Embedding Transfer with Enhanced Correlation Modeling for Cross-Domain Recommendation.
SDM(2023)
摘要
Modern internet platforms usually have different scenarios to provide rich recommendation services to meet the diverse demands of users. Cross-domain recommendation (CDR) and multi-domain recommendation (MDR) methods are widely used in such platforms to leverage rich auxiliary information from multiple domains. However, state-of-the-art CDR and MDR methods usually enforce some correlations between source and target embeddings on each user, ignoring the correlations between users in both domains. To address this problem, we adopt a relaxed contrastive loss, that employs the pairwise similarities in the source domain as relaxed labels, enforcing such inter-sample relations are reserved in a weighted manner in the target domain. The basic assumption behind such a design is that users with similar interests should be with similar interacted items in a rec- ommender system, and this work takes a step further to realize and specify such similarity modeling as collaborative signals encoded in both implicit embedding spaces. We validate the effectiveness of the proposed method on a large- scale public dataset and a real production dataset with over 700 million samples. We further experimentally show that the proposed embedding transfer method is generic, and can be plugged into any existing deep neural networks, such as YoutubeDNN and BERT4Rec. Currently, the proposed embedding transfer techniques have been successfully deployed in the Guess You Like in WeTV for the CDR/MDR task.
更多查看译文
关键词
enhanced correlation modeling,transfer,cross-domain
AI 理解论文
溯源树
样例
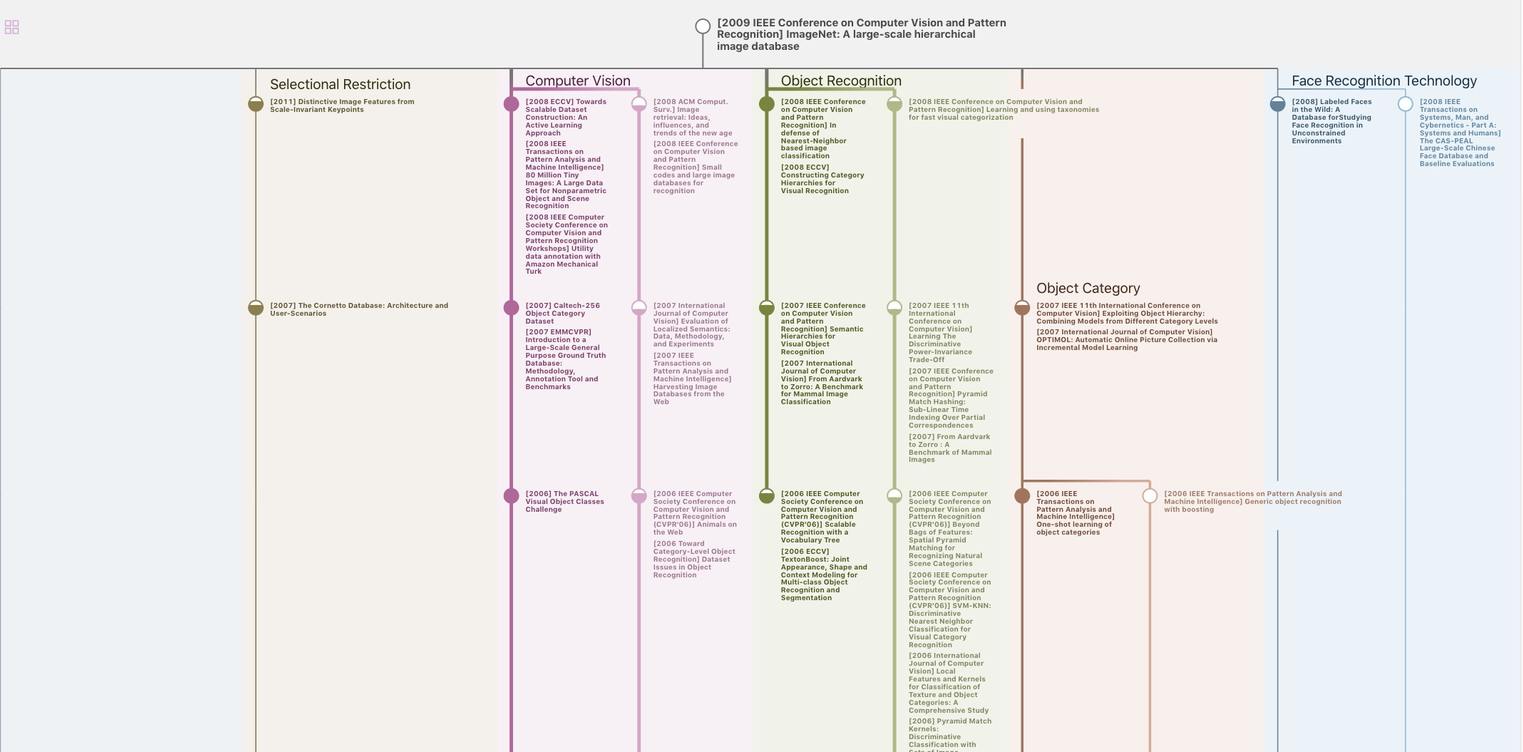
生成溯源树,研究论文发展脉络
Chat Paper
正在生成论文摘要