Automatic Detection of Cardiac Conditions from Photos of Electrocardiogram (ECG) Captured by Smartphones
medrxiv(2023)
摘要
Background Artificial intelligent electrocardiogram (ECG) diagnostic algorithms can achieve cardiologist-level accuracy, but their clinical use is limited as they cannot be installed in older ECG machines.
Objective To develop a smartphone application that extracts and analyzes ECG waveforms from photos using machine learning techniques.
Methods A smartphone application was developed to automatically extract ECG waveforms from photos taken by clinicians using computer vision and machine learning. Custom designed machine learning models were developed to perform waveform identification, gridline removal, and scale calibration. The extracted voltage-time series waveforms were analyzed using a pre-trained machine learning-based diagnostic algorithms, and the accuracy of the proof-of-concept application was assessed.
Results Waveforms from 40,516 scanned and 444 photographed ECGs were automatically extracted. 12,828 of 13,258 (96.8%) scanned and 5,399 of 5,743 (94.0%) photographed waveforms were correctly cropped and labelled. 11,604 of 12,735 (91.1%) scanned and 5,062 of 5,752 (88.0%) photographed waveforms achieved successful voltage-time signal extraction after automatic gridline and background noise removal. The AF diagnostic algorithm achieved 91.3% sensitivity, 94.2% specificity, 95.6% positive predictive value, 88.6% negative predictive value and 93.4% F1 score.
Conclusion Using computer vision and machine learning techniques to detect cardiac conditions from photos of ECGs taken with smartphones is feasible. This platform can enable widespread deployment of the latest machine learning-based ECG diagnostic algorithms.
### Competing Interest Statement
The authors have declared no competing interest.
### Clinical Trial
Not clinical trial
### Funding Statement
None.
### Author Declarations
I confirm all relevant ethical guidelines have been followed, and any necessary IRB and/or ethics committee approvals have been obtained.
Not Applicable
The details of the IRB/oversight body that provided approval or exemption for the research described are given below:
Institutional Review Board of the University of Hong Kong/Hospital Authority Hong Kong West Cluster
I confirm that all necessary patient/participant consent has been obtained and the appropriate institutional forms have been archived, and that any patient/participant/sample identifiers included were not known to anyone (e.g., hospital staff, patients or participants themselves) outside the research group so cannot be used to identify individuals.
Not Applicable
I understand that all clinical trials and any other prospective interventional studies must be registered with an ICMJE-approved registry, such as ClinicalTrials.gov. I confirm that any such study reported in the manuscript has been registered and the trial registration ID is provided (note: if posting a prospective study registered retrospectively, please provide a statement in the trial ID field explaining why the study was not registered in advance).
Not Applicable
I have followed all appropriate research reporting guidelines, such as any relevant EQUATOR Network research reporting checklist(s) and other pertinent material, if applicable.
Not Applicable
Anonymized data will be available from the corresponding author upon reasonable requests up to 3 years after publication of the article. Raw images of the electrocardiograms (ECGs) could not be transferred to third parties owing to patient privacy concerns.
* AF
: Atrial fibrillation
ECG
: Electrocardiography
SR
: Sinus rhythm
更多查看译文
AI 理解论文
溯源树
样例
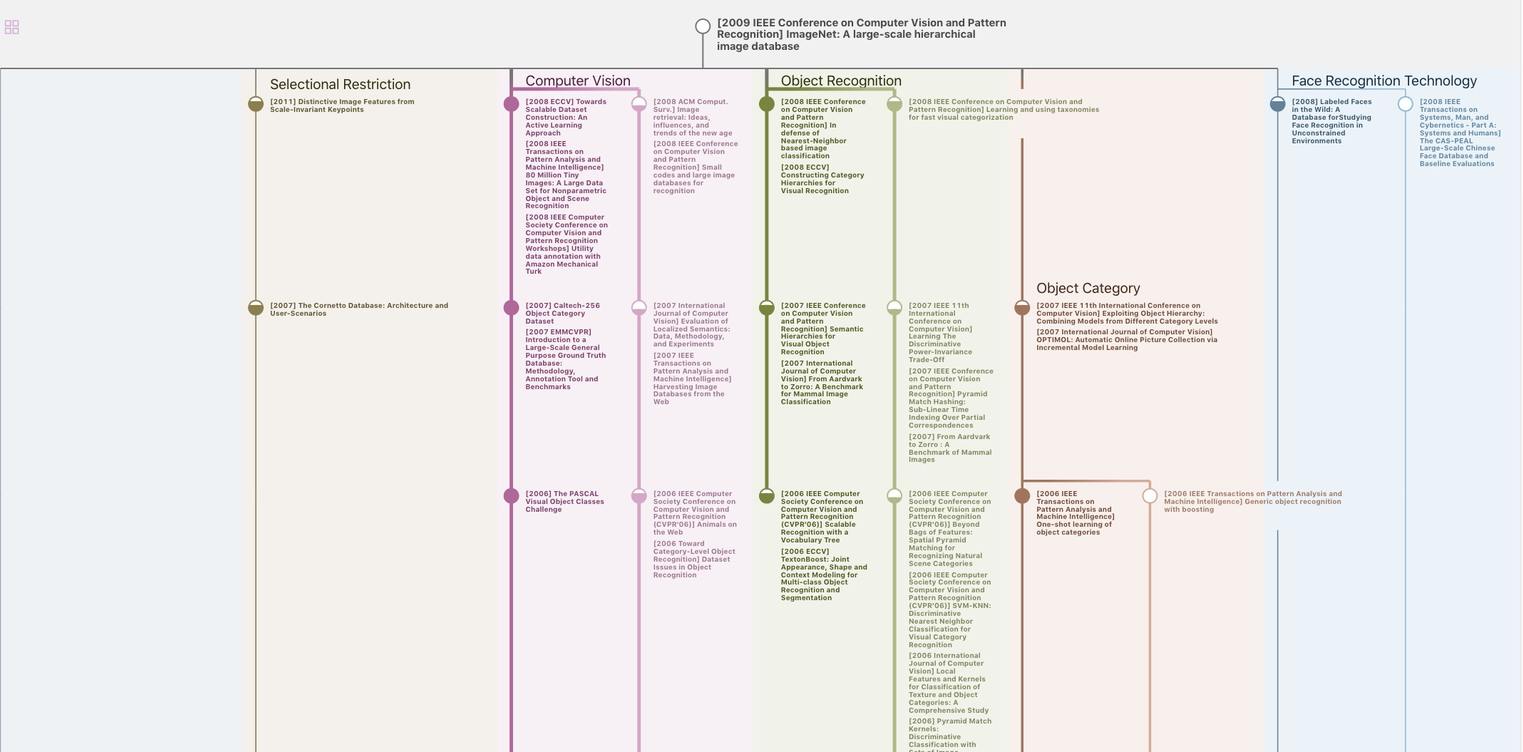
生成溯源树,研究论文发展脉络
Chat Paper
正在生成论文摘要