Fantastic Gradients and Where to Find Them: Improving Multi-attribute Text Style Transfer by Quadratic Program.
NLPCC (3)(2023)
摘要
Unsupervised text style transfer (TST) is an important task with extensive implications in natural language generation (NLG). A prevalent approach involves editing the latent representations of text, guided by gradients from an attribute classifier. However, in multi-attribute TST, the simultaneous satisfaction of all required attributes remains challenging. In this paper, we unveil that the gradient direction during editing might conflict with certain attribute representations through empirical analysis. To tackle this problem, we introduce a mathematical programming method to impose constraints on the editing direction of multiple attributes, effectively mitigating potential attribute conflicts during the inference stage. Our proposed method considers the potential conflict between different attributes for the first time. Experimental results from the YELP benchmark showcase that our method can effectively improve the multi-attribute-transfer accuracy and quality without compromising single attribute performance. Moreover, our method can be readily integrated with pre-trained auto-encoders, providing an effective and scalable solution for multi-attribute scenarios.
更多查看译文
关键词
fantastic gradients,text,quadratic program,multi-attribute
AI 理解论文
溯源树
样例
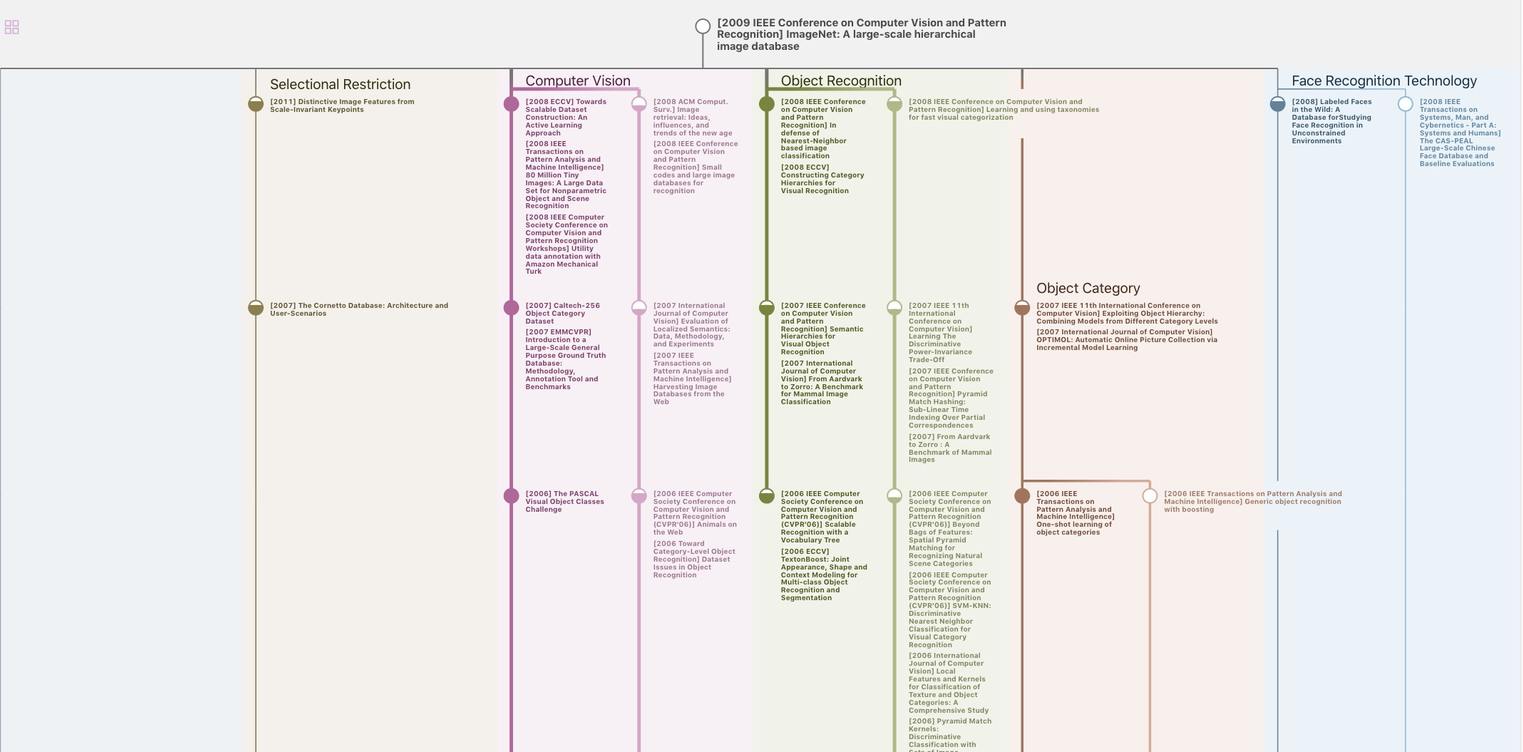
生成溯源树,研究论文发展脉络
Chat Paper
正在生成论文摘要