ScoreSeg: Leveraging Score-Based Generative Model for Self-Supervised Semantic Segmentation of Remote Sensing
IEEE J. Sel. Top. Appl. Earth Obs. Remote. Sens.(2023)
摘要
The performance of semantic segmentation of remote sensing images (RSIs) heavily depends on the number of pixel-level annotations. In practice, the accumulation of pixel-level annotations for large RSIs is quite expensive or even impossible under certain scenarios. Here, we try to solve this data-intensive problem from the novel aspect of score-based self-supervise learning (SSL) and introduce a robust RSI semantic segmentation model called ScoreSeg. Unlike traditional pixel-level SSL paradigms, the generative SSL mechanism in ScoreSeg is simple in loss design and stable in pretraining, granting it an indispensable ability in dense feature learning from very large RSIs. In the model implementation, ScoreSeg first extracts pixelwise representations of RSIs by pretraining a time-dependent score-based model on abundant off-the-shelf unlabeled RSIs. Then, to address the sparse feature problem in RSIs, the collected features from different timesteps and resolutions are aggregated together forming a rich feature map for downstream semantic segmentation. Experimental results on three datasets show that our proposed ScoreSeg outperforms state-of-the-art (SOTA) SSL methods and alternative pretraining models on ImageNet by nontrivial margins, especially with very limited annotations.
更多查看译文
关键词
Remote sensing,score-based generative model,self-supervised learning,semantic segmentation
AI 理解论文
溯源树
样例
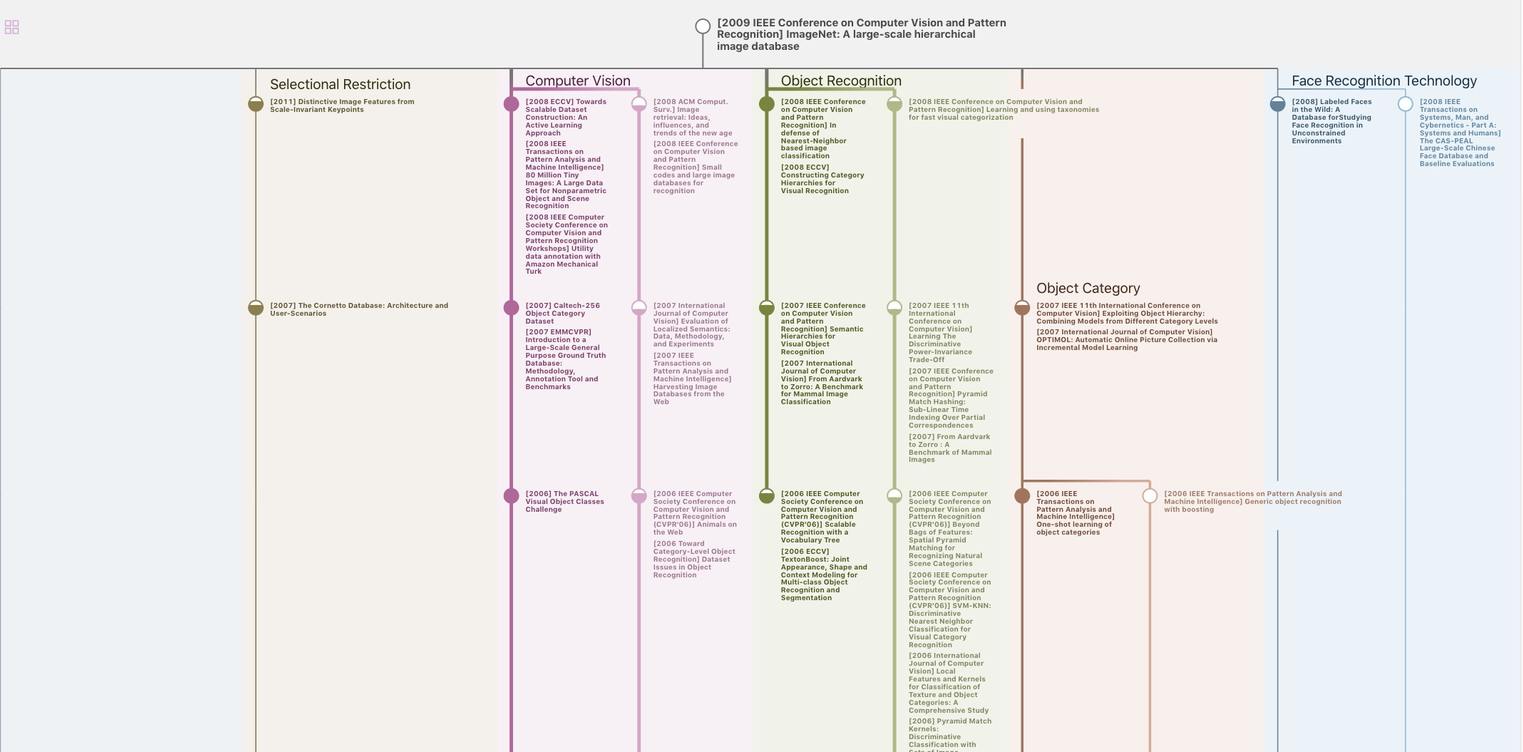
生成溯源树,研究论文发展脉络
Chat Paper
正在生成论文摘要