Less than One-shot: Named Entity Recognition via Extremely Weak Supervision.
CoRR(2023)
摘要
We study the named entity recognition (NER) problem under the extremely weak supervision (XWS) setting, where only one example entity per type is given in a context-free way. While one can see that XWS is lighter than one-shot in terms of the amount of supervision, we propose a novel method X-NER that can outperform the state-of-the-art one-shot NER methods. We first mine entity spans that are similar to the example entities from an unlabelled training corpus. Instead of utilizing entity span representations from language models, we find it more effective to compare the context distributions before and after the span is replaced by the entity example. We then leverage the top-ranked spans as pseudo-labels to train an NER tagger. Extensive experiments and analyses on 4 NER datasets show the superior end-to-end NER performance of X-NER, outperforming the state-of-the-art few-shot methods with 1-shot supervision and ChatGPT annotations significantly. Finally, our X-NER possesses several notable properties, such as inheriting the cross-lingual abilities of the underlying language models.
更多查看译文
关键词
named entity recognition,entity recognition,one-shot
AI 理解论文
溯源树
样例
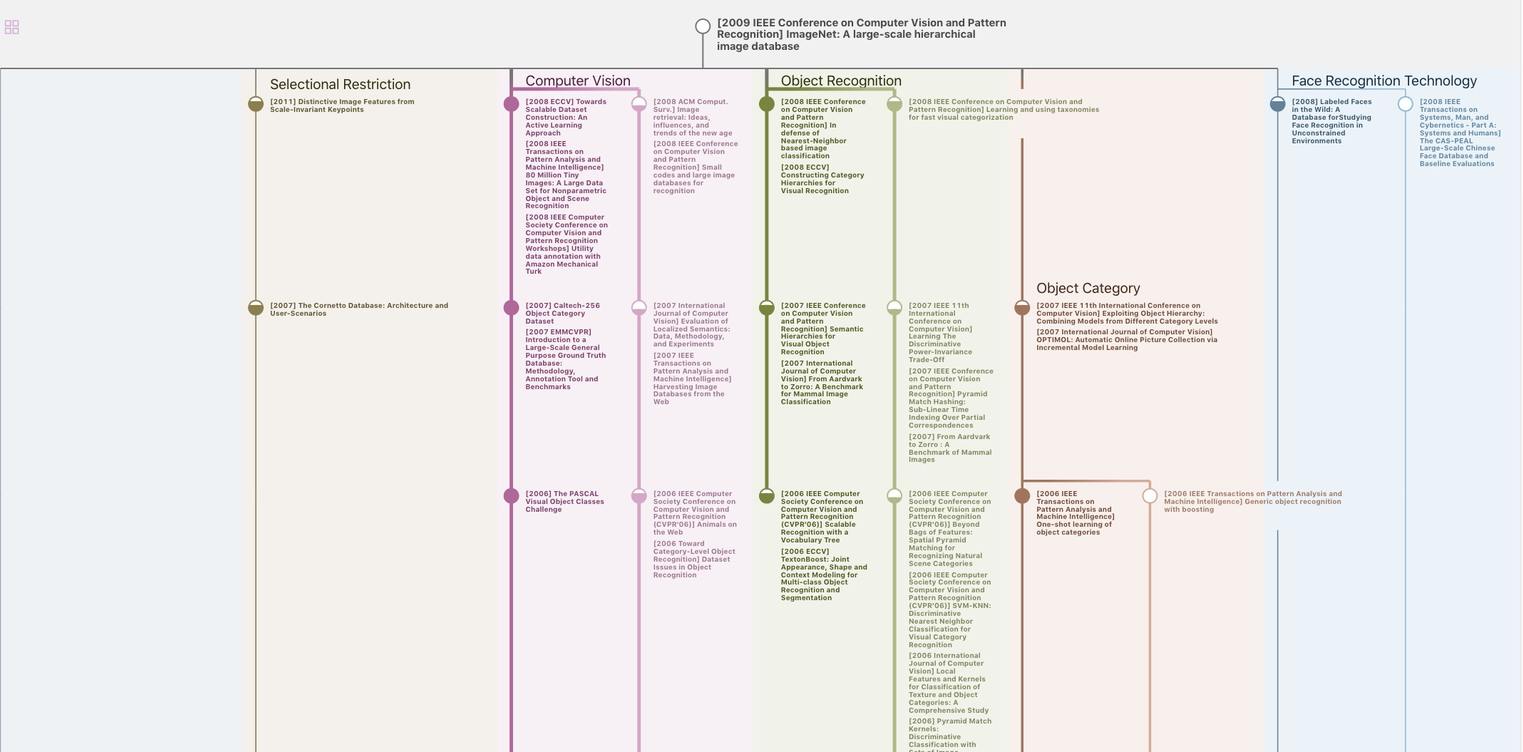
生成溯源树,研究论文发展脉络
Chat Paper
正在生成论文摘要