Gender Bias Analysis in Commonsense Knowledge Graph Embeddings
2023 15th International Conference on Knowledge and Systems Engineering (KSE)(2023)
摘要
In this study, we explore the existence of gender bias in ConceptNet triples by employing knowledge graph embedding models, a crucial aspect that has gained prominence in the artificial intelligence domain. Commonsense knowledge graphs, such as ConceptNet, play a vital role in an array of natural language processing tasks; nonetheless, the potential gender bias embedded within these graphs may considerably influence the fairness of associated applications. To tackle this concern, we introduce a comprehensive gender bias analysis framework tailored for ConceptNet triples, with a focus on the link prediction task utilizing knowledge graph embedding models. By evaluating multiple state-of-the-art knowledge graph embedding models, we assess the degree of inherent gender bias across the models. Furthermore, we investigate mitigation strategies to attain a debiased representation of gender within commonsense knowledge graphs. Our results contribute to a broader comprehension of gender bias in knowledge graphs, and the proposed mitigation strategies lay the groundwork for developing more equitable AI systems that use ConceptNet.
更多查看译文
关键词
Gender Bias,Commonsense Knowledge Graphs,Knowledge Graph Embedding Models
AI 理解论文
溯源树
样例
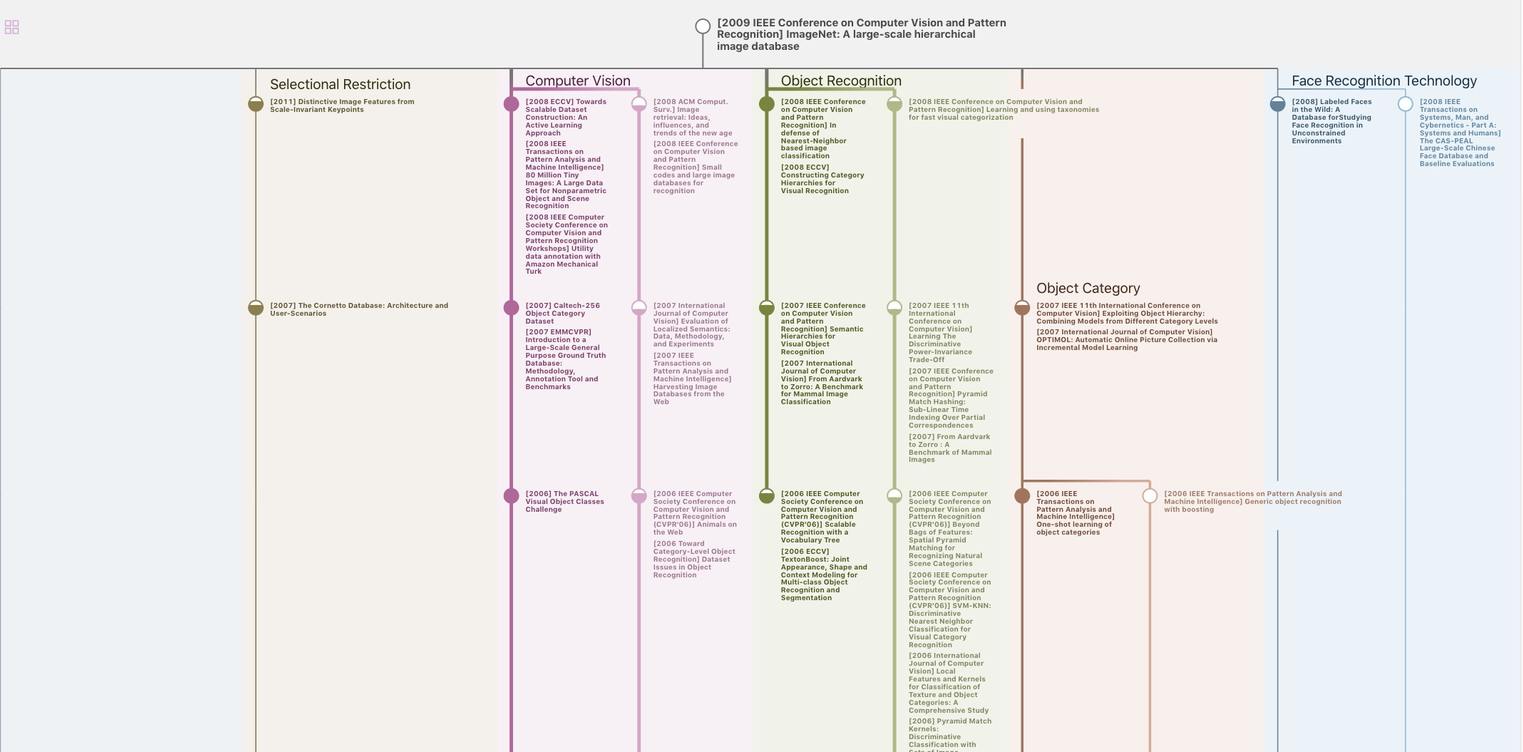
生成溯源树,研究论文发展脉络
Chat Paper
正在生成论文摘要