Hybrid Shot2Shot and Re-De-Noising Regularization for Random Noise Attenuation of Seismic Data
IEEE Geosci. Remote. Sens. Lett.(2023)
摘要
Random noise attenuation is essential in seismic data processing. In this letter, we propose an unsupervised method called "shot2shot (S2S) with re-de-noising regularization" to remove random noise. S2S is a new way to train a denoising neural network. S2S takes a shot-gather and its multiple neighboring shot-gathers as input and labels of the neural network, respectively. The principle that S2S can eliminate noise is the correlation of seismic waves and the independence of random noise between neighboring shot-gathers. Because neural networks are more likely to learn correlated information between inputs and labels rather than independent information. Although S2S is effective in denoising, this mode of training may lead to relatively coarse results. Therefore, we propose re-de-noising regularization to make the results of S2S more refined. The re-de-noising regularization consists of two penalty terms that balance each other, the re-de-noising term and the stability term. The stability term is responsible for introducing more fine content from the observations, such as weak waves, but this can introduce new noise. Thus the re-de-noising term is used to avoid the interference of this new noise. Experimentally, our method outperforms other state-of-the-art methods in terms of quantitative results. Visually, our method not only removes the noise but also reconstructs the noisy data more completely. In addition, we explain the role of S2S and re-de-noising regularization more intuitively through ablation experiments. Finally, the robustness of the key hyperparameters is discussed.
更多查看译文
关键词
Neural networks,Noise reduction,Attenuation,Training,Image reconstruction,Noise measurement,Geoscience and remote sensing,Random noise attenuation,re-de-noising regularization,seismic data,shot2shot (S2S),unsupervised learning
AI 理解论文
溯源树
样例
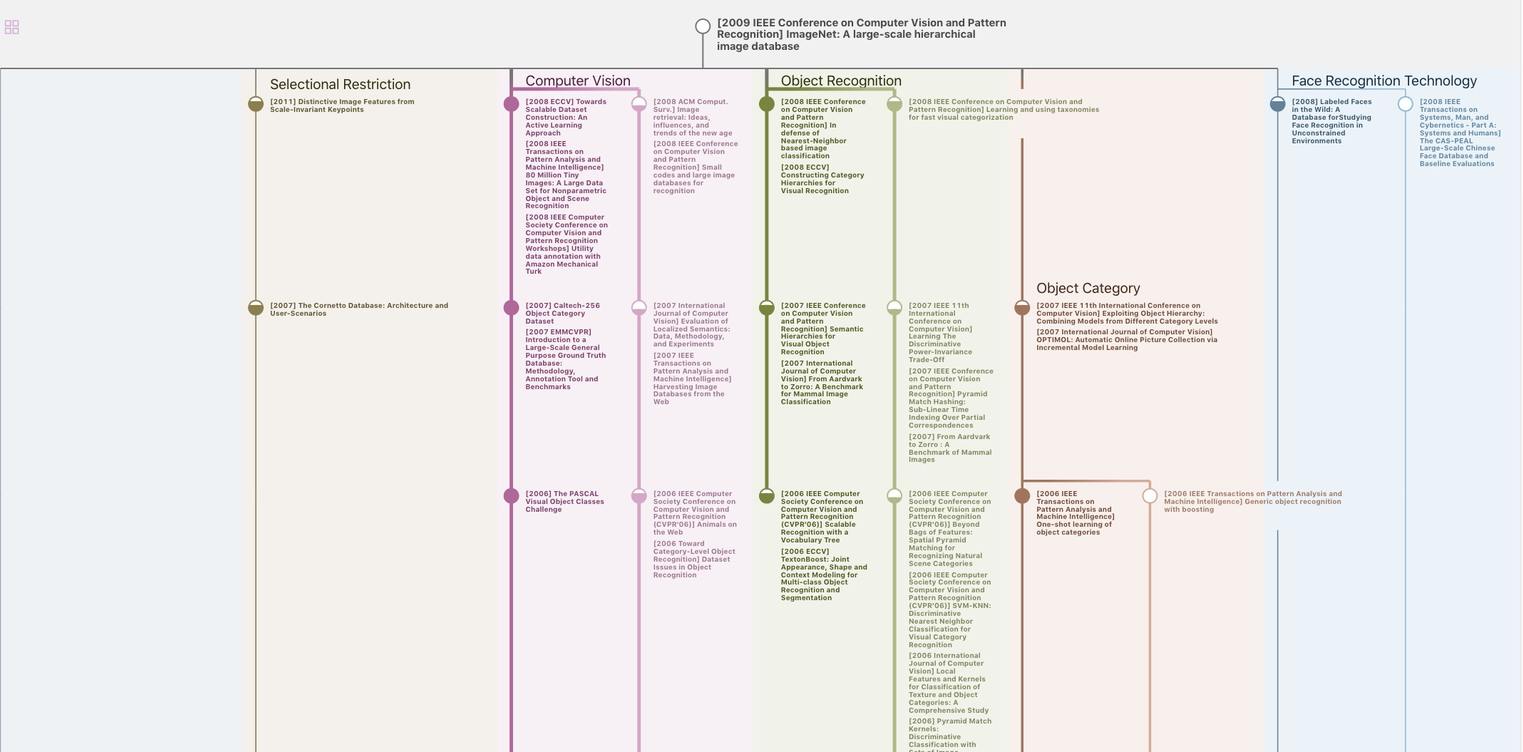
生成溯源树,研究论文发展脉络
Chat Paper
正在生成论文摘要