Positive Definite Wasserstein Graph Kernel for Brain Disease Diagnosis
MEDICAL IMAGE COMPUTING AND COMPUTER ASSISTED INTERVENTION, MICCAI 2023, PT V(2023)
摘要
In brain functional networks, nodes represent brain regions while edges symbolize the functional connections that enable the transfer of information between brain regions. However, measuring the transportation cost of information transfer between brain regions is a challenge for most existing methods in brain network analysis. To address this problem, we propose a graph sliced Wasserstein distance to measure the cost of transporting information between brain regions in a brain functional network. Building upon the graph sliced Wasserstein distance, we propose a new graph kernel called sliced Wasserstein graph kernel to measure the similarity of brain functional networks. Compared to existing graph methods, including graph kernels and graph neural networks, our proposed sliced Wasserstein graph kernel is positive definite and a faster method for comparing brain functional networks. To evaluate the effectiveness of our proposed method, we conducted classification experiments on functional magnetic resonance imaging data of brain diseases. Our experimental results demonstrate that our method can significantly improve classification accuracy and computational speed compared to state-of-the-art graph methods for classifying brain diseases.
更多查看译文
关键词
Graph kernel,Brain functional network,Brain diseases,Classification,Wasserstein distance
AI 理解论文
溯源树
样例
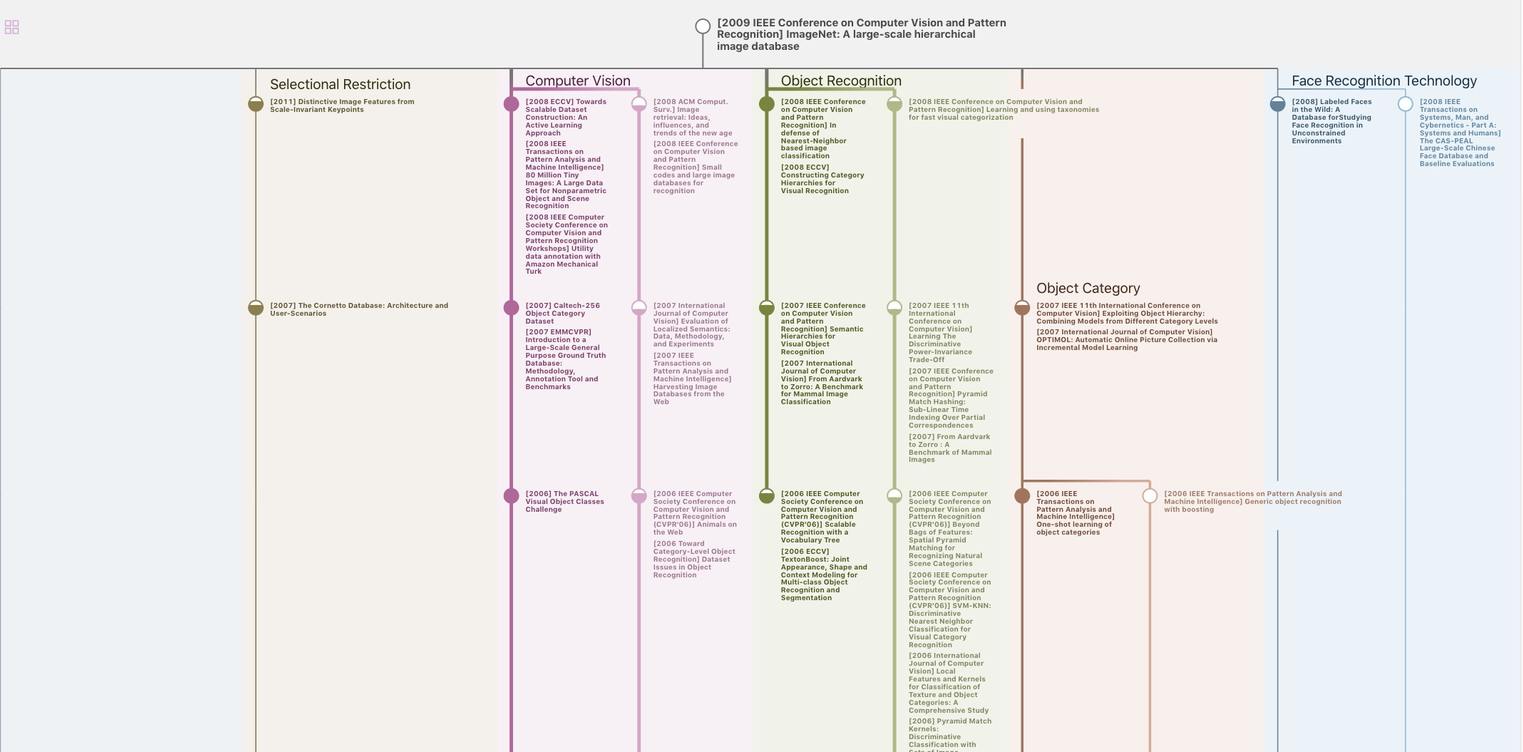
生成溯源树,研究论文发展脉络
Chat Paper
正在生成论文摘要