Towards Better Multilingual Code Search through Cross-Lingual Contrastive Learning.
Internetware(2023)
摘要
Recent advances in deep learning have significantly improved the understanding of source code by leveraging large amounts of open-source software data. Thanks to the larger amount of data, code representation models trained with multilingual datasets show superior performance to monolingual models and attract much more attention. However, the entangled source code from various programming languages makes multilingual models hard to differentiate language-specific textual semantics or syntactic structures, which significantly increases the difficulty of model learning from multilingual datasets directly. On the other hand, for a given problem, developers are likely to choose similar identifiers, even if coding in different languages. However, the presence of similar identifiers in multilingual code snippets does not mean that they implement the same functionality, which may misdirect models to overemphasize these unreliable signals and ignore the semantic information of multilingual code. To tackle the above issues, we propose LAMCode, a language-aware multilingual code understanding model. Specifically, we propose a simple yet effective method to perceive linguistic information by injecting language-specific viewer into the language models. Furthermore, we introduce a cross-lingual contrastive learning method by generating more similar training instances but with fewer overlapping features. This method prevents the models from over-relying on similar identifiers across languages. We conduct extensive experiments to evaluate the effectiveness of our approach on a large-scale multilingual dataset. The experimental results show that our approach significantly outperforms the state-of-the-art methods.
更多查看译文
AI 理解论文
溯源树
样例
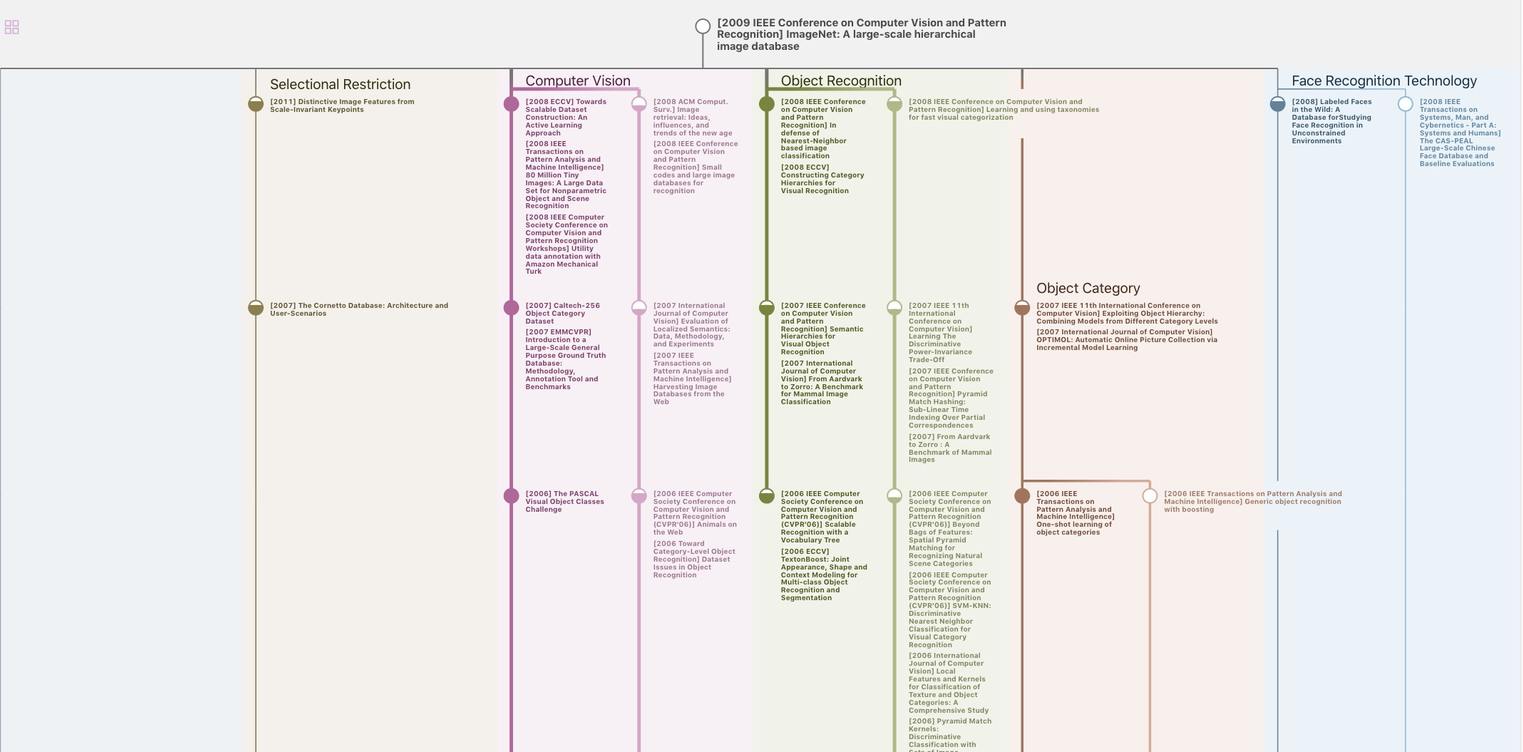
生成溯源树,研究论文发展脉络
Chat Paper
正在生成论文摘要