Enhanced feature extraction-based semantic segmentation network for remote sensing image using modified Swin Transformer.
BDIOT(2023)
摘要
Remote sensing image segmentation is a specialized form of semantic segmentation that presents unique challenges not typically found in general semantic segmentation tasks. The key issues addressed in this study are the highly imbalanced foreground-background distribution and the presence of multiple small objects intertwined in complex backgrounds. However, existing methods heavily rely on convolutional neural networks (CNNs), which, due to their local nature, struggle to effectively capture global context. by the powerful global modeling capability of the Swin Transformer [1], this paper proposes a novel U-shaped network for remote sensing image semantic segmentation called Light Swin Transformer_Unet. In this network, the attention calculation of the Swin Transformer is modified and employed in the encoding part of the network. Additionally, an adaptive multi-level feature pyramid pooling based on CNNs is integrated into the auxiliary decoder of the Unet, creating a novel parallel connection structure with feature processing capabilities. This module effectively addresses the limitations of Transformers in focusing on local features. Experimental results on the Loveda [2] dataset demonstrate that the proposed network outperforms pure CNNs, pure Transformer networks, as well as networks that fuse CNNs and Transformers in other forms. Moreover, the proposed network achieves a slight performance improvement with a decrease in parameter count compared to the Transformer alone.The research findings provide a reference for the fusion network of CNN and Transformer, and offer valuable methods and techniques to address challenges in this field.
更多查看译文
AI 理解论文
溯源树
样例
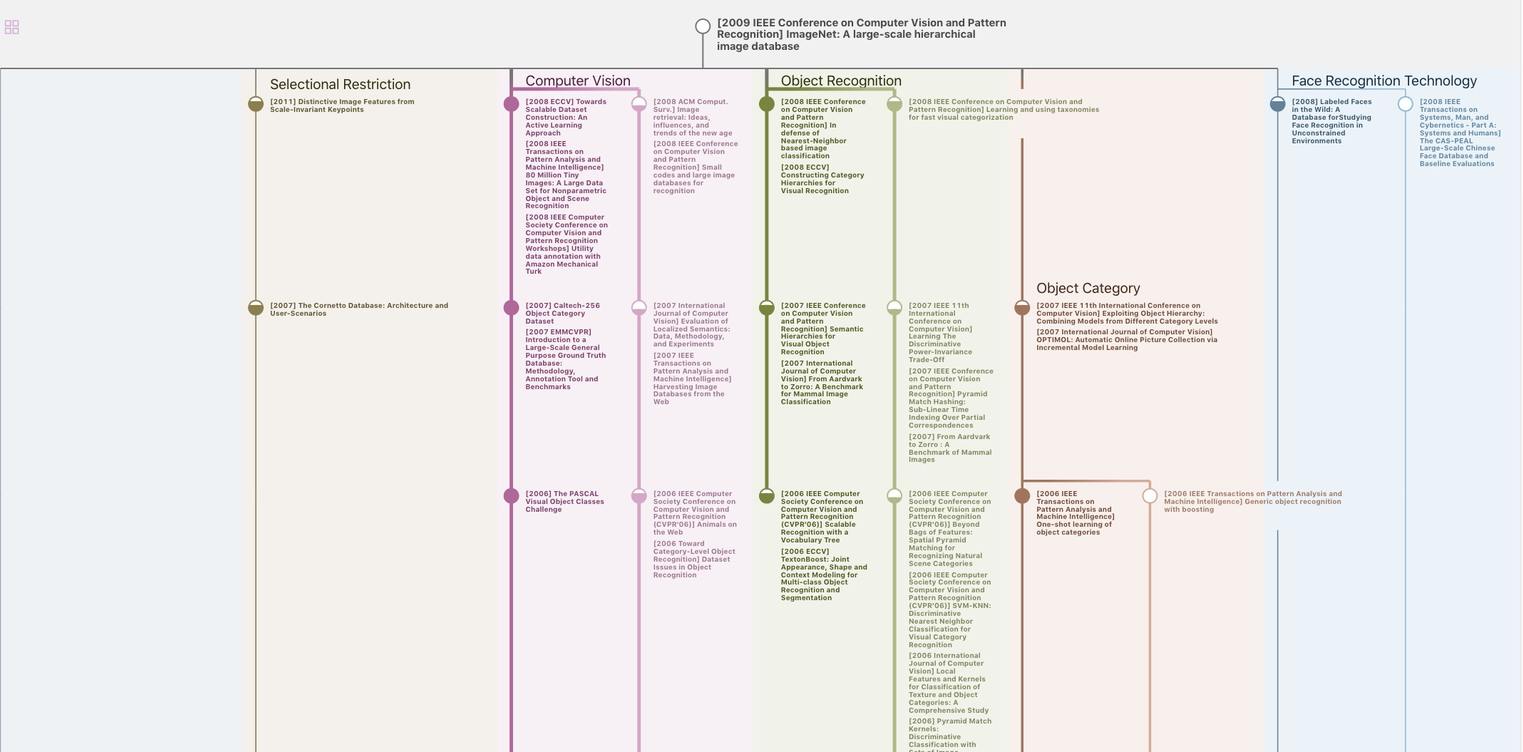
生成溯源树,研究论文发展脉络
Chat Paper
正在生成论文摘要