Differentially Private Denoise Diffusion Probability Models.
IEEE Access(2023)
摘要
Diffusion models and their variants have achieved high-quality image generation without adversarial training. These algorithms provide new ideas for data shortages in some fields. But the diffusion model also faces the same problem as other generative models: the learned probability density function will retain the characteristics of the training samples, which means that the high complexity of the deep network will make the model easily remember the training samples. When a diffusion model is applied to sensitive datasets, the distribution the model focuses on may reveal private information, and the security concerns described above become more pronounced. To address this challenge, this paper proposes a privacy diffusion model named DPDM (Differentially Private Denoise Diffusion Probability Models) that satisfies differential privacy by adding appropriate noise to the gradient during the training. Besides, this paper adopts a series of optimization strategies to improve model performance and training speed such as adaptive gradient clipping threshold and dynamic decay learning rate. Through the evaluation and analysis of the benchmark dataset, it is found that the attempt in this paper has promising usability, and the synthetic data has better performance.
更多查看译文
关键词
Data shortage,generate model,diffusion model,differential privacy
AI 理解论文
溯源树
样例
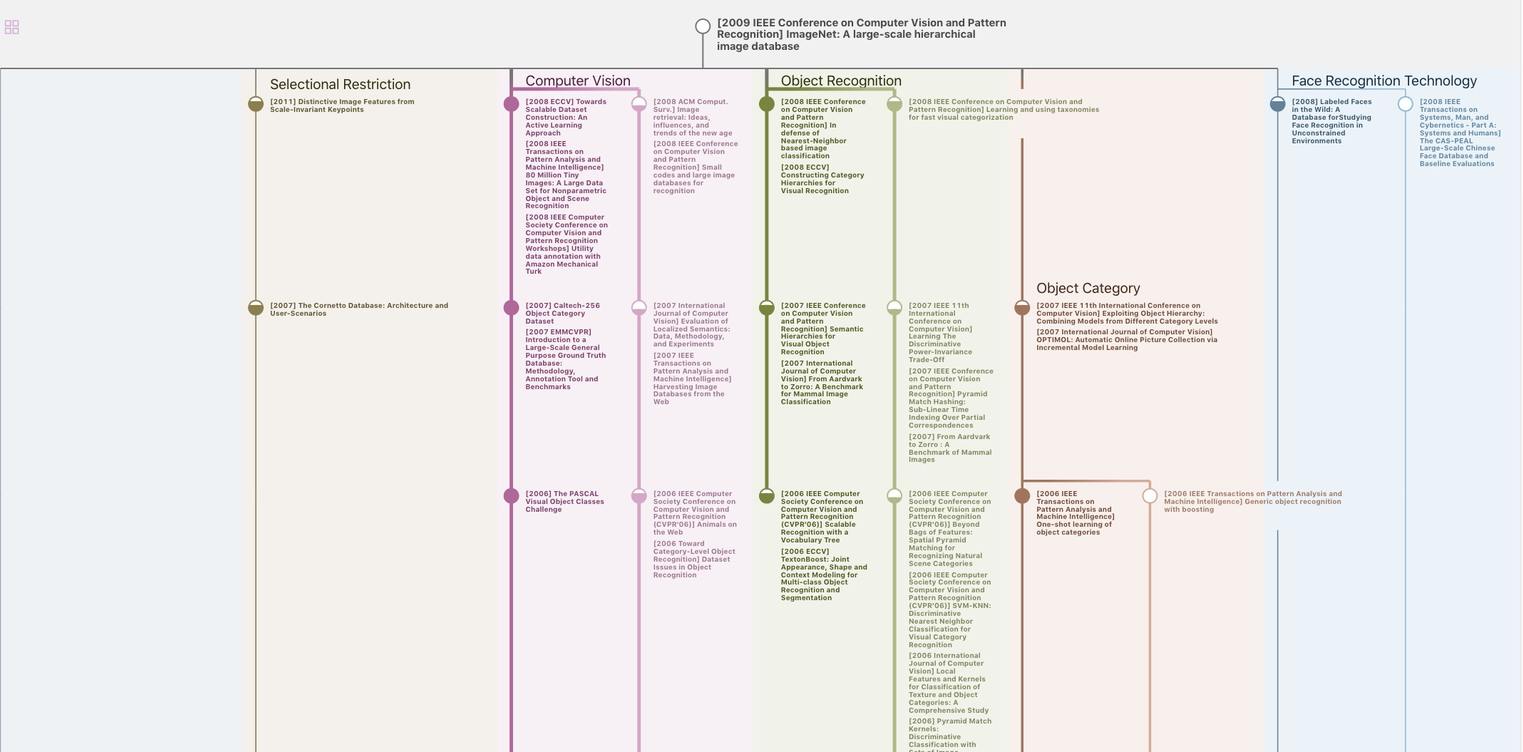
生成溯源树,研究论文发展脉络
Chat Paper
正在生成论文摘要