Anomaly Detection in Coastal Wireless Sensors via Efficient Deep Sequential Learning.
IEEE Access(2023)
摘要
Wireless Sensor Networks (WSNs) encounter a substantial challenge when it comes to energy conservation. As sensor nodes rely on battery power to operate in unattended environments, and it can be inconvenient to recharge the batteries. So, maximizing the network's operational lifetime by minimizing energy consumption is crucial for improving the quality of service. Anomaly detection is widely used technique in WSNs to identify anomalies or unusual events. However, timely anomaly detection can be challenging to execute reliably in real-time. This study presents a methodology that focuses on energy efficiency when detecting anomalies in real-time settings for multivariate time series data by utilizing compression of measurement data and reducing operating time without compromising measurement resolution. This effectively reduces energy consumption. Instead of modeling the time series of each sensor individually, the proposed deep sequential learning approach models the time series of multiple sensors concurrently, accounting for potential interactions among them. Additionally, the proposed deep sequential learning approach eliminates the need for labeled data and can be directly applied to real-world scenarios where labeling a large data stream is impractical and time-consuming. Finally, experiments with a real-world WSN demonstrate that the proposed approach is both adequate and robust in practice.
更多查看译文
关键词
Wireless sensor network (WSN), multivariate time series, energy conservation, anomaly detection, data compression, deep learning, model quantization
AI 理解论文
溯源树
样例
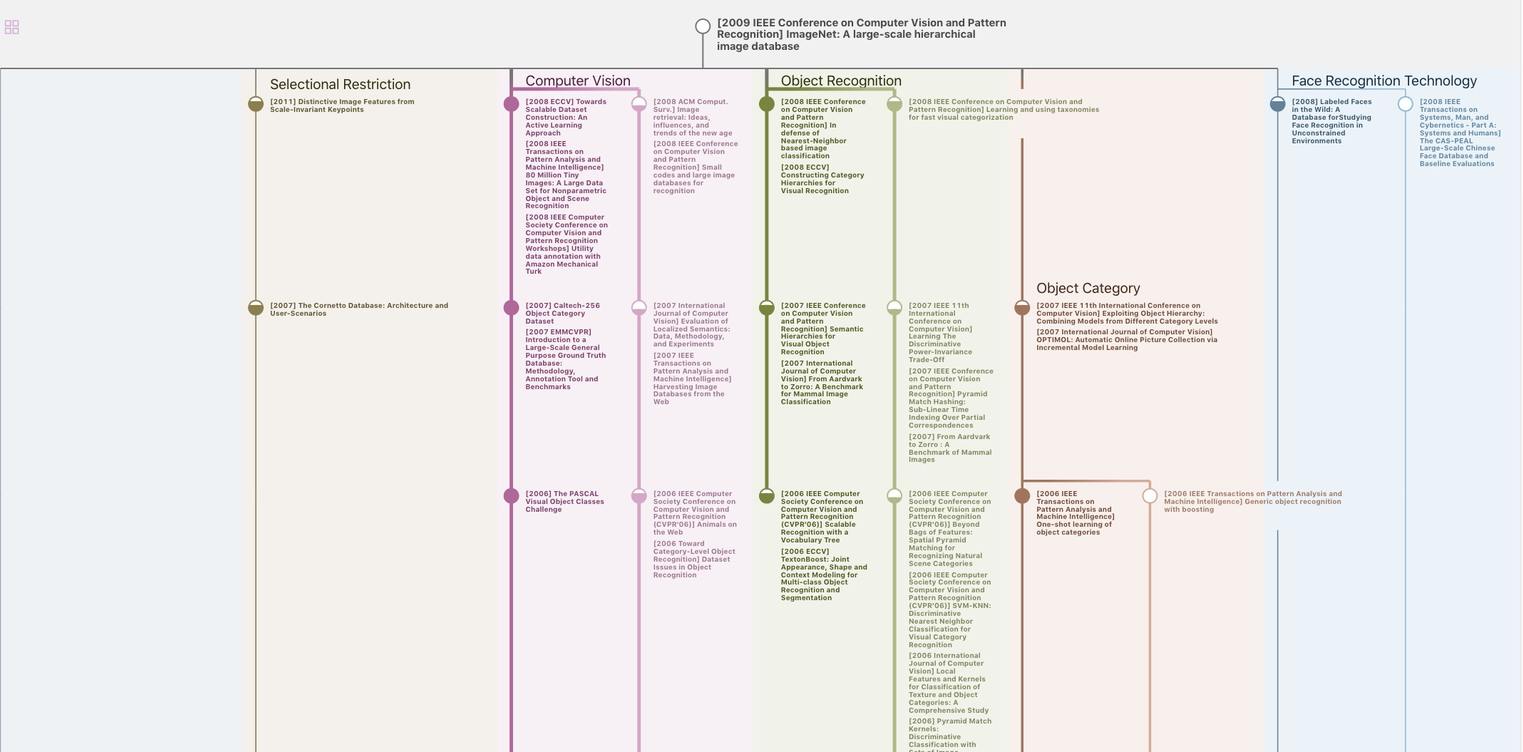
生成溯源树,研究论文发展脉络
Chat Paper
正在生成论文摘要