Enhancing Federated Learning by One-Shot Transferring of Intermediate Features from Clients
2023 IEEE 10th International Conference on Data Science and Advanced Analytics (DSAA)(2023)
摘要
Federated learning (FL) is an emerging paradigm using a parameter server (PS) to coordinate multiple decentralized clients for training a common model without exposing their raw data. Despite its amazing capability in preserving data privacy, FL confronts two significant challenges that have not been sufficiently addressed by existing works, which are: 1) heterogeneous data distributed on clients given that the PS cannot alter data locations. 2) limited computation resources of FL clients who may conduct model training with mobile devices. To tackle these challenges, we propose a novel federated one-shot transferring of intermediate features (FedOTF) algorithm. More specifically, FedOTF consists of two stages: feature extraction and model reconstruction. To overcome challenge 1, clients in stage 1 only collaboratively train a small model in a federated manner, with the objective to extract features. In stage 2, intermediate features (generated by the small model trained by stage 1) are transferred from clients to the PS so that a large model can be trained to overcome challenge 2. In addition, the design of FedOTF is robust, which can flexibly diminish the number of communication rounds of stage 1 when network capacity is limited, and reduce the amount of exposed features when privacy is concerned. To verify the superiority of FedOTF, we conduct comprehensive experiments with real datasets. The experiment results demonstrate that FedOTF can significantly improve the model utility of FL because a better model can be finally obtained by the PS without incurring heavy computational load on clients. Besides, we conduct robustness evaluation of FedOTF which can achieve stable performance when varying network capacity and privacy requirement.
更多查看译文
关键词
Federated Learning,Intermediate Feature,Data Heterogeneity,Limited Computation Resource
AI 理解论文
溯源树
样例
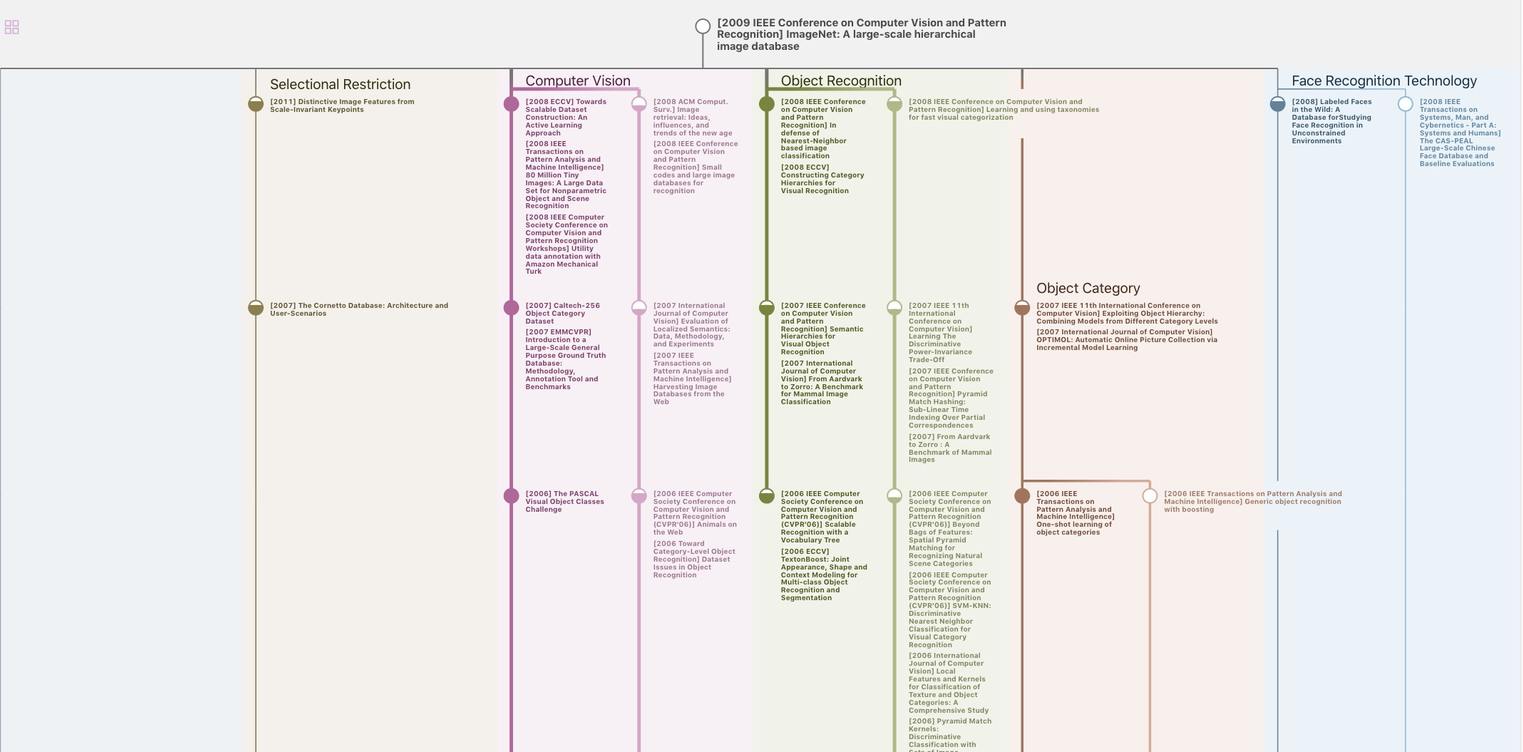
生成溯源树,研究论文发展脉络
Chat Paper
正在生成论文摘要