slidSHAPs – sliding Shapley Values for correlation-based change detection in time series
2023 IEEE 10th International Conference on Data Science and Advanced Analytics (DSAA)(2023)
摘要
For volatile multivariate time series, variations in the distributions of the input dimension and the correlation structure present an open challenge. The different distributions before and after a change-point hinder the performance of most of the predictive methods, mostly requiring re-training of the models. The detection of such change points represents a severe problem, as volatile data labeling is often either expensive or delayed in streaming data; Moreover, classical concept drift detectors usually struggle with detecting changes in correlations of multivariate time series’ input variables. We focus on unsupervised change detection, tracking correlation changes in the input variables without class labels. By introducing slidSHAPs, we propose a fully unsupervised change detector for multivariate time series with categorical value domains; our tool detects correlation-based changes through a representation of the correlation structure of the input data. The slidSHAPs series underlines distributional changes even in a few univariate input variables, thus, being more sensitive to changes than any prior change point detection method. In contrast to the well-known application of Shapley values for interpretable machine learning, we use this foundational game-theoretic concept to extrapolate information on the correlation structure of data streams and achieve higher sensitivity towards multiple changes in the empirical evaluation of synthetic and real-world data.
更多查看译文
关键词
Unsupervised change detection,correlation structure,Shapley values,multivariate time series
AI 理解论文
溯源树
样例
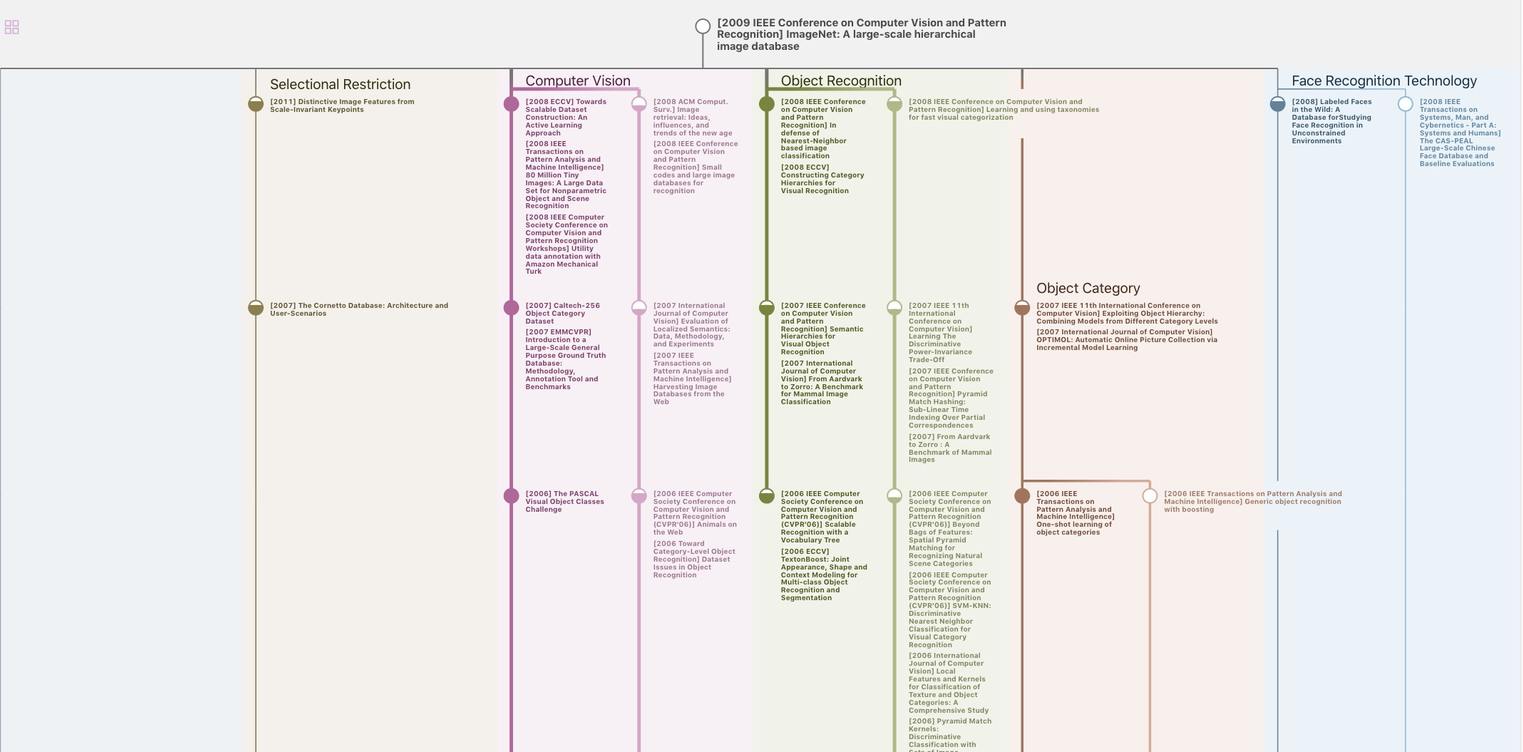
生成溯源树,研究论文发展脉络
Chat Paper
正在生成论文摘要