Feature fine-tuning and attribute representation transformation for zero-shot learning
Comput. Vis. Image Underst.(2023)
摘要
Zero-Shot Learning (ZSL) aims to generalize a pretrained classification model to unseen classes with the help of auxiliary semantic information. Recent generative methods are based on the paradigm of synthesizing unseen visual data from class attributes. A mapping is learnt from semantic attributes to visual features extracted by a pre-trained backbone such as ResNet101 by training a generative adversarial network. Considering the domain-shift problem between pre-trained backbone and task ZSL dataset as well as the information asymmetry problem between images and attributes, this manuscript suggests that the visual-semantic balance should be learnt separately from the ZSL models. In particular, we propose a plug-and-play Attribute Representation Transformation (ART) framework to pre-process visual features with a contrastive regression module and an attribute place-holder module. Our contrastive regression loss is a tailored design for visual-attribute transformation, which gains favorable properties from both classification and regression losses. As for the attribute place-holder module, an end-to-end mapping loss function is introduced to build the relationship between transformed features and semantic attributes. Experiments conducted on five popular benchmarks manifest that the proposed ART framework can significantly benefit existing generative models in both ZSL and generalized ZSL settings.
更多查看译文
关键词
Generalized zero-shot learning,Generative adversarial networks,Data distribution,Information asymmetric problem
AI 理解论文
溯源树
样例
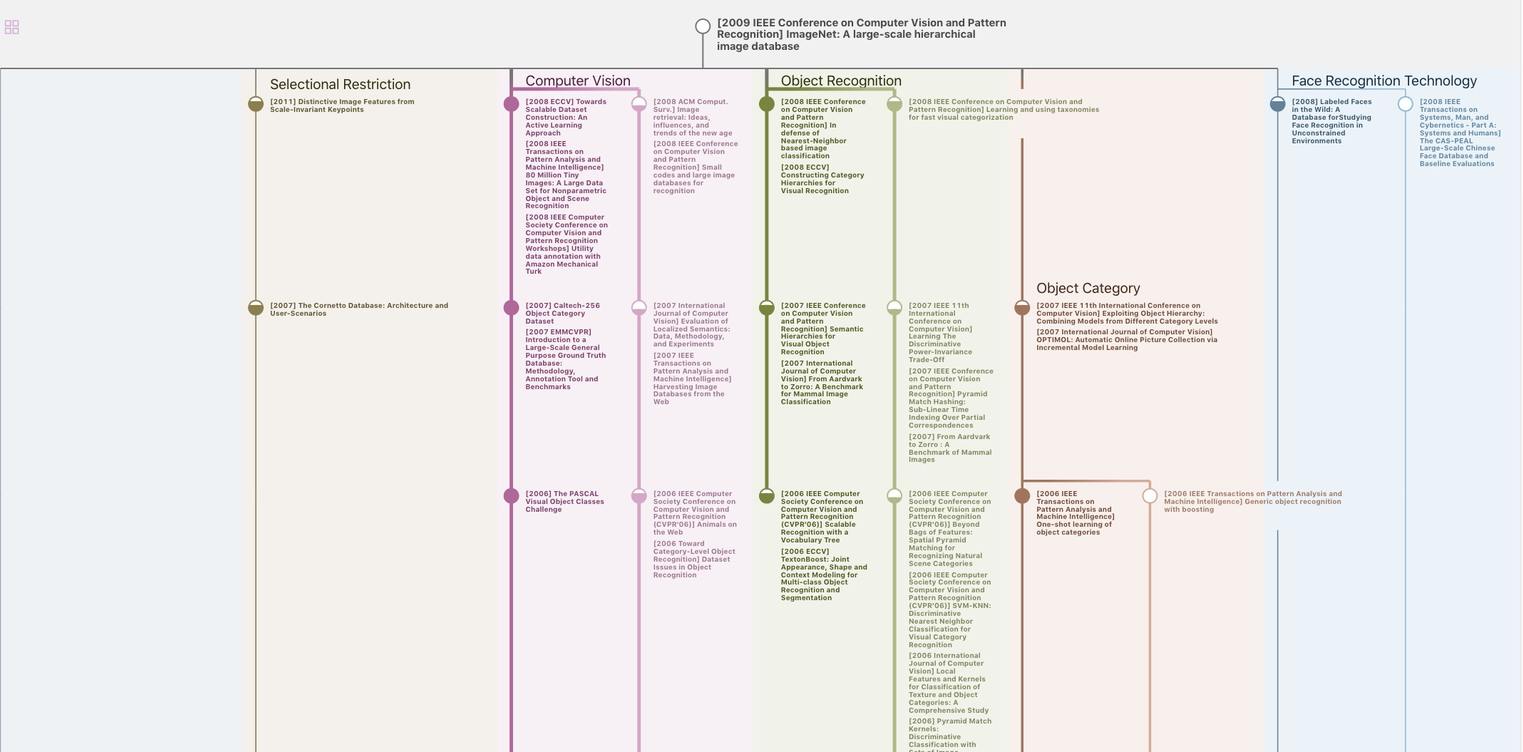
生成溯源树,研究论文发展脉络
Chat Paper
正在生成论文摘要