MC-SQ: A Highly Accurate Ensemble for Multi-class Quantification.
SDM(2023)
摘要
Quantification research proposes methods to estimate the class distribution in an independent sample. Many areas, such as epidemiology, sentiment analysis, political research and ecological surveillance, rely on quantification methods to estimate aggregated quantities. For instance, epidemiologists are often concerned with the dynamics of the number of disease cases across space and time. Thus, while classification predicts individual subjects, quantification is the class of methods that directly estimate the number of cases. Quantification is a thriving research area, and the community has proposed several approaches in the last decade. Nevertheless, most quantification research has focused on binary-class quantifiers, expecting these approaches to extend to multi-class using the one-versus-all (OVA) approach. However, there is enough empirical evidence indicating the performance of OVA multi-class quantifiers is subpar. This paper has two main contributions. First, we demonstrate why OVA quantifiers are doomed to underperform in multi- class settings due to a distribution shift they cannot handle. Second, we propose a new class of quantifiers based on ensemble learning that boosts the performance of the base quantifiers in the binary and, more importantly, multi-class settings. In one of the most comprehensive experimental setups ever attempted in quantification research, we show that our ensembles are the best-performing quantifiers compared with 33 state-of-the-art (single and ensemble) quantifiers and rank first in a recent quantification competition.
更多查看译文
关键词
accurate ensemble,multi-class
AI 理解论文
溯源树
样例
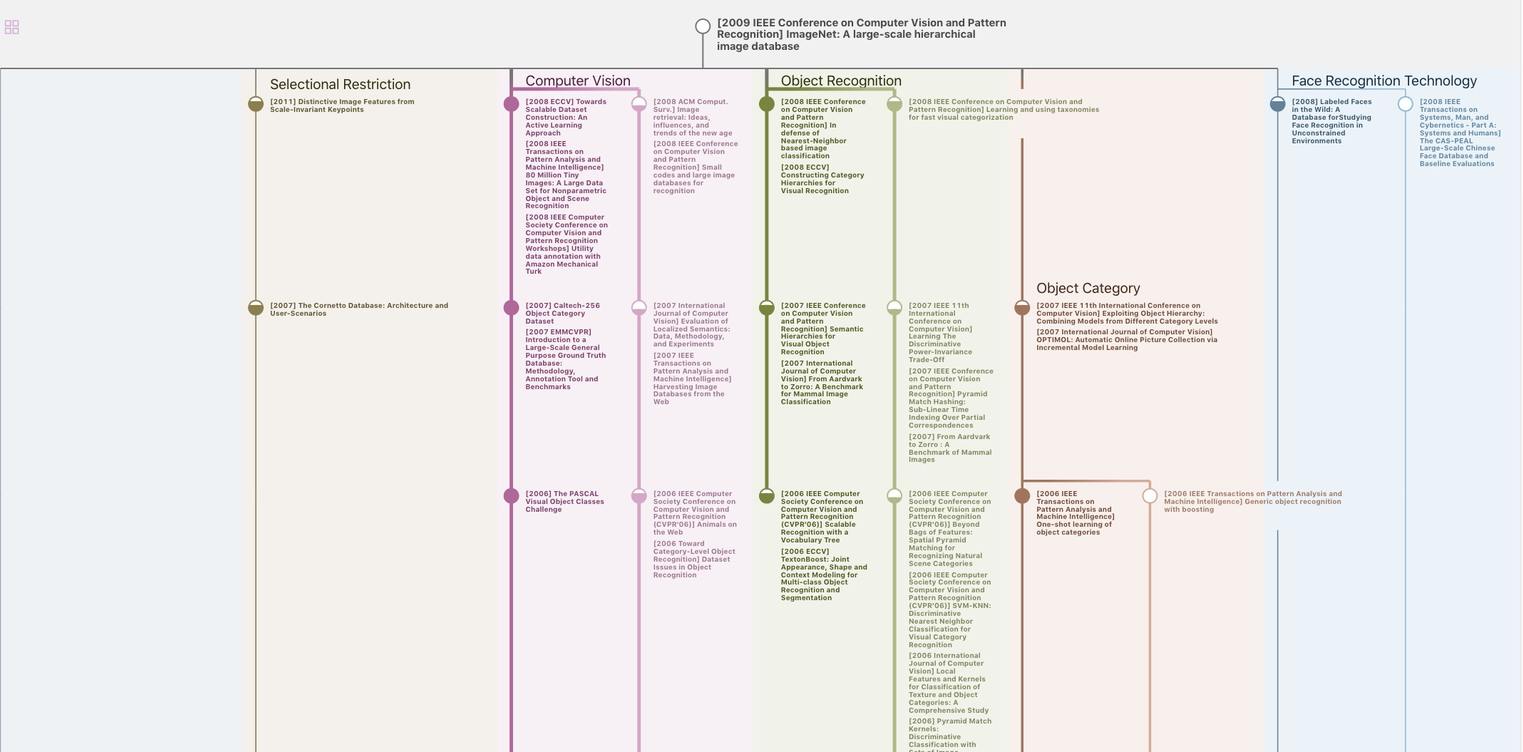
生成溯源树,研究论文发展脉络
Chat Paper
正在生成论文摘要