Wireless Model Splitting for Communication-Efficient Personalized Federated Learning with Pipeline Parallelism
2023 IEEE 24th International Workshop on Signal Processing Advances in Wireless Communications (SPAWC)(2023)
摘要
A wireless federated learning (WFL) system has limited bandwidth and computational power that confines the scale of a supervised learning model. To improve the scalability of the WFL model, a splitting approach has been used to partition the entire model into several sub-models and to assign the sub-models to the server and workers in the WFL system. The previous splitting approaches require to maintain a sub-model for each worker at the server (i.e., parallel splitting) or to sequentially pass the local training information of workers through the sub-model of the server (i.e., sequential splitting). However, when the number of workers is large, the parallel and sequential splitting respectively consume a significant amount of memory and training duration. In this work, we propose a split representation learning (SplitREP) that allows the server and the workers to respectively own the public and private sub-models. Compared with the parallel and sequential splitting, the SplitREP leverages the pipeline parallelism mechanism to reduce the required memory and training duration. Numerical results show that the proposed SplitREP outperforms the benchmarks in the WFL system.
更多查看译文
关键词
Personalized federated learning,pipeline parallelism,wireless model splitting
AI 理解论文
溯源树
样例
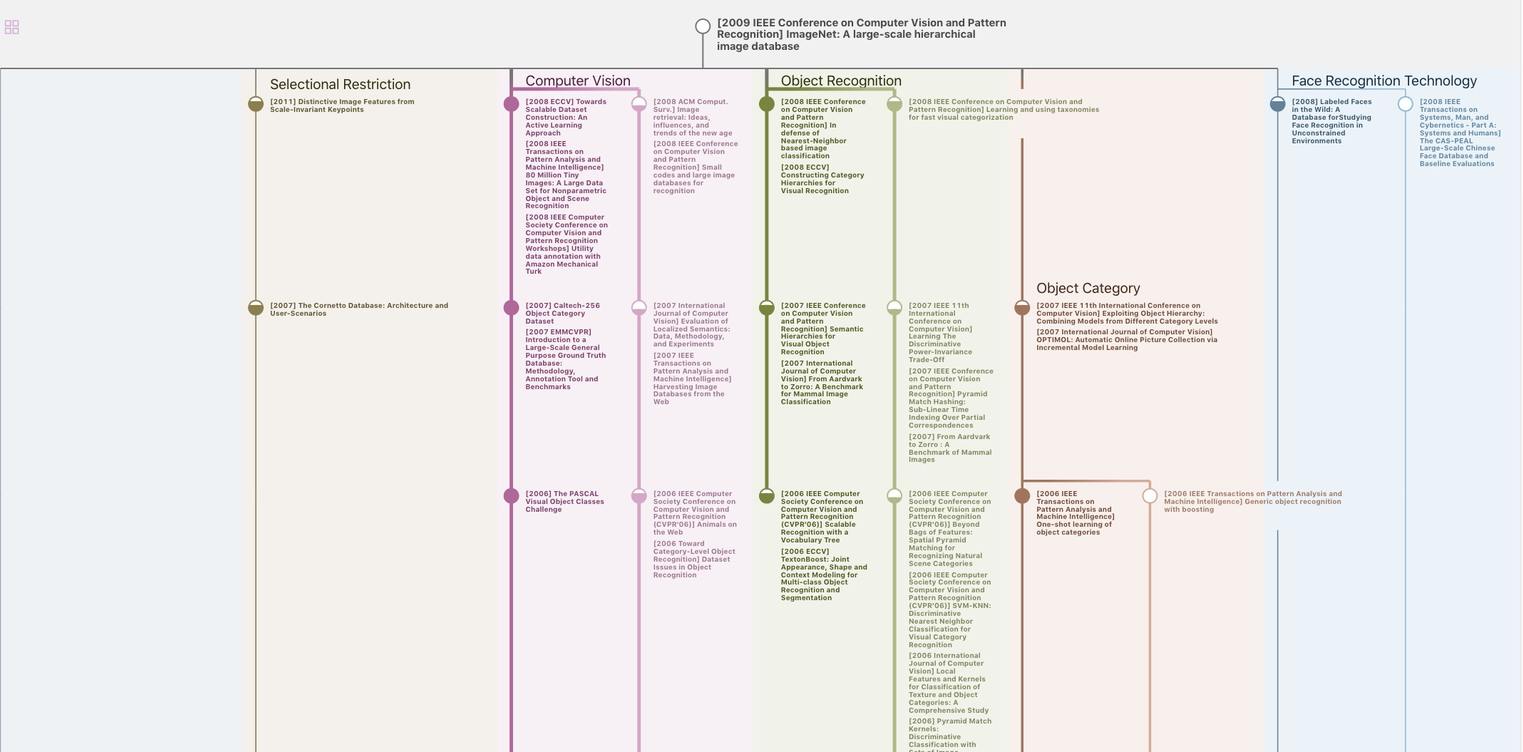
生成溯源树,研究论文发展脉络
Chat Paper
正在生成论文摘要