Weakly-supervised Deep Cognate Detection Framework for Low-Resourced Languages Using Morphological Knowledge of Closely-Related Languages.
CoRR(2023)
Abstract
Exploiting cognates for transfer learning in under-resourced languages is an exciting opportunity for language understanding tasks, including unsupervised machine translation, named entity recognition and information retrieval. Previous approaches mainly focused on supervised cognate detection tasks based on orthographic, phonetic or state-of-the-art contextual language models, which under-perform for most under-resourced languages. This paper proposes a novel language-agnostic weakly-supervised deep cognate detection framework for under-resourced languages using morphological knowledge from closely related languages. We train an encoder to gain morphological knowledge of a language and transfer the knowledge to perform unsupervised and weakly-supervised cognate detection tasks with and without the pivot language for the closely-related languages. While unsupervised, it overcomes the need for hand-crafted annotation of cognates. We performed experiments on different published cognate detection datasets across language families and observed not only significant improvement over the state-of-the-art but also our method outperformed the state-of-the-art supervised and unsupervised methods. Our model can be extended to a wide range of languages from any language family as it overcomes the requirement of the annotation of the cognate pairs for training. The code and dataset building scripts can be found at https://github.com/koustavagoswami/Weakly_supervised-Cognate_Detection
MoreTranslated text
Key words
deep cognate detection framework,morphological knowledge,languages,weakly-supervised,low-resourced,closely-related
AI Read Science
Must-Reading Tree
Example
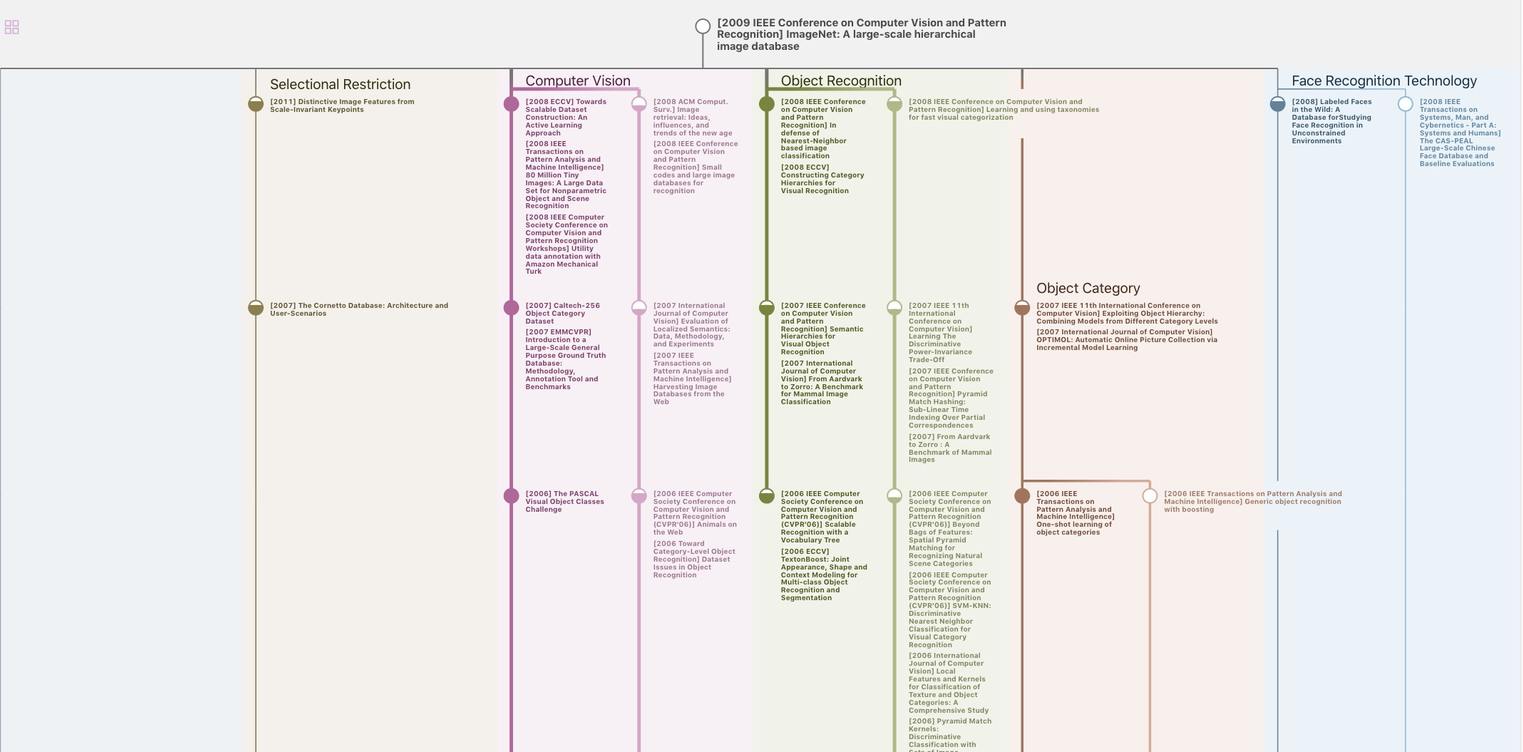
Generate MRT to find the research sequence of this paper
Chat Paper
Summary is being generated by the instructions you defined