Deep neural network for identification of impaired microvascular and vasomotor function from stress electrocardiography.
medRxiv : the preprint server for health sciences(2023)
摘要
Impaired microvascular and vasomotor function is a common consequence of aging, diabetes, and other risk factors, and is associated with adverse cardiac outcomes. Such impairments are not readily identified by standard clinical methods of cardiovascular testing such as coronary angiography and noninvasive single photon emission tomography (SPECT) myocardial perfusion imaging (MPI). We hypothesized that signals embedded within stress electrocardiograms (ECGs) identify individuals with microvascular and vasomotor dysfunction.
Methods:We developed and validated a novel convolutional neural network (CNN) using stress and rest ECG data (ECG-Flow) to identify patients with impaired myocardial flow reserve (MFR) on quantitative positron emission tomography (PET) MPI (N=3887). Diagnostic accuracy was validated with an internal holdout set of patients undergoing stress PET MPI (N=963). The prognostic association of ECG-Flow with mortality was then evaluated in a separate cohort of patients undergoing SPECT MPI (N=5102).
Results:ECG-Flow achieved good diagnostic accuracy for impaired MFR in the holdout PET cohort (AUC, sensitivity, specificity: 0.737, 71.1%, 65.7%). Abnormal ECG-Flow was found to be significantly associated with mortality in both PET holdout and SPECT MPI cohorts (adjusted HR 2.12 [95 ρ CI 1.45, 2.10], ρ = 0.0001, and 2.07 [1.82, 2.36], ρ < 0.0001, respectively).
Conclusion:Signals predictive of microvascular and vasomotor dysfunction are embedded in stress ECG waveforms. These signals can be identified by deep learning methods and are related to prognosis in patients undergoing both stress PET and SPECT MPI.
更多查看译文
关键词
stress electrocardiography,deep neural network,neural network
AI 理解论文
溯源树
样例
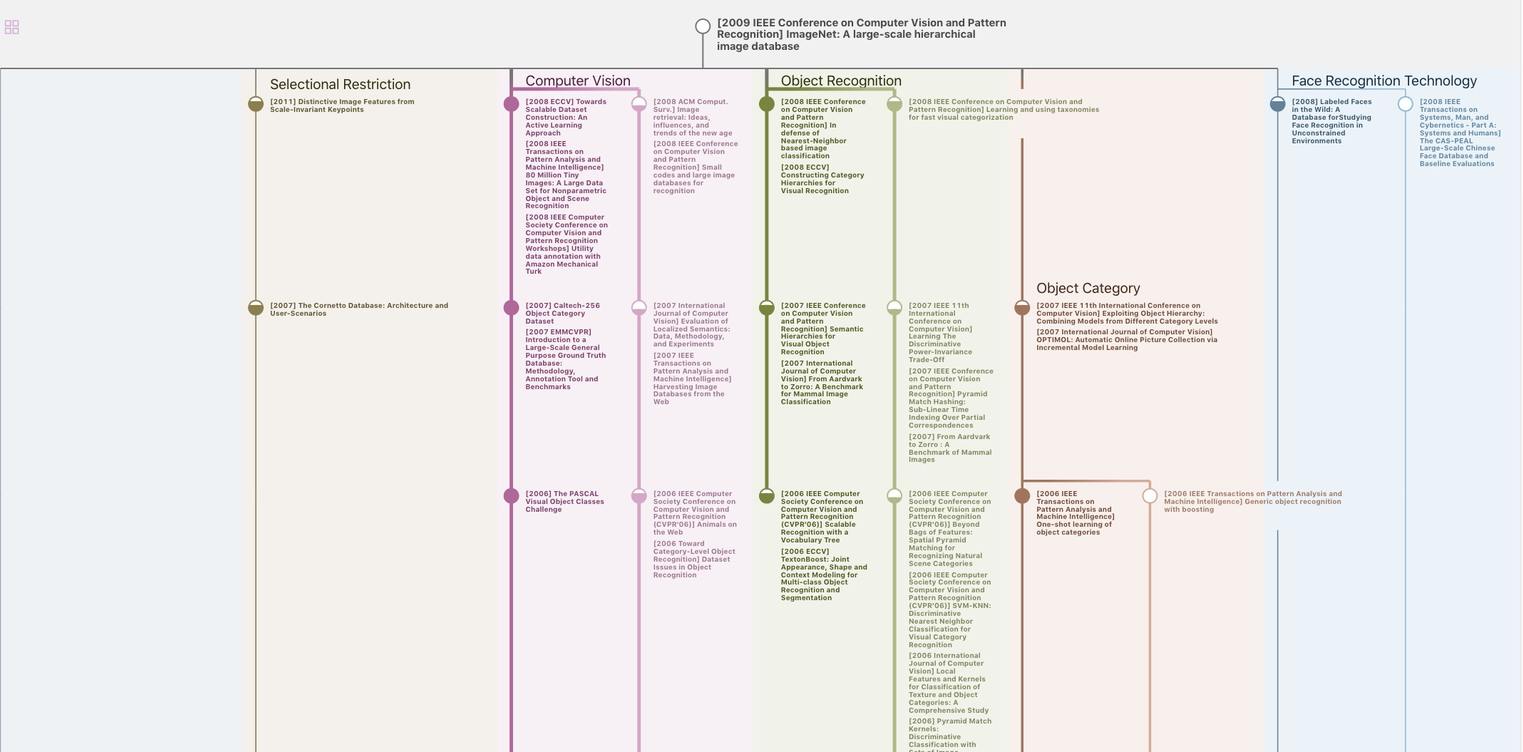
生成溯源树,研究论文发展脉络
Chat Paper
正在生成论文摘要