A Fine-Grained Evaluation of Mutation Operators for Deep Learning Systems: A Selective Mutation Approach.
Internetware(2023)
摘要
The widespread adoption of deep learning (DL) has made it critical to ensure its reliability. Mutation testing has been employed in DL testing to assess test data quality, but it can be costly of a large number of generated mutants. Cost reduction can be achieved by selecting a sufficient subset of mutation operators. However, it remains unclear to what extent the DL mutation operators contribute to test effectiveness, making it challenging to determine which are useful mutation operators in a selective mutation approach. In this paper, we perform a fine-grained evaluation of DL mutation operators by introducing the classification results of DL models. Based on the findings that mutants generated by some mutation operators can be redundant or useless in guiding the generation of high-quality test cases, we introduce two measures of DL mutation operators usefulness: redundancy score (RS), which quantifies the redundancy of operators, and quality score (QS), which quantifies the ability of operators to guide the generation of high-quality test cases. Our empirical evaluation on three widely used datasets and four DL models shows that RS and QS offer a dual evaluation of DL mutation operators. When employing mutation operators in a selective mutation strategy, prioritizing mutation operators based on RS can result in a reduction of mutants by 46.15 to 69.23, while retaining over 90 of mutation score. Similarly, prioritizing mutation operators based on QS can reduce mutants by 46.15 to 50.35, while retaining over 87 of test cases. Our study shows that RS-based and QS-based selective mutation can significantly reduce the number of mutants while maintaining high test effectiveness.
更多查看译文
AI 理解论文
溯源树
样例
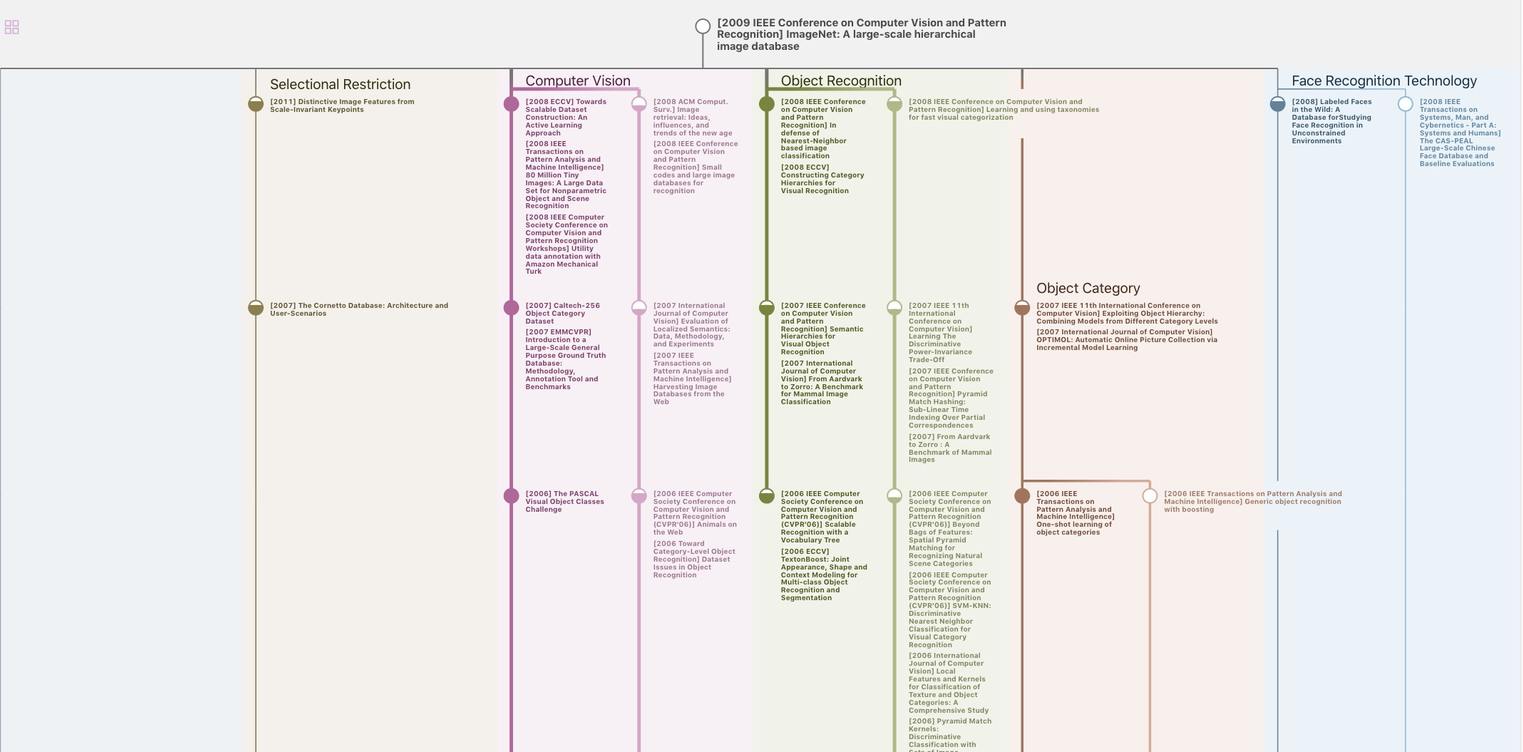
生成溯源树,研究论文发展脉络
Chat Paper
正在生成论文摘要