Learning Well-Separated and Representative Prototypes for Few-Shot Event Detection.
NLPCC (2)(2023)
摘要
Event detection can be solved with two subtasks: identification and classification of trigger words. Depending on whether these two subtasks are handled simultaneously, event detection models are divided into the pipeline-based paradigm and the joint-based paradigm. For both paradigms, prototypical networks are an extremely important method to solve a few-shot event detection task. Prototypical networks classify through the distances between instances and prototypes. Therefore, both the distance between prototypes and prototype representations are the key to prototypical networks. Compared with the pipeline-based paradigm, the joint-based paradigm handles two subtasks at the same time to effectively avoid the error cascade. To make better use of prototypical networks in the joint-based paradigm, we propose PN-SR, a P rototypical N etwork with well- S eparated and R epresentative prototypes. Specifically, we use representation learning methods to generate prototypes. At the same time, we add a loss factor to further enlarge the distances between prototypes of different classes and reduce semantic representation distances of instances with the same class. Experiments show our method outperforms many baselines by at least 26.15% F1 points under the same few-shot settings and achieves SOTA.
更多查看译文
关键词
detection,event,representative prototypes,well-separated,few-shot
AI 理解论文
溯源树
样例
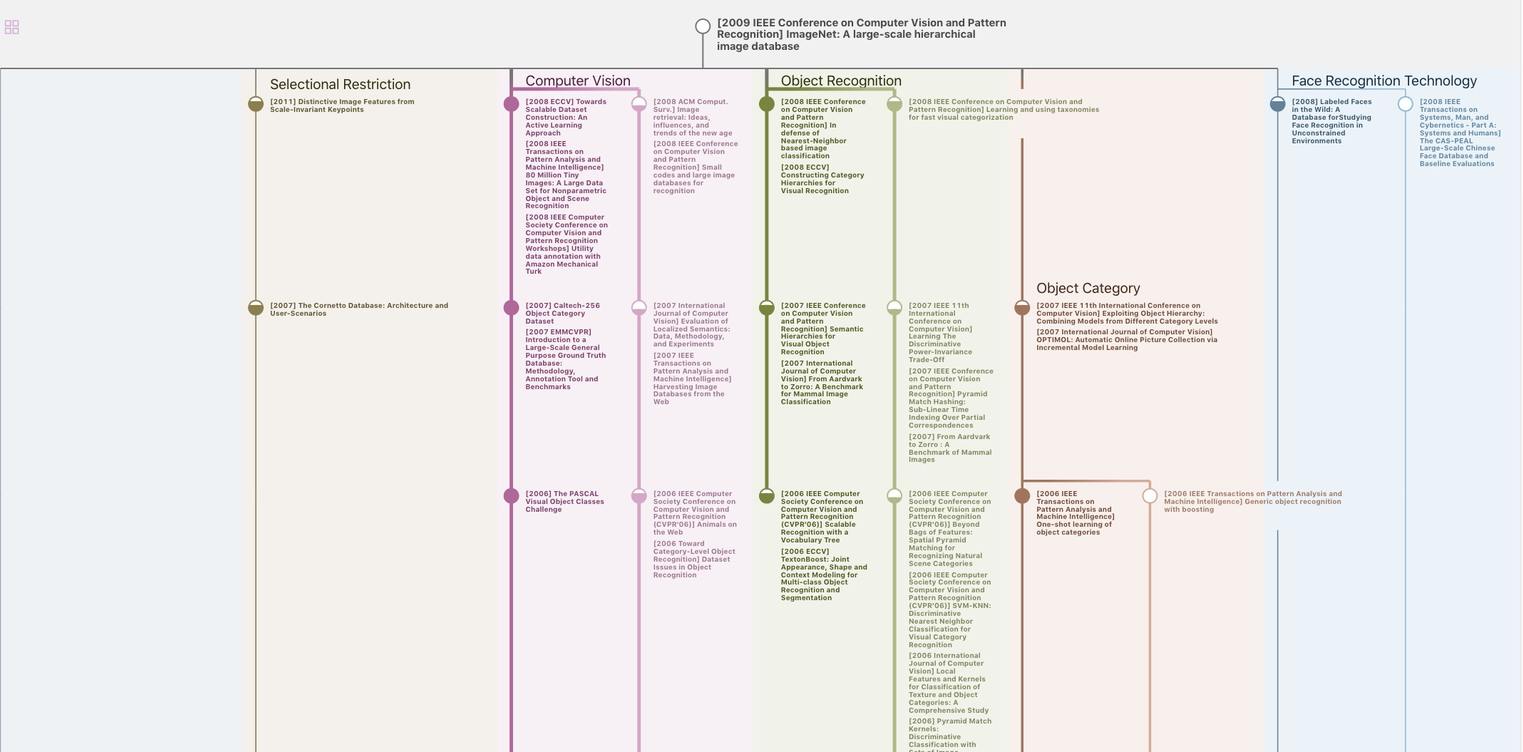
生成溯源树,研究论文发展脉络
Chat Paper
正在生成论文摘要