Parsimonious predictors for medical decision support: Minimizing the set of questionnaires used for tinnitus outcome prediction
EXPERT SYSTEMS WITH APPLICATIONS(2024)
摘要
Comprehensive assessment and treatment planning for chronic tinnitus requires the collection of multidimensional self-report data which may pose a response burden upon patients. In this study, we use the example of patients with chronic tinnitus to investigate the extent to which the scores of possible treatment outcomes such as tinnitus-related distress and depressed mood can be predicted by the answers to other questionnaires, so that assessments can be performed reliably with minimal respondent burden.We propose a parsimonious learning approach that predicts multiple outcomes at screening and after treatment, using a minimal low-cost set of questionnaires for the index symptom tinnitus. We model a questionnaire's "cost"on the basis of items to be answered in order to compute its score. We consider conventional regression and multi-output algorithms and incorporate them into a workflow that varies the input to the predictor by selecting different questionnaires, assesses the contribution of each questionnaire to prediction quality and ranks questionnaires on contribution versus cost.For our study with this new approach, we obtained access to a random sample of n = 500 out of 1287 Charite patients with a tinnitus duration of at least 3 months, treated at Charite between January 2011 and October 2015 with a multimodal psychosomatic therapy, and for whom no values were missing in the nine questionnaires under study (238 items total). We used this data to predict three outcomes of interest: tinnitus-related distress, perceived stress and depression before and after treatment.Results indicated that two (of nine) questionnaires sufficed in predicting the target outcomes with negligible trade-offs. Learning approaches can be helpful in using patient-generated data to construct questionnaire assessments that ideally balance informational value with patients' response burden.
更多查看译文
关键词
Tinnitus,Prediction,Medical decision support
AI 理解论文
溯源树
样例
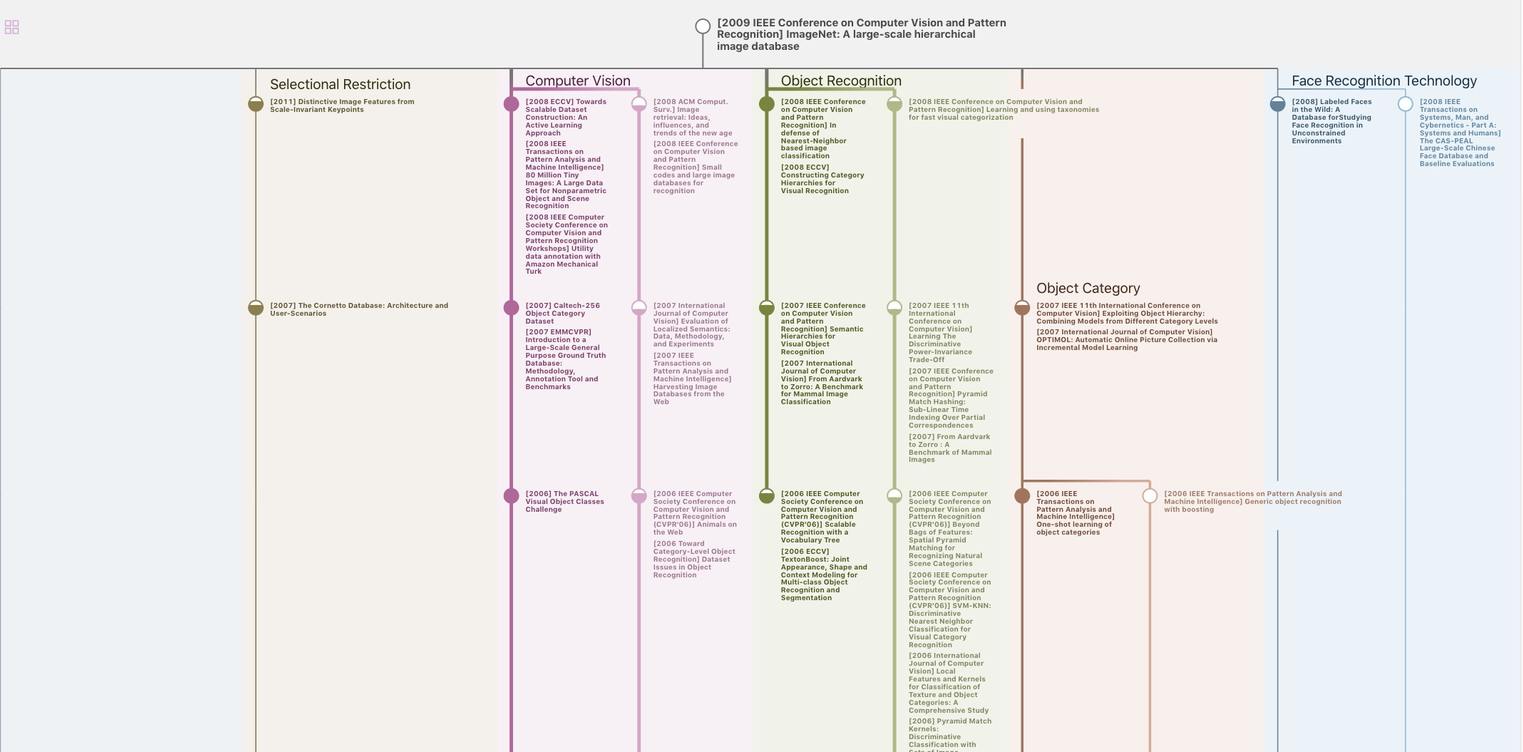
生成溯源树,研究论文发展脉络
Chat Paper
正在生成论文摘要