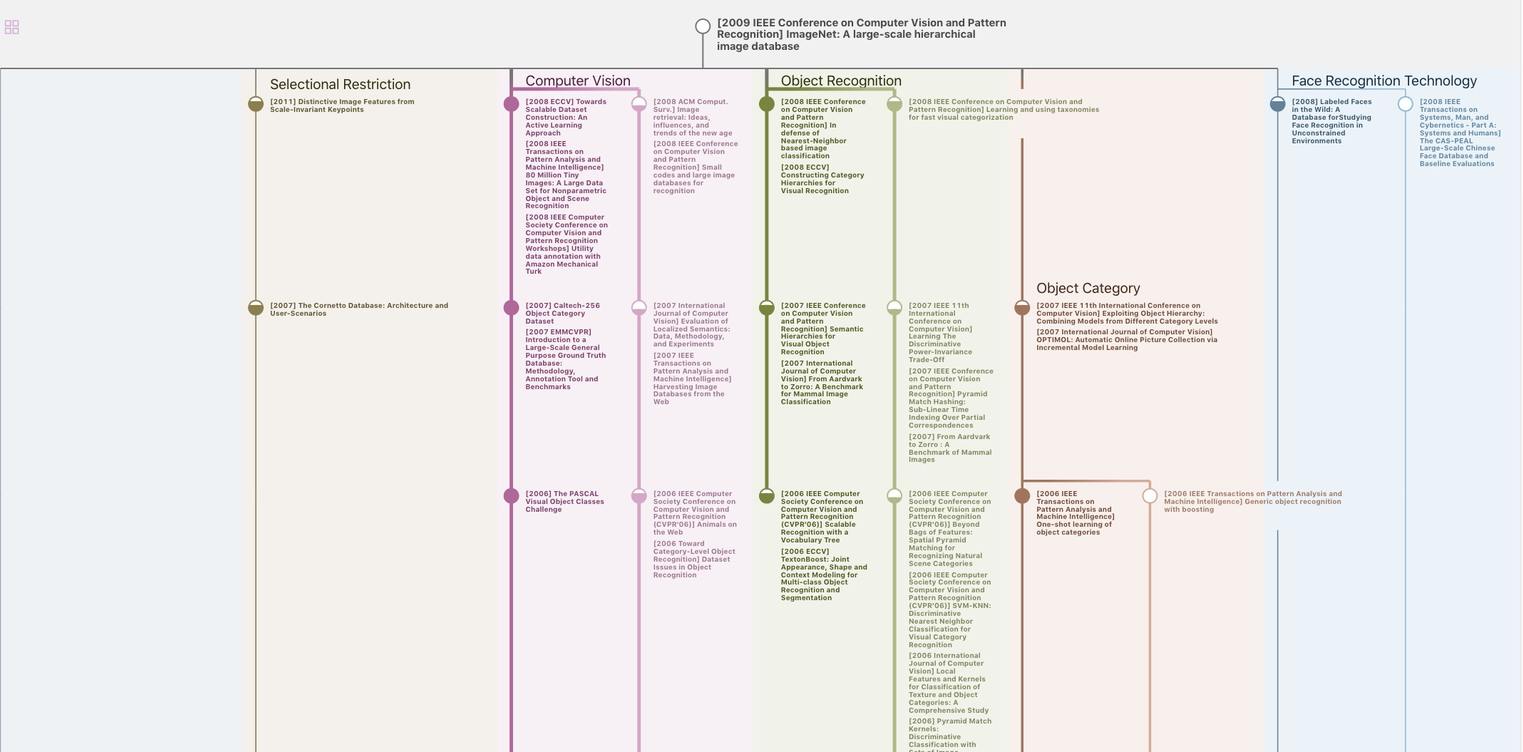
生成溯源树,研究论文发展脉络
Chat Paper
正在生成论文摘要